learning_rate = 0.01 momentum = 0.5 log_interval = 10 random_seed = 1
时间: 2024-06-06 11:07:49 浏览: 118
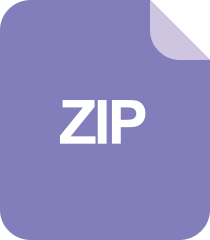
neural network.zip_Random Number _S函数BP网络_adding momentum_区间神经网络
These are parameters used in machine learning or deep learning algorithms. Here's what they mean:
- `learning_rate`: This is a hyperparameter that determines the step size at each iteration while moving toward a minimum of a loss function. A high learning rate can cause the algorithm to converge quickly, but it may overshoot the minimum. A low learning rate can cause the algorithm to converge slowly, but it may have a better chance of finding the true minimum.
- `momentum`: This is another hyperparameter that controls the rate at which the algorithm learns from previous iterations. A high momentum value means that the algorithm will take larger steps in the direction of the previous gradient, while a low momentum value means that the algorithm will take smaller steps.
- `log_interval`: This parameter determines how often the algorithm will print out progress updates. For example, if `log_interval` is set to 10, the algorithm will print out the current loss value every 10 iterations.
- `random_seed`: This parameter is used to set a random seed for the algorithm. Setting a random seed ensures that the algorithm will produce the same results every time it is run, which is useful for reproducibility and debugging.
Overall, these parameters can have a significant impact on the performance and convergence of machine learning algorithms. It's important to experiment with different values and find the optimal combination for a given task.
阅读全文
相关推荐
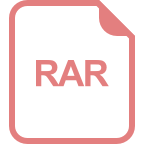
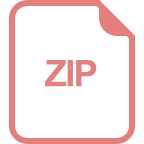
















