function [GD]=convergence(obj,ref_point)%传入已经归一化的目标值和参考点 [obj_size,~]=size(obj); [ref_size,~]=size(ref_point); distance=zeros(obj_size,ref_size); GD=0; for i=1:obj_size for j=1:ref_size distance(i,j)=(obj(i,1)-ref_point(j,1))^2+(obj(i,2)-ref_point(j,2))^2; distance(i,j)=sqrt(distance(i,j)); end GD=GD+min(distance(i,:)); end GD=GD/obj_size; end
时间: 2024-04-04 10:33:17 浏览: 99
这段代码实现了一种多目标优化算法中的收敛度量指标——广义距离(General Distance,GD)的计算。具体来说,它计算了一组已经归一化的目标值(obj)与一个参考点(ref_point)之间的距离(这里使用欧氏距离),并取每个目标值到最近的参考点的距离的平均值作为GD值。
代码中首先获取了obj和ref_point的大小(行数和列数),然后定义了一个大小为obj_size x ref_size的矩阵distance,并将其所有元素初始化为0。接下来使用两个for循环分别对obj和ref_point中的每个目标值和参考点进行遍历,计算它们之间的欧氏距离,并将计算结果存储到distance矩阵中。最后,对于每个目标值,找到它到所有参考点中距离最近的那个参考点,并将这个最小距离加入GD中。最后,将GD除以目标值的个数得到GD的值,并将其作为函数的返回值。
相关问题
function [GD]=convergence(obj,ref_point)%
这是一个函数,用于计算多目标优化算法的收敛性。其输入参数是obj和ref_point,其中obj是一个n行m列的矩阵,表示n个解的目标函数值,m为目标函数的个数;ref_point是一个1行m列的矩阵,表示参考点的目标函数值。
函数输出为GD,表示当前解集到参考点的距离的平均值。
具体实现如下:
```matlab
function [GD]=convergence(obj,ref_point)
% obj: n*m matrix, n is the number of solutions, and m is the number of objectives
% ref_point: 1*m matrix, the reference point for calculating GD
[n,m]=size(obj);
d=zeros(n,1);
for i=1:n
for j=1:m
d(i)=d(i)+(obj(i,j)-ref_point(j))^2;
end
d(i)=sqrt(d(i));
end
GD=mean(d);
end
```
该函数首先计算每个解到参考点的距离,然后求平均值作为GD的值返回。通过比较GD的大小,可以评估多目标优化算法的收敛性。如果GD值越小,则说明算法收敛得越好。
model.fit(initial_learning_rate=0.01, learning_rate_decay=1.5, convergence_epochs=5, batch_size=32, maximum_epochs=100, learning_rate_minimum=1e-4, training_split=0.8)
这是一个模型训练的函数调用,它使用了一些参数来控制训练过程。让我来解释一下这些参数的作用:
- `initial_learning_rate`:初始学习率,用于控制模型参数的更新速度。较大的学习率通常会使模型在初始训练阶段收敛更快,但也可能导致模型在后期训练时发散。
- `learning_rate_decay`:学习率衰减率,用于控制学习率的衰减速度。在每个迭代周期之后,学习率会乘以衰减率以降低其值。这有助于让模型在训练的后期阶段更加稳定。
- `convergence_epochs`:收敛周期数,指定当模型在连续多少个周期内没有显著改善时,训练过程将被提前终止。这有助于防止模型过拟合或者在训练过程中浪费时间。
- `batch_size`:批次大小,指定每次迭代中用于更新模型参数的样本数量。较大的批次大小可以提高训练速度,但也可能导致模型陷入局部最优解。
- `maximum_epochs`:最大训练周期数,用于指定模型的最大训练时长。当达到最大周期数时,训练过程将被终止,无论模型是否已经收敛。
- `learning_rate_minimum`:学习率下限,用于指定学习率的最小值。如果学习率衰减后的值小于该下限,将使用下限值作为实际学习率。
- `training_split`:训练集拆分比例,用于指定将数据集拆分为训练集和验证集的比例。训练集用于模型参数的更新,而验证集用于评估模型的性能。
这些参数的具体取值应根据具体问题和数据集进行调整。
阅读全文
相关推荐
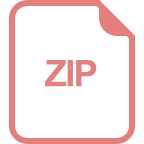
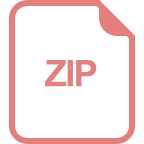
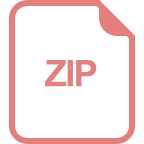
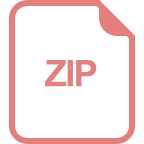
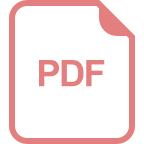
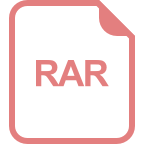










