CameraParameters:= ['area_scan_division',0.00640046,10205.5,1.91206e-06,1.9e-06,1503.65,1915.07,3024,4032] CameraPose := [0.000284489,0.00953188,0.226499,10.3425,0.0209458,0.458095,0] CamParVirtualFixed:=CameraParameters dev_close_window () dev_open_window (0, 0, 360, 270, 'black', WindowHandle) picture_number := 50 for Index := 1 to picture_number by 1 read_image (Image, 'C:/Users/Lenovo/Desktop/视觉课作业/大作业/卡片正面照片/' + str(Index, '0.4f') + '.JPG') gen_radial_distortion_map(MapFixed,CameraParameters,CamParVirtualFixed,'bilinear') map_image(Image,MapFixed,ImageRectifiedFixed) write_image (ImageRectifiedFixed, 'png', 0, 'C:/Users/Lenovo/Desktop/视觉课作业/大作业/正面畸变校正后/' + str(Index, '0.4f') + '.JPG') endfor
时间: 2024-02-05 19:04:25 浏览: 20
这段代码是用Halcon实现针对一组卡片正面照片进行畸变校正的操作。
1. 首先定义了相机参数和相机姿态:
```
CameraParameters:= ['area_scan_division',0.00640046,10205.5,1.91206e-06,1.9e-06,1503.65,1915.07,3024,4032]
CameraPose := [0.000284489,0.00953188,0.226499,10.3425,0.0209458,0.458095,0]
```
2. 打开一个窗口:
```
dev_close_window ()
dev_open_window (0, 0, 360, 270, 'black', WindowHandle)
```
3. 循环处理每张卡片正面照片:
```
picture_number := 50
for Index := 1 to picture_number by 1
read_image (Image, 'C:/Users/Lenovo/Desktop/视觉课作业/大作业/卡片正面照片/' + str(Index, '0.4f') + '.JPG')
gen_radial_distortion_map(MapFixed,CameraParameters,CamParVirtualFixed,'bilinear')
map_image(Image,MapFixed,ImageRectifiedFixed)
write_image (ImageRectifiedFixed, 'png', 0, 'C:/Users/Lenovo/Desktop/视觉课作业/大作业/正面畸变校正后/' + str(Index, '0.4f') + '.JPG')
endfor
```
在循环中,首先读取当前处理的卡片正面照片,然后使用gen_radial_distortion_map函数生成畸变矫正映射MapFixed。接着使用map_image函数对卡片正面照片进行畸变校正,并将校正后的图像保存到指定的目录下。循环处理所有的卡片正面照片后,程序结束。
需要注意的是,这段代码中的相机参数和相机姿态是针对特定相机和场景的,如果你的相机或者场景不同,需要重新定义相机参数和相机姿态。
相关推荐
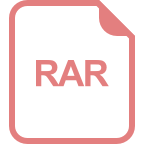
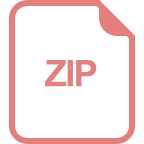















