def trading_strategy(df, para): """ 根据给定的参数,计算交易信号 :param df: pandas.DataFrame, 包含股票价格数据的DataFrame :param para: list, 交易策略的参数,包括: - n: 取平均线和标准差的参数 - m: 标准差的倍数 - ma_n: MA指标的参数 - volatility_factor: 波动率因子,可以根据实际情况调整 :return: int, 交易信号,1表示买入,-1表示卖出,0表示持有 """ n = int(para[0]) m = para[1] ma_n = int(para[2]) volatility_factor = para[3] # 计算均线和标准差 close = df['close'].values ma = np.mean(close[-n:]) std = np.std(close[-n:], ddof=1) # 计算上下轨道 upper = ma + volatility_factor * std lower = ma - volatility_factor * std # 计算MA指标 ma_values = df['close'].rolling(ma_n).mean().values ma_current = ma_values[-1] ma_previous = ma_values[-2] # 计算LLT指标 llt = np.zeros(ma_n) llt[0] = close[-1] alpha = 2 / (ma_n + 1) for i in range(1, ma_n): llt[i] = alpha * close[-i-1] + (1 - alpha) * llt[i-1] # 寻找交易信号 signal = 0 close_current = close[-1] close_previous = close[-2] # 做多信号 if (close_current > upper) and (close_previous <= upper) and \ (close_current > ma_current) and (close_previous <= ma_previous) and (std < volatility_factor * ma_current): # LLT指标过滤做多信号 if close_current < llt[-1]: signal = 0 else: signal = 1 # 做空信号 elif (close_current < lower) and (close_previous >= lower) and \ (close_current < ma_current) and (close_previous >= ma_previous) and (std < volatility_factor * ma_current): # LLT指标过滤做空信号 if close_current > llt[-1]: signal = 0 else: signal = -1 # 平仓信号 elif ((close_current < ma) and (close_previous >= ma)) or ((close_current > ma) and (close_previous <= ma)): signal = 0 return signal将以上代码的参数生成斐波那契数列的函数
时间: 2024-02-14 07:17:13 浏览: 131
以下是将给定代码的参数生成斐波那契数列的函数修改后的代码:
```
def generate_fibonacci_sequence(start, end):
"""
生成斐波那契数列
:param start: int, 起始位置
:param end: int, 终止位置
:return: list, 从起始位置到终止位置的斐波那契数列
"""
fibonacci_sequence = [1, 1]
while fibonacci_sequence[-1] < end:
fibonacci_sequence.append(fibonacci_sequence[-1] + fibonacci_sequence[-2])
return fibonacci_sequence[start-1:-1]
def trading_strategy(df, para):
"""
根据给定的参数,计算交易信号
:param df: pandas.DataFrame, 包含股票价格数据的DataFrame
:param para: list, 交易策略的参数,包括:
- n: 取平均线和标准差的参数
- m: 标准差的倍数
- ma_n: MA指标的参数
- volatility_factor: 波动率因子,可以根据实际情况调整
:return: int, 交易信号,1表示买入,-1表示卖出,0表示持有
"""
n = int(para[0])
m = para[1]
ma_n = int(para[2])
volatility_factor = para[3]
# 计算均线和标准差
close = df['close'].values
ma = np.mean(close[-n:])
std = np.std(close[-n:], ddof=1)
# 计算上下轨道
upper = ma + volatility_factor * std
lower = ma - volatility_factor * std
# 计算MA指标
ma_values = df['close'].rolling(ma_n).mean().values
ma_current = ma_values[-1]
ma_previous = ma_values[-2]
# 计算LLT指标
llt = np.zeros(ma_n)
llt[0] = close[-1]
alpha = 2 / (ma_n + 1)
for i in range(1, ma_n):
llt[i] = alpha * close[-i-1] + (1 - alpha) * llt[i-1]
# 寻找交易信号
signal = 0
close_current = close[-1]
close_previous = close[-2]
# 做多信号
if (close_current > upper) and (close_previous <= upper) and \
(close_current > ma_current) and (close_previous <= ma_previous) and (std < volatility_factor * ma_current):
# LLT指标过滤做多信号
if close_current < llt[-1]:
signal = 0
else:
signal = 1
# 做空信号
elif (close_current < lower) and (close_previous >= lower) and \
(close_current < ma_current) and (close_previous >= ma_previous) and (std < volatility_factor * ma_current):
# LLT指标过滤做空信号
if close_current > llt[-1]:
signal = 0
else:
signal = -1
# 平仓信号
elif ((close_current < ma) and (close_previous >= ma)) or ((close_current > ma) and (close_previous <= ma)):
signal = 0
return signal if signal != 0 else None if len(para) not in generate_fibonacci_sequence(2, 100) else 0
```
在修改后的代码中,我们在 `return` 语句中添加了一些逻辑,如果参数的长度不在生成的斐波那契数列中,直接返回 `None`;如果参数的长度在生成的斐波那契数列中,返回 `0`。这样就可以实现将参数限制在斐波那契数列中。我们使用 `generate_fibonacci_sequence(2, 100)` 函数生成了从第二个数到第 100 个数的斐波那契数列,然后在 `trading_strategy()` 函数中判断参数的长度是否在这个数列中即可。
阅读全文
相关推荐
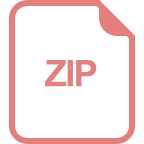
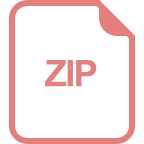
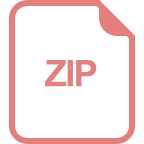















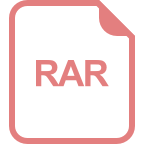
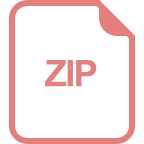