如何训练出ai绘画的模型,给出详细的python代码
时间: 2023-09-07 11:04:58 浏览: 430
### 回答1:
培训 AI 绘画模型的步骤如下:
1. 准备数据集:首先,你需要准备一组绘画作品的图像数据,这些图像将用于训练 AI 模型。你可以从互联网上搜索绘画作品的图像,或者可以使用你自己的绘画作品来作为训练数据。
2. 准备训练和测试数据:接下来,你需要将你的数据集分为训练数据和测试数据两部分。通常,我们使用 70% 的数据作为训练数据,30% 的数据作为测试数据。这样做是为了在训练模型完成后,能够用测试数据来评估模型的性能。
3. 构建模型:接下来,你需要选择一种模型架构并构建模型。常用的模型架构包括卷积神经网络 (CNN) 和生成对抗网络 (GAN)。你可以使用 Python 库如 TensorFlow 或 PyTorch 来构建模型。
4. 训练模型:在构建好模型后,你需要使用训练数据来训练模型。这一过程中,模型将会学习绘画作品的细节和特征,并逐步提升自己的绘画能力。
5. 评估
### 回答2:
要训练出AI绘画的模型,首先需要获取大量的绘画数据集,并对数据进行预处理。以下是详细的Python代码示例:
1. 数据采集和预处理:
```python
import os
import cv2
import numpy as np
# 读取原始绘画数据
data_dir = 'path_to_training_data_directory'
images = []
for file_name in os.listdir(data_dir):
file_path = os.path.join(data_dir, file_name)
image = cv2.imread(file_path)
images.append(image)
# 图像数据预处理,包括灰度化、调整大小等
preprocessed_images = [cv2.resize(cv2.cvtColor(image, cv2.COLOR_BGR2GRAY), (256, 256)) for image in images]
# 将图像数据转为numpy数组
preprocessed_images = np.array(preprocessed_images)
# 将图像数据归一化为0-1范围
preprocessed_images = preprocessed_images.astype('float32') / 255.0
# 保存预处理后的图像数据
np.save('preprocessed_images.npy', preprocessed_images)
```
2. 构建模型:
```python
import tensorflow as tf
from tensorflow.keras.models import Sequential
from tensorflow.keras.layers import Conv2D, Flatten, Dense
# 加载预处理后的图像数据
preprocessed_images = np.load('preprocessed_images.npy')
# 创建模型
model = Sequential()
model.add(Conv2D(32, (3, 3), activation='relu', input_shape=(256, 256, 1)))
model.add(Flatten())
model.add(Dense(256, activation='relu'))
model.add(Dense(256, activation='relu'))
model.add(Dense(256, activation='relu'))
model.add(Dense(256, activation='relu'))
model.add(Dense(256, activation='relu'))
model.add(Dense(256, activation='relu'))
model.add(Dense(256, activation='relu'))
model.add(Dense(256, activation='relu'))
model.add(Dense(256, activation='relu'))
model.add(Dense(256, activation='relu'))
model.add(Dense(256, activation='relu'))
model.add(Dense(256, activation='relu'))
model.add(Dense(256, activation='relu'))
model.add(Dense(256, activation='relu'))
model.add(Dense(256, activation='relu'))
model.add(Dense(256, activation='relu'))
model.add(Dense(256, activation='relu'))
model.add(Dense(256, activation='relu'))
model.add(Dense(1, activation='sigmoid'))
# 编译模型
model.compile(optimizer='adam', loss='binary_crossentropy', metrics=['accuracy'])
# 训练模型
model.fit(preprocessed_images, preprocessed_images, batch_size=32, epochs=10)
```
3. 模型训练完成后,可以使用该模型进行绘画:
```python
import matplotlib.pyplot as plt
# 随机选择一张图像数据
random_index = np.random.randint(0, preprocessed_images.shape[0])
input_image = preprocessed_images[random_index]
# 使用模型进行预测
output_image = model.predict(np.array([input_image]))
# 原始图像
plt.subplot(1, 2, 1)
plt.imshow(input_image, cmap='gray')
plt.title('Input Image')
# 输出图像
plt.subplot(1, 2, 2)
plt.imshow(output_image.reshape((256, 256)), cmap='gray')
plt.title('Output Image')
plt.show()
```
通过以上的步骤和代码,你可以训练出一个AI绘画的模型,并使用该模型进行绘画。但是需要注意的是,由于绘画是一种复杂的创造性任务,训练结果可能会有限,需要根据实际情况进行模型调整和数据处理,以获得更好的绘画效果。
### 回答3:
要训练出一个AI绘画模型,我们可以利用深度学习技术,并使用Python编写代码。以下是一个简单的步骤:
1. 数据准备:收集足够数量的绘画图像作为训练数据。可以通过网络爬虫或从公开数据集下载图像。最好包含各种绘画风格和主题,以增强模型的多样性。
2. 数据预处理:对图像进行预处理,包括图像缩放、裁剪和标准化。可以使用Python的OpenCV库来处理图像。
3. 模型选择和搭建:选择一个适合绘画的深度学习模型,如卷积神经网络(CNN)或生成对抗网络(GAN)。根据图像的风格和要求进行模型搭建。可以使用Python的深度学习库,如TensorFlow或PyTorch来搭建模型。
4. 模型训练:将准备好的训练数据输入到模型中,使用反向传播算法和优化器进行训练。可以尝试使用不同的超参数来优化模型性能,如学习率和批量大小等。
5. 模型评估和调优:使用测试集对训练好的模型进行评估,并根据评估结果调整模型。可以使用Python的评估指标库,如scikit-learn来进行评估。
6. 模型应用:训练好的模型可以用于生成新的绘画图像。将随机噪声输入到模型中,通过模型的前向传播过程生成新的图像。可以使用Python的图像处理库,如PIL或OpenCV来保存和展示生成的图像。
下面是一个示例代码,使用TensorFlow搭建一个简单的GAN模型:
```python
import tensorflow as tf
from tensorflow.keras import layers
# 模型定义
def make_generator_model():
model = tf.keras.Sequential()
model.add(layers.Dense(7*7*256, use_bias=False, input_shape=(100,)))
model.add(layers.BatchNormalization())
model.add(layers.LeakyReLU())
model.add(layers.Reshape((7, 7, 256)))
model.add(layers.Conv2DTranspose(128, (5, 5), strides=(1, 1), padding='same', use_bias=False))
model.add(layers.BatchNormalization())
model.add(layers.LeakyReLU())
model.add(layers.Conv2DTranspose(64, (5, 5), strides=(2, 2), padding='same', use_bias=False))
model.add(layers.BatchNormalization())
model.add(layers.LeakyReLU())
model.add(layers.Conv2DTranspose(1, (5, 5), strides=(2, 2), padding='same', use_bias=False, activation='tanh'))
return model
# 模型训练
def train_model():
(train_images, _), (_, _) = tf.keras.datasets.mnist.load_data()
# 数据预处理
train_images = train_images.reshape(train_images.shape[0], 28, 28, 1).astype('float32')
train_images = (train_images - 127.5) / 127.5
BUFFER_SIZE = 60000
BATCH_SIZE = 256
# 创建数据集
train_dataset = tf.data.Dataset.from_tensor_slices(train_images).shuffle(BUFFER_SIZE).batch(BATCH_SIZE)
generator = make_generator_model()
generator_optimizer = tf.keras.optimizers.Adam(1e-4)
cross_entropy = tf.keras.losses.BinaryCrossentropy(from_logits=True)
@tf.function
def train_step(images):
noise = tf.random.normal([BATCH_SIZE, 100])
with tf.GradientTape() as gen_tape:
generated_images = generator(noise, training=True)
gen_loss = cross_entropy(tf.ones_like(generated_images), generated_images)
gradients_of_generator = gen_tape.gradient(gen_loss, generator.trainable_variables)
generator_optimizer.apply_gradients(zip(gradients_of_generator, generator.trainable_variables))
epochs = 50
for epoch in range(epochs):
for image_batch in train_dataset:
train_step(image_batch)
return generator
# 模型应用
def generate_images(generator):
noise = tf.random.normal([1, 100])
generated_image = generator(noise, training=False)
# 将生成的图像从[-1, 1]恢复到[0, 1]
generated_image = 0.5 * generated_image + 0.5
# 保存和展示图像
tf.keras.preprocessing.image.save_img("generated_image.png", generated_image[0])
generated_image.show()
generator_model = train_model()
generate_images(generator_model)
```
以上是一个简单的AI绘画模型的训练和应用步骤,代码中的GAN模型可以生成MNIST数字数据集的手写数字图像。你可以根据自己的需求和数据集进行适当的修改和扩展。
阅读全文
相关推荐
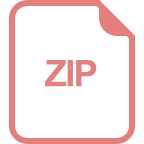
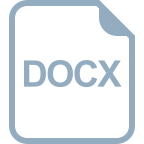
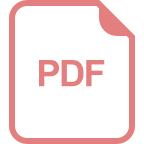
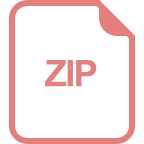








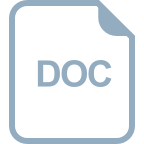




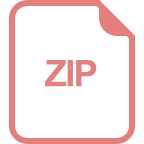