`training_epoch_end`
时间: 2023-10-22 12:06:40 浏览: 24
`training_epoch_end` 是一个在训练过程中的每个 epoch 结束时被调用的函数。在训练神经网络时,通常会分为多个 epoch,每个 epoch 表示模型遍历整个训练数据集的一次。而 `training_epoch_end` 函数则用于对每个 epoch 进行一些后处理操作。
在 `training_epoch_end` 函数中,可以执行一些与模型训练相关的操作,例如计算并记录训练集和验证集上的指标,绘制训练曲线图等。这个函数可以提供有关每个 epoch 的一些统计信息,以便在训练过程中进行监控和可视化。
需要注意的是,`training_epoch_end` 函数是在每个 epoch 结束时被调用的,而不是在整个训练过程结束时被调用的。在这个函数中,你可以访问当前 epoch 的模型状态、损失值、准确率等信息,并根据需要进行自定义处理。
这只是对 `training_epoch_end` 的一个简要解释,具体的使用方法和实现细节可能会因不同的开发框架或库而有所不同。如果你需要更详细的信息,请提供更具体的背景或上下文。
相关问题
from mindspore.train.callback import ModelCheckpoint, CheckpointConfig, LossMonitor, TimeMonitor class LossCallBack(LossMonitor): """ Monitor the loss in training. If the loss in NAN or INF terminating training. """ def __init__(self, has_trained_epoch=0, per_print_times=per_print_steps): super(LossCallBack, self).__init__() self.has_trained_epoch = has_trained_epoch self._per_print_times = per_print_times def step_end(self, run_context): cb_params = run_context.original_args() loss = cb_params.net_outputs if isinstance(loss, (tuple, list)): if isinstance(loss[0], ms.Tensor) and isinstance(loss[0].asnumpy(), np.ndarray): loss = loss[0] if isinstance(loss, ms.Tensor) and isinstance(loss.asnumpy(), np.ndarray): loss = np.mean(loss.asnumpy()) cur_step_in_epoch = (cb_params.cur_step_num - 1) % cb_params.batch_num + 1 if isinstance(loss, float) and (np.isnan(loss) or np.isinf(loss)): raise ValueError("epoch: {} step: {}. Invalid loss, terminating training.".format( cb_params.cur_epoch_num, cur_step_in_epoch)) if self._per_print_times != 0 and cb_params.cur_step_num % self._per_print_times == 0: # pylint: disable=line-too-long print("epoch: %s step: %s, loss is %s" % (cb_params.cur_epoch_num + int(self.has_trained_epoch), cur_step_in_epoch, loss), flush=True) time_cb = TimeMonitor(data_size=step_size) loss_cb = LossCallBack(has_trained_epoch=0) cb = [time_cb, loss_cb] ckpt_save_dir = cfg['output_dir'] device_target = context.get_context('device_target') if cfg['save_checkpoint']: config_ck = CheckpointConfig(save_checkpoint_steps=save_ckpt_num*step_size, keep_checkpoint_max=10) # config_ck = CheckpointConfig(save_checkpoint_steps=5*step_size, keep_checkpoint_max=10) ckpt_cb = ModelCheckpoint(prefix="resnet", directory=ckpt_save_dir, config=config_ck) cb += [ckpt_cb]
这段代码定义了一些回调函数,用于在训练过程中监控和保存模型。
首先,定义了一个名为LossCallBack的类,继承自LossMonitor回调类。它重写了step_end方法,在每个训练步骤结束时监控损失值。如果损失值为NaN或INF,将抛出ValueError以终止训练。如果_per_print_times参数不为0且当前步骤数是_per_print_times的倍数,将打印当前的训练损失值。
然后,创建了一个TimeMonitor回调实例和一个LossCallBack回调实例。TimeMonitor用于监控训练时间,LossCallBack用于监控训练损失值。
接着,创建了一个回调列表cb,并将time_cb和loss_cb添加到列表中。同时,获取配置文件中的ckpt_save_dir和device_target。
如果配置文件中的save_checkpoint为True,则创建一个CheckpointConfig实例config_ck,用于配置模型保存的参数(保存间隔、最大保存个数等)。然后,创建一个ModelCheckpoint回调实例ckpt_cb,并将其添加到回调列表cb中。
最后,返回回调列表cb,用于在训练过程中使用。
pytorch_lightning metric
### 回答1:
PyTorch Lightning Metric 是 PyTorch Lightning 中用于评估模型性能的一种工具。Metric 可以用于监控训练过程中的指标,并在每个 epoch 结束时输出结果。PyTorch Lightning Metric 提供了多种内置的评估指标,如 accuracy、precision、recall、F1 等,并且可以自定义评估指标。
使用 PyTorch Lightning Metric 的基本步骤如下:
1. 定义 Metric 类,继承自 `pl.metrics.Metric`
2. 在类中实现 `update` 方法,用于更新评估指标
3. 在类中实现 `compute` 方法,用于计算最终的评估结果
4. 在 LightningModule 中使用 `self.log()` 方法输出评估结果
例如,下面是一个计算 accuracy 的 Metric 类的示例代码:
```python
import torch
import pytorch_lightning as pl
class Accuracy(pl.metrics.Metric):
def __init__(self, dist_sync_on_step=False):
super().__init__(dist_sync_on_step=dist_sync_on_step)
self.add_state("correct", default=torch.tensor(0), dist_reduce_fx="sum")
self.add_state("total", default=torch.tensor(0), dist_reduce_fx="sum")
def update(self, preds, target):
preds = torch.argmax(preds, dim=1)
self.correct += torch.sum(preds == target)
self.total += target.numel()
def compute(self):
return self.correct.float() / self.total
```
在 LightningModule 中使用该 Metric 可以如下使用:
```python
class MyModel(pl.LightningModule):
def __init__(self):
super().__init__()
self.accuracy = Accuracy()
def training_step(self, batch, batch_idx):
...
