基于神经网路的THUCNews数据集分类python代码实践
时间: 2023-11-09 11:04:42 浏览: 85
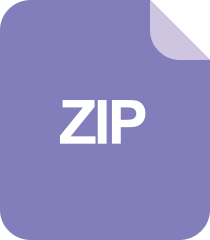
NLP实战之基于tensorflow+字符级CNN进行THUCNews文本分类python
首先需要下载并解压THUCNews数据集,然后安装必要的Python库,如numpy、pandas、jieba、scikit-learn和tensorflow等。
1. 数据预处理
```python
import os
import pandas as pd
import jieba
def read_file(file_path):
with open(file_path, 'r', encoding='utf-8') as f:
text = f.read()
return text
def read_files(file_dir):
news_list = []
for category in os.listdir(file_dir):
category_path = os.path.join(file_dir, category)
for news_file in os.listdir(category_path):
news_file_path = os.path.join(category_path, news_file)
news_content = read_file(news_file_path)
news_list.append({'category': category, 'content': news_content})
news_df = pd.DataFrame(news_list)
return news_df
def preprocess(news_df):
stop_words = read_file('stopwords.txt').split('\n')
news_df['content'] = news_df['content'].apply(lambda x: ' '.join([word for word in jieba.cut(x) if word not in stop_words]))
return news_df
file_dir = 'THUCNews'
news_df = read_files(file_dir)
news_df = preprocess(news_df)
```
2. 特征工程
```python
from sklearn.feature_extraction.text import TfidfVectorizer
from sklearn.model_selection import train_test_split
def tfidf_feature(news_df):
vectorizer = TfidfVectorizer(max_features=5000)
X = vectorizer.fit_transform(news_df['content'])
y = news_df['category']
X_train, X_test, y_train, y_test = train_test_split(X, y, test_size=0.2, random_state=42)
return X_train, X_test, y_train, y_test
X_train, X_test, y_train, y_test = tfidf_feature(news_df)
```
3. 模型训练
```python
import tensorflow as tf
model = tf.keras.Sequential([
tf.keras.layers.Dense(64, activation='relu', input_shape=(5000,)),
tf.keras.layers.Dense(32, activation='relu'),
tf.keras.layers.Dense(14, activation='softmax')
])
model.compile(optimizer='adam', loss='sparse_categorical_crossentropy', metrics=['accuracy'])
model.fit(X_train, y_train, validation_data=(X_test, y_test), epochs=10)
```
这里使用了一个简单的神经网络模型,包括一个输入层、一个隐藏层和一个输出层,其中隐藏层的激活函数为ReLU,输出层的激活函数为softmax。模型使用adam优化器和sparse_categorical_crossentropy损失函数进行训练,并在测试集上进行了验证。模型训练完成后,可以使用以下代码进行预测:
```python
y_pred = model.predict(X_test)
y_pred = [y.argmax() for y in y_pred]
```
可以使用sklearn提供的classification_report函数查看模型的精确度、召回率和F1值等指标:
```python
from sklearn.metrics import classification_report
print(classification_report(y_test, y_pred))
```
这样就完成了THUCNews数据集分类的Python代码实践。
阅读全文
相关推荐
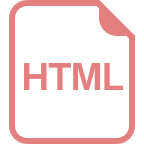








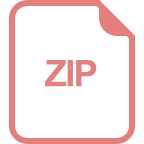
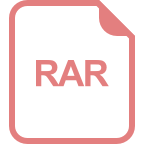
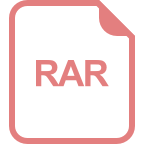
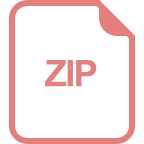

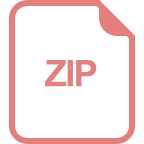
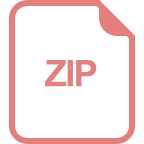
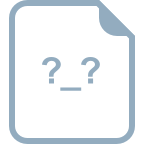