ValueError: Setting a random_state has no effect since shuffle is False. You should leave random_state to its default (None), or set shuffle=True.
时间: 2024-03-07 10:49:47 浏览: 220
这个错误是因为你在使用 `train_test_split` 函数时设置了 `random_state` 参数,但同时将 `shuffle` 参数设置为了 `False`。这意味着你想按照数据集中的顺序进行拆分,而不是随机拆分。因此,设置 `random_state` 参数没有意义。
要解决这个问题,你可以:
1. 将 `shuffle` 参数设置为 `True`,以便在拆分数据集时随机打乱顺序;
2. 将 `random_state` 参数设置为 `None`,这是默认值,表示使用随机种子来拆分数据集。
以下是两种解决方法的代码示例:
1. 将 `shuffle` 参数设置为 `True`
```python
from sklearn.model_selection import train_test_split
X_train, X_test, y_train, y_test = train_test_split(X, y, test_size=0.2, shuffle=True, random_state=42)
```
2. 将 `random_state` 参数设置为 `None`
```python
from sklearn.model_selection import train_test_split
X_train, X_test, y_train, y_test = train_test_split(X, y, test_size=0.2, shuffle=False, random_state=None)
```
相关问题
valueerror: setting a random_state has no effect since shuffle is false. you should leave random_state to its default (none), or set shuffle=true.
### 回答1:
这是一个错误提示,意思是当shuffle参数设置为false时,设置random_state参数没有任何作用。建议将random_state参数保持默认值(none),或者将shuffle参数设置为true。
### 回答2:
这个错误提示出现在使用机器学习算法中,通常是由于设置了 random_state 参数,但同时将 shuffle 参数设置为 false。此时 random_state 的设置将无效,因为没有进行打乱数据集的操作。因此,如果你需要使用 random_state,应该将 shuffle 参数设置为 true,以确保数据集被打乱。
random_state 是为了保证每次运行时得到的结果一致,它是生成随机数的种子。在机器学习中,经常需要将数据集打乱,以避免模型过度依赖数据集的顺序。如果不对数据集进行打乱操作,可能会导致模型出现过度拟合的情况,从而影响模型的泛化性能。
因此,我们通常会使用 shuffle 参数来控制是否打乱数据集,同时设置 random_state 参数来确保每次打乱的结果一致。但如果在设置 random_state 的同时将 shuffle 设置为 false,那么 random_state 的设置就会失效,因为数据集没有被打乱。为了避免这种情况,应该将 shuffle 参数设置为 true,并且根据需要设置 random_state 参数的数值。
总之,在使用机器学习算法时,应该注意设置 random_state 和 shuffle 参数,以确保模型训练的正确性和结果的可重复性。当出现类似 valueerror: setting a random_state has no effect since shuffle is false. you should leave random_state to its default (none), or set shuffle=true. 这样的错误提示时,需要仔细检查代码中的参数设置是否存在误区,并进行相应的调整。
### 回答3:
这个错误提示是由于在使用某些机器学习算法时,当shuffle参数设置为False时,设置random_state参数并不能产生随机效果。具体来说,当shuffle参数设置为False时,数据不会被打乱,因此无论random_state参数取什么值,数据顺序都不会改变,即不会产生随机效果。
因此,如果想要产生随机效果,应将shuffle参数设置为True。同时,如果不想产生随机效果,应将random_state参数保持默认值(即None),或者不设置该参数。
需要注意的是,某些机器学习算法具有随机性,并且在每次运行时都会随机初始化,因此即使在每次运行中设置了相同的random_state参数,也无法保证得到相同的结果。因此,在使用这些算法时,除了设置random_state参数外,还需要注意其他可能导致结果不稳定的因素,并进行相应的处理。
kf = KFold(n_splits=k, random_state=rs)时候报错下ValueError: Setting a random_state has no effect since shuffle is False. You should leave random_state to its default (None), or set shuffle=True.
这个错误是因为你在使用 `KFold` 函数时设置了 `random_state` 参数,但同时将 `shuffle` 参数设置为了 `False`。这意味着你想按照数据集中的顺序进行拆分,而不是随机拆分。因此,设置 `random_state` 参数没有意义。
要解决这个问题,你可以:
1. 将 `shuffle` 参数设置为 `True`,以便在拆分数据集时随机打乱顺序;
2. 将 `random_state` 参数设置为 `None`,这是默认值,表示使用随机种子来拆分数据集。
以下是两种解决方法的代码示例:
1. 将 `shuffle` 参数设置为 `True`
```python
from sklearn.model_selection import KFold
kf = KFold(n_splits=k, shuffle=True, random_state=rs)
```
2. 将 `random_state` 参数设置为 `None`
```python
from sklearn.model_selection import KFold
kf = KFold(n_splits=k, shuffle=False, random_state=None)
```
阅读全文
相关推荐
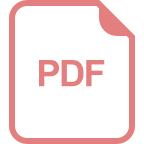
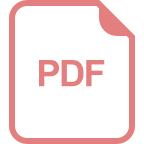
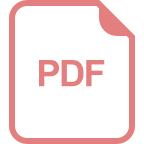












