def reports (test_loader, y_test, name, net, device): count = 0 # 模型测试 for inputs, _ in test_loader: inputs = inputs.to(device) outputs = net(inputs) outputs = np.argmax(outputs.detach().cpu().numpy(), axis=1) if count == 0: y_pred = outputs count = 1 else: y_pred = np.concatenate( (y_pred, outputs) ) if name == 'IP': target_names = ['Wheat', 'Woods']
时间: 2023-06-01 16:04:26 浏览: 77
这段代码定义了一个函数 reports,接受五个参数,分别是 test_loader(测试数据集的加载器)、y_test(测试数据集的标签)、name(模型的名称)、net(神经网络模型)和 device(设备,如 CPU 或 GPU)。
函数中定义了一个变量 count,并将其初始化为 0。
相关问题
def reports (test_loader, y_test, name, net, device): count = 0 for inputs, _ in test_loader: inputs = inputs.to(device) outputs = net(inputs) outputs = np.argmax(outputs.detach().cpu().numpy(), axis=1) if count == 0: y_pred = outputs count = 1 else: y_pred = np.concatenate( (y_pred, outputs) ) if name == 'IP': target_names = ['Wheat', 'Woods']
elif name == 'MNIST': target_names = ['0', '1', '2', '3', '4', '5', '6', '7', '8', '9'] else: target_names = ['Class 0', 'Class 1', 'Class 2', 'Class 3', 'Class 4'] print(classification_report(y_test, y_pred, target_names=target_names))
model.eval() with torch.no_grad(): j = 0 barl = tqdm(enumerate(data_test_loader), desc='accary', total=len(data_test_loader), colour='blue') for step, data in barl: inputs, labels = data inputs = inputs.to(device) # labels = labels.to(device) outputs = model(inputs) pred_y = torch.max(outputs, 1)[1].cpu().detach().numpy() # torch.max labels = labels.detach().numpy() # torch.max for i in range(len(pred_y)): if pred_y[i] == labels[i]: j += 1 print('accary:', j / len(data_test))
这段代码是用来计算模型在测试集上的准确率,其中model.eval()表示将模型设置为评估模式,torch.no_grad()表示在评估模式下不需要计算梯度,j是正确预测的样本数,barl是一个进度条,用于显示当前处理的进度,step表示当前处理的批次,data表示当前批次的数据,inputs表示输入数据,labels表示标签,outputs表示模型的输出,pred_y表示预测的标签,labels表示真实标签,len(pred_y)表示当前批次的样本数。最后通过除以总样本数来计算准确率并打印输出。
阅读全文
相关推荐
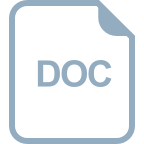
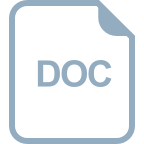
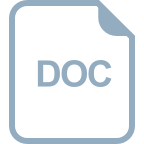









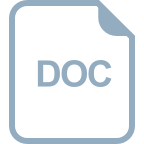
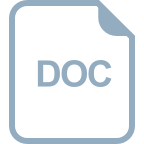
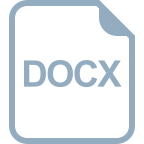