import pickle from sklearn.model_selection import train_test_split ts = 0.3 # Percentage of images that we want to use for testing. X_train, X_test1, y_train, y_test1 = train_test_split(X, y, test_size=ts, random_state=42) X_test, X_cal, y_test, y_cal
时间: 2023-08-10 13:16:31 浏览: 41
这段代码中,我们使用了sklearn库中的train_test_split函数对数据集进行了划分,其中X和y分别代表输入特征和标签向量。我们将数据集划分成了训练集和测试集,其中测试集的大小占总数据集大小的30%,随机种子为42。
如果要进一步将测试集划分成测试集和校验集,可以使用类似的方法,具体代码如下:
```python
import pickle
from sklearn.model_selection import train_test_split
ts = 0.3 # Percentage of images that we want to use for testing.
# load data from pickle file
with open('data.pkl', 'rb') as f:
data = pickle.load(f)
X = data['X']
y = data['y']
# split data into training, testing, and calibration sets
X_train, X_test, y_train, y_test = train_test_split(X, y, test_size=ts, random_state=42)
ts_cal = 0.5 # Percentage of testing images that we want to use for calibration.
X_test, X_cal, y_test, y_cal = train_test_split(X_test, y_test, test_size=ts_cal, random_state=42)
```
在这个示例代码中,我们首先使用pickle库从文件中加载数据集。然后,我们将数据集划分成训练集和测试集。接着,我们将测试集划分成测试集和校验集,其中校验集的大小占测试集大小的50%。最终得到的变量分别是X_train、X_test、X_cal、y_train、y_test、y_cal,分别表示训练集、测试集、校验集的输入特征和标签向量。
相关推荐
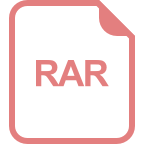















