function [Sigma,S,CostNew] = graphupdate(KH,Sigma,GradNew,CostNew,option) gold = (sqrt(5)+1)/2 ; SigmaNew = SigmaInit= Sigma ; NormGrad = sum(abs(GradNew)); CostOld=CostNew=GradNew/NormGrad; [val,coord] = max(SigmaNew) ; GradNew = GradNew - GradNew(coord); desc = - GradNew.* ( (SigmaNew>0) | (GradNew<0) ); desc(coord) = - sum(desc); stepmin = 0; costmin = CostOld; costmax = 0; ind = find(desc<0); stepmax = min(-(SigmaNew(ind))./desc(ind)); deltmax = stepmax; if isempty(stepmax) || stepmax==0 Sigma = SigmaNew; return end if stepmax > 0.1 stepmax=0.1; end while costmax<costmin [costmax, S] = costgraph(KH,stepmax,desc,SigmaNew); if costmax<costmin costmin = costmax; SigmaNew = SigmaNew + stepmax * desc; desc = desc .* ( (SigmaNew>option.numericalprecision)|(desc>0)); desc(coord) = - sum(desc([[1:coord-1] [coord+1:end]])); ind = find(desc<0); if ~isempty(ind) stepmax = min(-(SigmaNew(ind))./desc(ind)); deltmax = stepmax; costmax = 0; else stepmax = 0; deltmax = 0; end end end Step = [stepmin stepmax]; Cost = [costmin costmax]; [val,coord] = min(Cost); while (stepmax-stepmin)>option.goldensearch_deltmax*(abs(deltmax)) && stepmax > eps stepmedr = stepmin+(stepmax-stepmin)/gold; stepmedl = stepmin+(stepmedr-stepmin)/gold; [costmedr, S1] = costgraph(KH,stepmedr,desc,SigmaNew); [costmedl, S2] = costgraph(KH,stepmedl,desc,SigmaNew); Step = [stepmin stepmedl stepmedr stepmax]; Cost = [costmin costmedl costmedr costmax]; [val,coord] = min(Cost); switch coord case 1 stepmax = stepmedl; costmax = costmedl; S = S2; case 2 stepmax = stepmedr; costmax = costmedr; S = S2; case 3 stepmin = stepmedl; costmin = costmedl; S = S2; case 4 stepmin = stepmedr; costmin = costmedr; S = S1; end end
时间: 2023-12-19 18:07:16 浏览: 116
这段代码是一个用于更新图的算法,它使用了黄金分割法来找到最小化代价函数的步长。主要输入参数包括KH(图的邻接矩阵)、Sigma(节点的位置坐标)、GradNew(节点的梯度信息)、CostNew(节点代价函数值)和option(算法参数)。输出参数包括Sigma(更新后的节点位置坐标)、S(更新后的图形结构)和CostNew(更新后的节点代价函数值)。
该算法的核心思想是通过调整节点位置坐标来最小化整个图的代价函数。在每次迭代中,算法会计算当前节点位置的梯度信息,并根据梯度信息和当前节点位置坐标来计算出一个方向(desc),然后使用黄金分割法来找到最小化代价函数的步长。最终,通过更新节点位置坐标来更新整个图的结构,并计算出新的代价函数值。
相关问题
详细解释这段代码function [Sigma,S,CostNew] = graphupdate(KH,Sigma,GradNew,CostNew,option) gold = (sqrt(5)+1)/2 ; SigmaNew = SigmaInit= Sigma ; NormGrad = sum(abs(GradNew)); CostOld=CostNew=GradNew/NormGrad; [val,coord] = max(SigmaNew) ; GradNew = GradNew - GradNew(coord); desc = - GradNew.* ( (SigmaNew>0) | (GradNew<0) ); desc(coord) = - sum(desc); stepmin = 0; costmin = CostOld; costmax = 0; ind = find(desc<0); stepmax = min(-(SigmaNew(ind))./desc(ind)); deltmax = stepmax; if isempty(stepmax) || stepmax==0 Sigma = SigmaNew; return end if stepmax > 0.1 stepmax=0.1; end while costmax<costmin [costmax, S] = costgraph(KH,stepmax,desc,SigmaNew); if costmax<costmin costmin = costmax; SigmaNew = SigmaNew + stepmax * desc; desc = desc .