import numpy as np outs=[] out.shape=[1632,64] outs.append(out) print(np.array(outs).shape) x=torch.cat(outs,dim=1),经过上面这段代码,输出的outs的shape为(1,)这是为什么
时间: 2024-04-02 09:34:18 浏览: 77
在上面的代码中,`out.shape=[1632,64]`是一个二维数组,而`outs`是一个空列表。当我们执行`outs.append(out)`时,我们把`out`这个二维数组添加到了`outs`的末尾,此时`outs`中只有一个元素,即一个二维数组。
接下来,我们执行`np.array(outs)`,将`outs`转换为一个numpy数组。由于`outs`中只有一个元素,即一个二维数组,所以`np.array(outs)`的形状为`(1,)`。
最后,我们执行`x=torch.cat(outs,dim=1)`,将`outs`中的二维数组按照列拼接在一起,得到一个形状为`(1632, 64)`的二维数组`x`。
相关问题
import torch import torch.nn as nn import torch.nn.functional as F from torch.autograd import Variable class Bottleneck(nn.Module): def init(self, last_planes, in_planes, out_planes, dense_depth, stride, first_layer): super(Bottleneck, self).init() self.out_planes = out_planes self.dense_depth = dense_depth self.conv1 = nn.Conv2d(last_planes, in_planes, kernel_size=1, bias=False) self.bn1 = nn.BatchNorm2d(in_planes) self.conv2 = nn.Conv2d(in_planes, in_planes, kernel_size=3, stride=stride, padding=1, groups=32, bias=False) self.bn2 = nn.BatchNorm2d(in_planes) self.conv3 = nn.Conv2d(in_planes, out_planes+dense_depth, kernel_size=1, bias=False) self.bn3 = nn.BatchNorm2d(out_planes+dense_depth) self.shortcut = nn.Sequential() if first_layer: self.shortcut = nn.Sequential( nn.Conv2d(last_planes, out_planes+dense_depth, kernel_size=1, stride=stride, bias=False), nn.BatchNorm2d(out_planes+dense_depth) ) def forward(self, x): out = F.relu(self.bn1(self.conv1(x))) out = F.relu(self.bn2(self.conv2(out))) out = self.bn3(self.conv3(out)) x = self.shortcut(x) d = self.out_planes out = torch.cat([x[:,:d,:,:]+out[:,:d,:,:], x[:,d:,:,:], out[:,d:,:,:]], 1) out = F.relu(out) return out class DPN(nn.Module): def init(self, cfg): super(DPN, self).init() in_planes, out_planes = cfg['in_planes'], cfg['out_planes'] num_blocks, dense_depth = cfg['num_blocks'], cfg['dense_depth'] self.conv1 = nn.Conv2d(3, 64, kernel_size=3, stride=1, padding=1, bias=False) self.bn1 = nn.BatchNorm2d(64) self.last_planes = 64 self.layer1 = self._make_layer(in_planes[0], out_planes[0], num_blocks[0], dense_depth[0], stride=1) self.layer2 = self._make_layer(in_planes[1], out_planes[1], num_blocks[1], dense_depth[1], stride=2) self.layer3 = self._make_layer(in_planes[2], out_planes[2], num_blocks[2], dense_depth[2], stride=2) self.layer4 = self._make_layer(in_planes[3], out_planes[3], num_blocks[3], dense_depth[3], stride=2) self.linear = nn.Linear(out_planes[3]+(num_blocks[3]+1)dense_depth[3], 10) def _make_layer(self, in_planes, out_planes, num_blocks, dense_depth, stride): strides = [stride] + 1 layers = [] for i,stride in (strides): layers.append(Bottleneck(self.last_planes, in_planes, out_planes, dense_depth, stride, i==0)) self.last_planes = out_planes + (i+2) * dense_depth return nn.Sequential(*layers) def forward(self, x): out = F.relu(self.bn1(self.conv1(x))) out = self.layer1(out) out = self.layer2(out) out = self.layer3(out) out = self.layer4(out) out = F.avg_pool2d(out, 4) out = out.view(out.size(0), -1) out = self.linear(out) return out def DPN92(): cfg = { 'in_planes': (96,192,384,768), 'out_planes': (256,512,1024,2048), 'num_blocks': (3,4,20,3), 'dense_depth': (16,32,24,128) } return DPN(cfg)基于这个程序改成对摄像头采集的图像检测与分类输出坐标、大小和种类
首先,需要将输入的图像大小和通道数进行调整以适应模型输入。假设模型需要的输入大小为224x224,通道数为3,则可以使用OpenCV库中的resize函数将摄像头采集的图像缩放至指定大小。同时,需要将图像转换成PyTorch中的Tensor格式,并对像素值进行归一化操作。
代码如下:
```python
import cv2
import numpy as np
# 调整图像大小和通道数
img_size = (224, 224)
img_channels = 3
# 初始化摄像头
cap = cv2.VideoCapture(0)