self.accuracy(preds, target)
...
def training_epoch_end(self, outputs):
...
self.log('train_acc', self.accuracy.compute(), on_step=False, on_epoch=True)
...
```
在每个 epoch 结束时,`self.accuracy.compute()` 方法将计算 accuracy 并返回最终的评估结果。`self.log()` 方法用于输出评估结果,其中 `on_epoch=True` 表示只在每个 epoch 结束时输出,而不是每个 batch 结束时都输出。
### 回答2:
PyTorch Lightning是一个轻量级而强大的深度学习框架,提供了许多指标(metric)来帮助我们评估模型的性能。这些指标可以帮助我们了解训练过程中模型的表现,从而对模型进行改进和优化。
PyTorch Lightning中的指标(metric)可以分为两类:训练指标和验证指标。训练指标是针对训练阶段的评估,而验证指标则是在验证阶段对模型进行评估。
常见的训练指标包括准确率(Accuracy)和损失(Loss)。准确率可以衡量模型在训练集上的分类预测准确性,而损失则可以衡量模型的学习效果。PyTorch Lightning提供了内置的函数来计算这些指标,使得评估过程更加方便。
此外,PyTorch Lightning还提供了丰富的验证指标。常见的验证指标包括精确度(Precision)、召回率(Recall)和F1-score。这些指标可以帮助我们更全面地了解模型在验证集上的性能表现。PyTorch Lightning也提供了内置的函数来计算这些指标。
对于更复杂的模型评估需求,PyTorch Lightning还可以自定义指标。我们可以通过继承`torchmetrics.Metric`类来定义自己的指标函数,并在训练或验证过程中使用这些指标。
总之,PyTorch Lightning提供了丰富的指标来帮助我们评估模型的性能。无论是训练指标还是验证指标,这些指标都能够帮助我们更好地了解模型的表现,并且能够进行自定义来满足特定的评估需求。
### 回答3:
PyTorch Lightning是一个针对PyTorch的轻量级深度学习框架,它提供了一种易于使用的方式来组织和管理训练代码。在PyTorch Lightning中,Metric(度量指标)是一个用于评估模型性能的重要组成部分。
PyTorch Lightning Metric的主要作用是衡量模型在训练和验证过程中的性能。它提供了一种标准化的方式来计算和跟踪诸如准确率、损失、F1分数等指标。使用Metric能够帮助我们更好地理解和衡量模型的表现。
PyTorch Lightning预定义了一些常见的Metric,如Accuracy、Precision、Recall、F1、Mean Squared Error等。使用这些预定义的Metric,我们只需简单地实例化并传递给Lightning Module,再通过训练循环使用update方法来更新Metric的值。例如,我们可以在每个训练批次和验证结束后计算Accuracy,并跟踪模型在训练过程中的性能。
此外,PyTorch Lightning还支持自定义Metric,我们可以根据实际需求定义自己的Metric函数。实现自定义Metric函数时,我们需要定义`__init__`方法、`update`方法和`compute`方法。`__init__`方法用于初始化Metric的变量,`update`方法用于根据模型预测结果和真实标签更新Metric的值,`compute`方法用于计算Metric最终的结果。
总结来说,PyTorch Lightning Metric是一种用于评估模型性能的工具,它提供了一种标准化的方式来计算和跟踪模型的性能指标。它可以帮助我们更好地理解和衡量模型的表现,并且可以方便地使用预定义的指标或自定义的指标来评估模型。
相关推荐
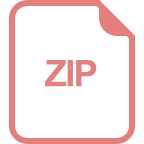












