使用sklearn对数据进行非线性回归预测的代码
时间: 2023-11-23 20:07:55 浏览: 70
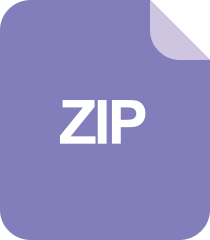
基于支持向量机(SVM)的数据回归预测Python代码 sklearn实现

下面是一个使用sklearn对数据进行非线性回归预测的示例代码:
```python
from sklearn.pipeline import make_pipeline
from sklearn.preprocessing import PolynomialFeatures
from sklearn.linear_model import LinearRegression
import numpy as np
# 生成数据
X = np.linspace(-3, 3, 100)
y = np.sin(X) + np.random.randn(100) * 0.1
# 创建模型
model = make_pipeline(PolynomialFeatures(7), LinearRegression())
# 训练模型
model.fit(X.reshape(-1, 1), y)
# 预测
X_test = np.linspace(-3, 3, 100)
y_pred = model.predict(X_test.reshape(-1, 1))
# 绘图
import matplotlib.pyplot as plt
plt.scatter(X, y)
plt.plot(X_test, y_pred, color='red')
plt.show()
```
在这个示例中,我们首先生成了一些具有非线性关系的数据。然后,我们创建了一个`PolynomialFeatures`对象,它可以将特征转换为多项式特征,从而实现对非线性关系的建模。然后,我们将`PolynomialFeatures`对象和`LinearRegression`对象包装在一个`make_pipeline`对象中,这样我们就可以方便地进行训练和预测。最后,我们使用预测结果绘制了一个图形,以直观地展示模型的预测效果。
阅读全文
相关推荐

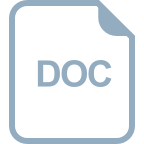
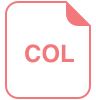



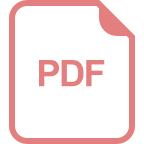








