self.fc = nn.Linear(in_feature, numclass, bias=True) self.fc.weight.data[:out_feature] = weight self.fc.bias.data[:out_feature] = bias
时间: 2024-04-17 19:23:50 浏览: 10
这段代码是在定义一个神经网络模型的类,并初始化了一个线性层(`nn.Linear`)。`in_feature`表示输入特征的数量,`numclass`表示输出的类别数量。`bias=True`表示是否使用偏置项。
接下来的两行代码是对线性层的权重和偏置进行初始化。`weight`是一个形状为`(out_feature, in_feature)`的张量,表示权重矩阵,其中`out_feature`表示输出特征的数量。通过`self.fc.weight.data[:out_feature] = weight`将权重矩阵的前`out_feature`行赋值为`weight`。
`bias`是一个形状为`(out_feature,)`的张量,表示偏置向量。通过`self.fc.bias.data[:out_feature] = bias`将偏置向量的前`out_feature`个元素赋值为`bias`。
这样,线性层的权重和偏置就被初始化为指定的值。
相关问题
self.fc = nn.Linear(in_features=576, out_features=128)
好的,那么这个代码就是添加了一个输入特征维度为576,输出特征维度为128的全连接层。完整的代码如下:
```python
import torch.nn as nn
class MyModel(nn.Module):
def __init__(self):
super(MyModel, self).__init__()
# 假设输入的特征维度为 input_size
input_size = 768
# 假设输出的特征维度为 output_size
output_size = 10
# 添加一个全连接层
self.fc = nn.Linear(in_features=576, out_features=128)
# 初始化全连接层的权重和偏置
nn.init.xavier_normal_(self.fc.weight)
nn.init.constant_(self.fc.bias, 0.0)
def forward(self, x):
# 假设输入 x 的形状为 [batch_size, input_size]
# 经过全连接层后,输出的形状为 [batch_size, 128]
x = self.fc(x)
return x
```
这个全连接层的输入特征维度为576,输出特征维度为128。在 `__init__` 函数中,我们将这个全连接层添加到模型中,并使用 `nn.init` 初始化其权重和偏置。在 `forward` 函数中,我们将输入 `x` 经过全连接层并返回输出。
self.fc = nn.Linear(in_dim, num_heads * out_dim, bias=False)
这行代码定义了一个全连接层(`nn.Linear`)作为模型的一部分。
`nn.Linear`是PyTorch中的线性层,它将输入张量与权重矩阵相乘,并加上一个可选的偏置项。它的函数签名如下:
```python
nn.Linear(in_features, out_features, bias=True)
```
参数说明:
- `in_features`:输入特征的大小,也就是输入张量的维度。
- `out_features`:输出特征的大小,也就是输出张量的维度。
- `bias`:是否使用偏置项,默认为`True`。
在给定的代码中,`in_dim`表示输入特征的大小,`num_heads * out_dim`表示输出特征的大小,`bias=False`表示不使用偏置项。这行代码创建了一个全连接层,输入大小为`in_dim`,输出大小为`num_heads * out_dim`,并且没有偏置项。该全连接层被赋值给`self.fc`属性,以便在模型中进行后续使用。
相关推荐
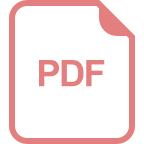
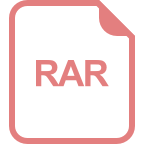
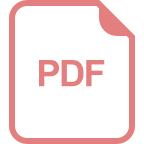













