torch.concat用法,各参数含义
时间: 2024-03-01 15:53:16 浏览: 297
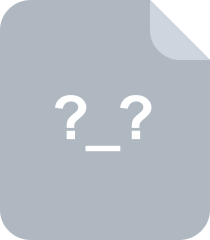
0459-极智开发-解读torch.argmax()函数

torch.concat是PyTorch中的一个函数,用于将多个张量拼接在一起。其基本语法如下:
```python
torch.cat(tensors, dim=0, out=None) -> Tensor
```
其中,参数含义如下:
- `tensors`:需要拼接在一起的张量序列,可以是一个列表或元组。
- `dim`:在哪个维度上进行拼接,默认为0,表示在第一个维度上进行拼接。
- `out`:输出张量,如果不为None,则将结果拷贝到输出张量中。
例如,假设有两个张量a和b,它们的shape分别为(2, 3)和 (2, 4),我们可以按照如下方式将它们在第二个维度上拼接起来:
```python
import torch
a = torch.randn(2, 3)
b = torch.randn(2, 4)
c = torch.cat([a, b], dim=1)
print(c.shape) # 输出(2, 7)
```
在上述示例中,我们首先使用`torch.randn`函数生成了两个大小不同的张量a和b,然后使用`torch.cat`函数将它们在第二个维度上进行拼接,并将结果保存到c中。最后,我们打印c的shape,可以看到它的shape是(2, 7),符合我们的预期。
阅读全文
相关推荐
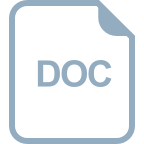
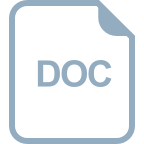

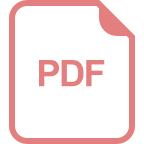





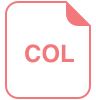
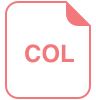
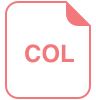
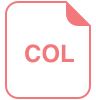
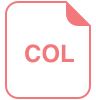
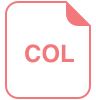

