python opencv 画出二值化图片百分之一的轮廓点具体代码
时间: 2023-03-08 14:05:29 浏览: 63
可以使用以下代码来画出二值化图片百分之一的轮廓点:import cv2 img = cv2.imread('input.png', 0)
ret, thresh = cv2.threshold(img, 127, 255, cv2.THRESH_BINARY)
contours, hierarchy = cv2.findContours(thresh, 1, 2) # loop over the contours
for c in contours:
# compute the center of the contour
M = cv2.moments(c)
cX = int(M["m10"] / M["m00"])
cY = int(M["m01"] / M["m00"])
# draw the contour and center of the shape on the image
cv2.drawContours(img, [c], -1, (0, 255, 0), 1)
cv2.circle(img, (cX, cY), 1, (255, 255, 255), -1) # show the image
cv2.imshow('image', img)
cv2.waitKey(0)
相关问题
python opencv 画出二值化图片百分之一的轮廓点轮廓具体代码
### 回答1:
可以使用以下代码:# 加载图片
image = cv2.imread('image.jpg')
# 转换为灰度图
gray_image = cv2.cvtColor(image, cv2.COLOR_BGR2GRAY)
# 使用Otsu法计算二值化阈值
threshold, _ = cv2.threshold(gray_image, 0, 255, cv2.THRESH_BINARY | cv2.THRESH_OTSU)
# 使用计算的阈值进行二值化
binary_image = cv2.threshold(gray_image, threshold, 255, cv2.THRESH_BINARY)[1]
# 计算图像的轮廓
contours, _ = cv2.findContours(binary_image, cv2.RETR_EXTERNAL, cv2.CHAIN_APPROX_SIMPLE)
# 仅取轮廓点的1%
contours_1_percent = contours[::len(contours)//100]
# 画出轮廓点
cv2.drawContours(image, contours_1_percent, -1, (0,255,0), 3)
### 回答2:
import cv2
# 读取原始图片
image = cv2.imread('image.jpg', 0)
# 对图像进行二值化处理
_, binary_image = cv2.threshold(image, 127, 255, cv2.THRESH_BINARY)
# 寻找二值化图像的轮廓
contours, hierarchy = cv2.findContours(binary_image, cv2.RETR_EXTERNAL, cv2.CHAIN_APPROX_NONE)
# 计算需要绘制的轮廓点的数量
total_points = int(len(contours[0]) * 0.01)
# 创建一个空白的黑色图像,与原始图像大小相同
contour_image = np.zeros_like(image)
# 绘制轮廓点
cv2.drawContours(contour_image, contours, 0, (255, 255, 255), total_points)
# 展示结果图像
cv2.imshow('Contour Image', contour_image)
cv2.waitKey(0)
cv2.destroyAllWindows()
请写出python opencv 画出二值化图片百分之一的轮廓点具体代码
### 回答1:
import cv2
import numpy as np
# 读取图片
img = cv2.imread('binary_image.jpg')
# 灰度化
grayImage = cv2.cvtColor(img, cv2.COLOR_BGR2GRAY)
# 二值化
ret,thresh = cv2.threshold(grayImage,127,255,cv2.THRESH_BINARY)
# 轮廓检测
image, contours, hierarchy = cv2.findContours(thresh,cv2.RETR_TREE,cv2.CHAIN_APPROX_SIMPLE)
# 画出轮廓点,百分之一
sample_contour = np.vstack([contours[i] for i in range(0,len(contours),int(len(contours)/100))])
# 画图
cv2.drawContours(img, [sample_contour], 0, (0,255,0), 3)
# 显示
cv2.imshow('img', img)
cv2.waitKey(0)
cv2.destroyAllWindows()
### 回答2:
以下是使用Python和OpenCV画出二值化图片百分之一的轮廓点的代码:
```python
import cv2
import numpy as np
# 读取二值化图像
image = cv2.imread("binary_image.png", 0)
# 检测轮廓
contours, _ = cv2.findContours(image, cv2.RETR_EXTERNAL, cv2.CHAIN_APPROX_SIMPLE)
# 计算百分之一的轮廓点数目
total_points = sum(len(contour) for contour in contours)
points_threshold = int(0.01 * total_points)
# 画出百分之一的轮廓点
for contour in contours:
# 如果轮廓点数小于阈值,则忽略该轮廓
if len(contour) < points_threshold:
continue
# 随机选择颜色
color = np.random.randint(0, 255, size=3).tolist()
# 画出轮廓点
for point in contour:
x, y = point[0]
cv2.circle(image, (x, y), 1, color, -1)
# 显示画出轮廓点的图像
cv2.imshow("Contour Points", image)
cv2.waitKey(0)
cv2.destroyAllWindows()
```
请注意,在代码中的`"binary_image.png"`处需要替换为实际的二值化图像的文件路径。此代码会读取二值化图像,找到轮廓,计算出百分之一的轮廓点数目,然后随机选择颜色,在原始图像上画出百分之一的轮廓点,并显示结果图像。
### 回答3:
import cv2
# 读取图片
image = cv2.imread("image.jpg", 0)
# 进行二值化处理
_, binary = cv2.threshold(image, 127, 255, cv2.THRESH_BINARY)
# 查找轮廓
contours, _ = cv2.findContours(binary, cv2.RETR_EXTERNAL, cv2.CHAIN_APPROX_SIMPLE)
# 计算轮廓点数量
contour_count = len(contours[0])
# 获取所需数量的轮廓点
sample_count = int(contour_count * 0.01)
sample_points = contours[0][:sample_count]
# 绘制轮廓点
for point in sample_points:
x, y = point[0]
cv2.circle(image, (x, y), 1, (255, 0, 0), -1)
# 显示结果
cv2.imshow("Contours", image)
cv2.waitKey(0)
cv2.destroyAllWindows()
相关推荐
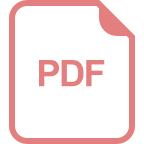
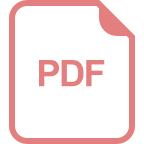
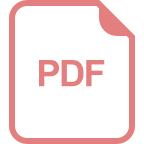