* ( (SigmaNew>option.numericalprecision)|(desc>0)); desc(coord) = - sum(desc([[1:coord-1] [coord+1:end]])); ind = find(desc<0); if ~isempty(ind) stepmax = min(-(SigmaNew(ind))./desc(ind)); deltmax = stepmax; costmax = 0; else stepmax = 0; deltmax = 0; end end end Step = [stepmin stepmax]; Cost = [costmin costmax]; [val,coord] = min(Cost); while (stepmax-stepmin)>option.goldensearch_deltmax*(abs(deltmax)) && stepmax > eps stepmedr = stepmin+(stepmax-stepmin)/gold; stepmedl = stepmin+(stepmedr-stepmin)/gold; [costmedr, S1] = costgraph(KH,stepmedr,desc,SigmaNew); [costmedl, S2] = costgraph(KH,stepmedl,desc,SigmaNew); Step = [stepmin stepmedl stepmedr stepmax]; Cost = [costmin costmedl costmedr costmax]; [val,coord] = min(Cost); switch coord case 1 stepmax = stepmedl; costmax = costmedl; S = S2; case 2 stepmax = stepmedr; costmax = costmedr; S = S2; case 3 stepmin = stepmedl; costmin = costmedl; S = S2; case 4 stepmin = stepmedr; costmin = costmedr; S = S1; end end
这段代码实现了一个图更新算法,用于优化一个图的布局。具体而言,输入参数包括:
- KH: 图的邻接矩阵;
- Sigma: 布局矩阵,即每个点在二维空间中的坐标;
- GradNew: 梯度向量,表示当前布局的梯度;
- CostNew: 当前布局的代价;
- option: 控制图更新算法的参数。
根据输入参数,该算法首先计算出当前梯度的模长NormGrad,并将GradNew除以该模长,以避免梯度大小对更新步长的影响。然后,算法依次进行以下步骤:
1. 初始化SigmaNew为当前布局,SigmaInit为当前布局的备份。
2. 找到SigmaNew中的最大值和对应的坐标coord,将GradNew中在该坐标上的梯度从GradNew中减去,以避免在该坐标方向上的更新。
3. 计算更新方向desc,其中对于SigmaNew中小于等于0的元素,不需要在该维度上进行更新;对于GradNew中小于0的元素,也不需要在该维度上进行更新。
4. 设置stepmin和stepmax为合适的初值,并计算在stepmax处的代价costmax和在stepmin处的代价costmin。如果desc中没有小于0的元素,则返回SigmaNew作为更新后的布局。
5. 在[stepmin, stepmax]区间内使用黄金分割法寻找代价最小的更新步长。具体而言,算法将该区间分成左右两个子区间,计算在每个子区间的中点处的代价,然后选择代价更小的子区间继续寻找。这个过程一直进行,直到更新步长的变化量小于设定的阈值goldensearch_deltmax或者步长stepmax小于一个极小值eps。
6. 最后返回更新后的布局矩阵Sigma、更新后的代价CostNew和更新后的图S。
解释这段代码:function [Sigma,S,CostNew] = graphupdate(KH,Sigma,GradNew,CostNew,option) %------------------------------------------------------------------------------% % Initialize初始化 %------------------------------------------------------------------------------% gold = (sqrt(5)+1)/2 ; SigmaInit = Sigma; SigmaNew = SigmaInit; NormGrad = sum(abs(GradNew)); GradNew=GradNew/NormGrad; CostOld=CostNew;
这段代码实现了一个名为 "graphupdate" 的函数,并定义了输入参数 KH、Sigma、GradNew、CostNew 和 option,以及输出参数 Sigma、S 和 CostNew。
接下来是函数的主体部分,它开始执行初始化操作。gold 变量被初始化为黄金比例,SigmaInit 和 SigmaNew 变量被初始化为输入参数 Sigma。NormGrad 变量计算了 GradNew 的绝对值之和,GradNew 变量被标准化为其绝对值之和,以便在后续计算过程中进行更准确的比较。CostOld 变量被初始化为 CostNew。
这段代码实现了一个优化算法的一步更新过程,具体实现需要结合后续代码进行分析。
阅读全文
相关推荐
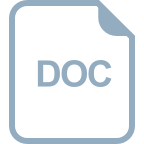
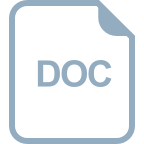
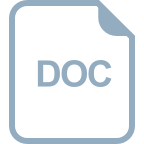
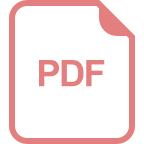
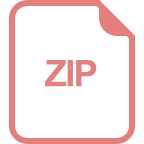
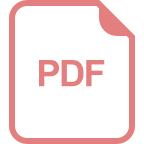
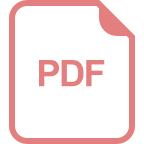
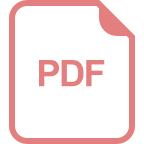
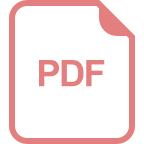
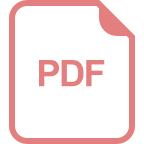
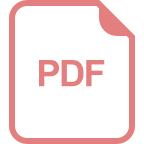
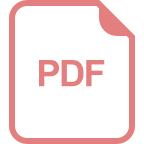
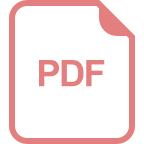
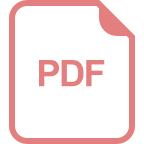
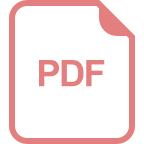
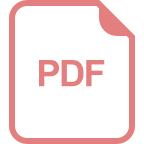
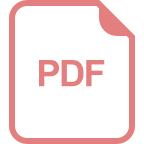