for t in task_names + ["common", "data_loaders", "models", "schedule"]: sys.modules.pop(t)
时间: 2023-09-21 17:07:14 浏览: 32
这代码的作用是清除Python模块缓存中已经加载的模块。其中,task_names 是一个模块名的列表,"common", "data_loaders", "models", "schedule" 是额外需要清除的模块名。
当Python加载一个模块时,它会将模块的信息存储在缓存中,以便在下一次需要同一模块时可以更快地访问它。但是,有时候需要重新加载模块,以便在代码修改后生效。这时就需要清除缓存中的模块信息,让Python重新加载模块。
在这个代码片段中,使用了 sys.modules.pop() 方法来清除模块缓存。该方法会从 sys.modules 字典中移除指定的模块,并返回该模块对象。由于模块对象被移除,下一次再加载该模块时,Python会重新从磁盘中读取模块文件并重新构建模块对象。
相关问题
for loader, name in conf.eval_loaders: AttributeError: module 'module' has no attribute 'eval_loaders'
This error occurs when the module being imported does not have the attribute "eval_loaders". This could be because the attribute is not defined in the module or there is a typo in the attribute name.
To solve this error, you can try the following steps:
1. Check if the attribute is defined in the module. You can open the module and search for the "eval_loaders" attribute.
2. Check if there is a typo in the attribute name. Make sure that the attribute name is spelled correctly and matches the attribute name in the module.
3. If the module is part of a package, make sure that you are importing the correct module. You may need to check the package structure to ensure that you are importing the correct module.
4. If none of the above solutions work, try restarting your Python interpreter or IDE. Sometimes, modules can get cached and restarting can help refresh the cache.
File was processed with these loaders: * ./node_modules/@pmmmwh/react-refresh-webpack-plugin/loader/index.js You may need an additional loader to handle the result of these loaders. | import "./App.less"; | > const Container: React.FC<{}> = () => { | return ( | <>
这个错误通常是由于缺少对应的 loader 导致的。根据错误提示信息,你使用了 `react-refresh-webpack-plugin` 这个插件,而这个插件使用了一个自定义的 loader。可能的解决方法是在 webpack 配置文件中添加一个额外的 loader,用来处理这个插件的返回值。你可以尝试在 webpack 配置文件中添加如下代码:
```js
module: {
rules: [
{
test: /\.js$/,
exclude: /node_modules/,
use: {
loader: 'babel-loader',
options: {
presets: ['@babel/preset-react'],
},
},
},
{
test: /\.less$/,
use: [
{
loader: 'style-loader',
},
{
loader: 'css-loader',
},
{
loader: 'less-loader',
},
],
},
// 添加这个 loader 处理 react-refresh-webpack-plugin 的返回值
{
test: /react-refresh-webpack-plugin/,
use: [
{
loader: 'babel-loader',
options: {
plugins: ['react-refresh/babel'],
},
},
],
},
],
},
```
这段代码中,我们针对 `react-refresh-webpack-plugin` 添加了一个 babel-loader,用来处理该插件的返回值。注意,这个 loader 的 `test` 属性需要设置成 `react-refresh-webpack-plugin`,这样才能正确匹配到该插件的返回值。
相关推荐
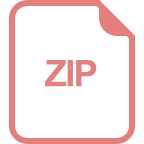
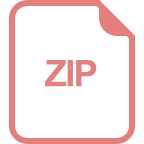












