以下代码是对NSL-KDD数据集网络入侵检测:model = Sequential() model.add(LSTM(128, return_sequences=True, input_shape=(1, X_train.shape[2]))) model.add(Dropout(0.2)) model.add(LSTM(64, return_sequences=True)) model.add(Attention()) model.add(Flatten()) model.add(Dense(units=50)) model.add(Dense(units=5, activation='softmax')) # Defining loss function, optimizer, metrics and then compiling model model.compile(loss='categorical_crossentropy', optimizer='adam', metrics=['accuracy']) # Summary of model layers model.summary() # training the model on training dataset history = model.fit(X_train, y_train, epochs=150, batch_size=5000,validation_split=0.2) # predicting target attribute on testing dataset test_results = model.evaluate(X_test, y_test, verbose=1) # Use the trained model to make predictions on the test dataset y_pred = model.predict(X_test) # Convert predictions from one-hot encoding to integers y_pred = np.argmax(y_pred, axis=1) # Convert true labels from one-hot encoding to integers y_test = np.argmax(y_test, axis=1) # Calculate the confusion matrix cm = confusion_matrix(y_test, y_pred) # Calculate the false positive rate (FPR) fpr = cm[0, 1] / (cm[0, 0] + cm[0, 1])如何用代码实现对模型复杂度的计算
时间: 2023-06-25 15:06:43 浏览: 45
可以使用模型的参数数量作为模型复杂度的度量,可以通过以下代码实现:
```python
# Counting the number of model parameters
num_params = sum([np.prod(var.shape) for var in model.trainable_variables])
print("Number of model parameters: {}".format(num_params))
```
该代码会输出模型中可训练的参数数量,即模型的复杂度。
相关问题
以下代码是对NSL-KDD数据集网络入侵检测:model = Sequential() model.add(LSTM(128, return_sequences=True, input_shape=(1, X_train.shape[2]))) model.add(Dropout(0.2)) model.add(LSTM(64, return_sequences=True)) model.add(Attention()) model.add(Flatten()) model.add(Dense(units=50)) model.add(Dense(units=5, activation='softmax')) # Defining loss function, optimizer, metrics and then compiling model model.compile(loss='categorical_crossentropy', optimizer='adam', metrics=['accuracy']) # Summary of model layers model.summary() # training the model on training dataset history = model.fit(X_train, y_train, epochs=150, batch_size=5000,validation_split=0.2) # predicting target attribute on testing dataset test_results = model.evaluate(X_test, y_test, verbose=1) # Use the trained model to make predictions on the test dataset y_pred = model.predict(X_test) # Convert predictions from one-hot encoding to integers y_pred = np.argmax(y_pred, axis=1) # Convert true labels from one-hot encoding to integers y_test = np.argmax(y_test, axis=1) # Calculate the confusion matrix cm = confusion_matrix(y_test, y_pred) # Calculate the false positive rate (FPR) fpr = cm[0, 1] / (cm[0, 0] + cm[0, 1])如何用代码实现对模型时间复杂度的计算
要计算模型的时间复杂度,需要先确定模型的计算量。可以使用Keras提供的model.count_params()方法来计算模型的总参数数量,然后根据LSTM和Attention层的计算复杂度(详见论文 "An Empirical Exploration of Recurrent Network Architectures")来估算模型的计算量。
代码示例:
```python
import keras.backend as K
def get_lstm_output_shape(layer, input_shape):
# 计算LSTM层输出的形状
if isinstance(layer.output_shape, list):
return tuple(layer.output_shape[0][1:])
else:
return tuple(layer.output_shape[1:])
def get_lstm_params(layer, input_shape):
# 计算LSTM层的参数数量
if layer.use_bias:
bias_multiplier = 3
else:
bias_multiplier = 2
input_dim = input_shape[-1]
units = layer.units
return (input_dim*units + units**2)*bias_multiplier + units*4
def get_attention_params(layer, input_shape):
# 计算Attention层的参数数量
return 2*input_shape[-1]
def get_model_complexity(model, input_shape):
# 计算模型的计算量
total_params = model.count_params()
total_flops = 0
for layer in model.layers:
if isinstance(layer, LSTM):
output_shape = get_lstm_output_shape(layer, input_shape)
params = get_lstm_params(layer, input_shape)
flops = output_shape[0] * (params + output_shape[1] * 2)
total_flops += flops
input_shape = output_shape
elif isinstance(layer, Attention):
params = get_attention_params(layer, input_shape)
flops = input_shape[0] * input_shape[1] * params
total_flops += flops
else:
input_shape = layer.output_shape[1:]
return total_params, total_flops
# 计算模型的计算量
total_params, total_flops = get_model_complexity(model, (1, X_train.shape[2]))
print("Total parameters:", total_params)
print("Total FLOPS:", total_flops)
```
其中,get_lstm_output_shape()函数用于计算LSTM层的输出形状,get_lstm_params()函数用于计算LSTM层的参数数量,get_attention_params()函数用于计算Attention层的参数数量,get_model_complexity()函数则用于计算整个模型的参数数量和计算量。最后,这段代码会输出模型的总参数数量和总计算量。
基于NSL-KDD数据集实现入侵检测python代码
好的,以下是基于NSL-KDD数据集实现入侵检测的Python代码:
```python
# 导入需要的库和模块
import pandas as pd
import numpy as np
from sklearn.model_selection import train_test_split
from sklearn.preprocessing import StandardScaler
from sklearn.metrics import classification_report
from keras.models import Sequential
from keras.layers import Dense, Dropout
# 读取NSL-KDD数据集
data = pd.read_csv('KDDTrain+.txt', header=None)
# 将标签列转换成0和1
data[41] = data[41].apply(lambda x: 0 if x=='normal' else 1)
# 将数据集分为特征和标签
X = data.iloc[:, :-1]
y = data.iloc[:, -1]
# 将特征进行标准化
sc = StandardScaler()
X = sc.fit_transform(X)
# 将数据集分为训练集和测试集
X_train, X_test, y_train, y_test = train_test_split(X, y, test_size=0.2, random_state=42)
# 构建神经网络模型
model = Sequential()
model.add(Dense(units=64, activation='relu', input_dim=X_train.shape[1]))
model.add(Dropout(0.5))
model.add(Dense(units=32, activation='relu'))
model.add(Dropout(0.5))
model.add(Dense(units=1, activation='sigmoid'))
# 编译模型
model.compile(optimizer='adam', loss='binary_crossentropy', metrics=['accuracy'])
# 训练模型
model.fit(X_train, y_train, epochs=10, batch_size=32, validation_data=(X_test, y_test))
# 预测测试集
y_pred = model.predict(X_test)
y_pred = (y_pred > 0.5).astype(int)
# 打印分类报告
print(classification_report(y_test, y_pred))
```
注意,此代码需要Keras和Scikit-learn库。并且,需要将数据集文件KDDTrain+.txt与代码文件放在同一目录下。
相关推荐
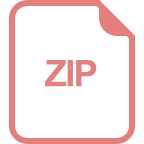
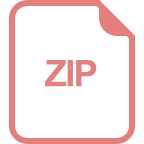












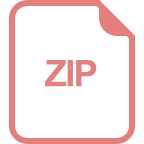
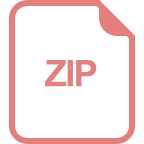