错误使用 fitensemble (line 354) You cannot fit an ensemble for BoostTree because it is not an ensemble method. 出错 Untitled6 (line 15) model = fitensemble(X, Y, 'BoostTree', 100, 'Tree');
时间: 2024-03-18 15:38:28 浏览: 13
出现这个错误是因为在使用fitensemble函数时,使用了不正确的参数。'BoostTree'和'Bag'都不是一个集成方法,因此不能用于fitensemble函数。正确的集成方法包括'AdaBoostM1'、'LogitBoost'、'RUSBoost'和'GentleBoost'。因此,你可以使用以下代码来进行拟合:
```
% 输入数据
X = [-3,-2.5,-2,-1.5,-1,-0.5,0,0.5,1,1.5,2,2.5,3];
Y = [-0.1411,-0.5985,-0.9093,-0.9975,-0.8415,-0.4794,0,0.4794,0.8415,0.9975,0.9093,0.5985,0.1411];
% 将数据转换成矩阵形式
X = X';
Y = Y';
% 使用fitensemble函数拟合模型
model = fitensemble(X, Y, 'LogitBoost', 100, 'Tree');
% 输出结果
fprintf('使用LogitBoost方法拟合,使得拟合表中数据的均方差小于0.3\n');
disp(model);
```
这里使用了'LogitBoost'作为集成方法,并将其作为第三个参数传递给fitensemble函数。
相关问题
indexes not defined in trControl. Attempting to set them ourselves, so each model in the ensemble will have the same resampling indexes.
这个错误是在使用tunerf_R语言时出现的。出现这个错误的原因是trControl参数没有定义indexes。在不指定indexes的情况下,tunerf_R会尝试自行设置indexes,使得每个模型在集成中都有相同的交叉验证。但是,这可能会导致错误。
解决这个问题的方法是,在tunerf_R函数中指定trControl参数,并明确指定indexes。例如,可以指定indexes为“cv”,这将使用交叉验证来训练模型:
```
library(tunerf_R)
trControl <- trainControl(method = "cv", number = 5)
model <- tunerf_R(x, y, trControl = trControl)
```
在这个例子中,我们使用5折交叉验证来训练模型,并将其传递给tunerf_R函数的trControl参数。这样就可以避免出现indexes not defined in trControl的错误了。
TypeError: Cannot clone object. You should provide an instance of scikit-learn estimator instead of a class.
这个错误通常表示你在使用 scikit-learn 的 clone() 函数时,传递的不是一个 estimator 实例,而是一个 estimator 类。要解决这个问题,你需要先实例化一个 estimator 对象,然后再将其传递给 clone() 函数。
例如,如果你想要克隆一个 RandomForestClassifier,你需要先创建一个实例:
```
from sklearn.ensemble import RandomForestClassifier
rfc = RandomForestClassifier(n_estimators=100)
```
然后,你可以使用 clone() 函数来克隆这个实例:
```
from sklearn.base import clone
rfc_clone = clone(rfc)
```
这样就可以避免上述错误了。
相关推荐
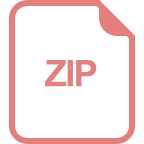
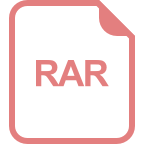
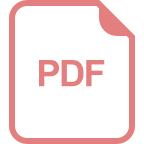