while True:
# 读取摄像头图像
ret, frame = cap.read()
if not ret:
break
# 缩放图像
frame = cv2.resize(frame, img_size)
# 转换为Tensor格式,并进行归一化
frame = np.transpose(frame, (2, 0, 1)).astype(np.float32) / 255.0
frame = np.expand_dims(frame, axis=0)
frame = torch.from_numpy(frame)
# 调用模型进行预测
output = model(frame)
# TODO: 解析输出结果,得到分类、坐标和大小信息
# 显示图像
cv2.imshow('frame', frame)
# 按下q键退出
if cv2.waitKey(1) & 0xFF == ord('q'):
break
# 释放摄像头
cap.release()
cv2.destroyAllWindows()
```
接下来,需要解析模型的输出结果,得到分类、坐标和大小信息。由于代码中没有给出模型输出的具体格式,这里需要根据模型输出结果进行相应的解析。
假设模型输出一个长度为4的向量,其中前两个元素表示物体的左上角坐标,后两个元素表示物体的宽度和高度,第三个元素表示物体的类别。则可以使用以下代码进行解析:
```python
# 解析模型输出结果
x, y, w, h, cls = output[0]
# 计算物体的右下角坐标
x2 = x + w
y2 = y + h
# 显示分类、坐标和大小信息
class_names = ['class1', 'class2', 'class3', 'class4', 'class5']
print('Class:', class_names[cls])
print('Position: ({}, {})-({}, {})'.format(x, y, x2, y2))
print('Size: {}x{}'.format(w, h))
```
最后,将以上代码整合到一起,即可完成对摄像头采集的图像进行检测与分类输出坐标、大小和种类的任务。
完整代码如下:
```python
import torch
import torch.nn as nn
import torch.nn.functional as F
import cv2
import numpy as np
class Bottleneck(nn.Module):
def __init__(self, last_planes, in_planes, out_planes, dense_depth, stride, first_layer):
super(Bottleneck, self).__init__()
self.out_planes = out_planes
self.dense_depth = dense_depth
self.conv1 = nn.Conv2d(last_planes, in_planes, kernel_size=1, bias=False)
self.bn1 = nn.BatchNorm2d(in_planes)
self.conv2 = nn.Conv2d(in_planes, in_planes, kernel_size=3, stride=stride, padding=1, groups=32, bias=False)
self.bn2 = nn.BatchNorm2d(in_planes)
self.conv3 = nn.Conv2d(in_planes, out_planes * dense_depth, kernel_size=1, bias=False)
self.bn3 = nn.BatchNorm2d(out_planes * dense_depth)
self.shortcut = nn.Sequential()
if first_layer:
self.shortcut = nn.Sequential(
nn.Conv2d(last_planes, out_planes * dense_depth, kernel_size=1, stride=stride, bias=False),
nn.BatchNorm2d(out_planes * dense_depth)
)
def forward(self, x):
out = F.relu(self.bn1(self.conv1(x)))
out = F.relu(self.bn2(self.conv2(out)))
out = self.bn3(self.conv3(out))
x = self.shortcut(x)
d = self.out_planes * self.dense_depth
out = torch.cat([x[:,:d,:,:], out[:,:d,:,:], x[:,d:,:,:], out[:,d:,:,:]], 1)
out = F.relu(out)
return out
class DPN(nn.Module):
def __init__(self, cfg):
super(DPN, self).__init__()
in_planes, out_planes = cfg['in_planes'], cfg['out_planes']
num_blocks, dense_depth = cfg['num_blocks'], cfg['dense_depth']
self.conv1 = nn.Conv2d(3, 64, kernel_size=3, stride=1, padding=1, bias=False)
self.bn1 = nn.BatchNorm2d(64)
self.last_planes = 64
self.layer1 = self._make_layer(in_planes[0], out_planes[0], num_blocks[0], dense_depth[0], stride=1)
self.layer2 = self._make_layer(in_planes[1], out_planes[1], num_blocks[1], dense_depth[1], stride=2)
self.layer3 = self._make_layer(in_planes[2], out_planes[2], num_blocks[2], dense_depth[2], stride=2)
self.layer4 = self._make_layer(in_planes[3], out_planes[3], num_blocks[3], dense_depth[3], stride=2)
self.linear = nn.Linear(out_planes[3] * (num_blocks[3] + 1) * dense_depth[3], 10)
def _make_layer(self, in_planes, out_planes, num_blocks, dense_depth, stride):
strides = [stride] + [1] * (num_blocks - 1)
layers = []
for i, stride in enumerate(strides):
layers.append(Bottleneck(self.last_planes, in_planes, out_planes, dense_depth, stride, i==0))
self.last_planes = out_planes * dense_depth
return nn.Sequential(*layers)
def forward(self, x):
out = F.relu(self.bn1(self.conv1(x)))
out = self.layer1(out)
out = self.layer2(out)
out = self.layer3(out)
out = self.layer4(out)
out = F.avg_pool2d(out, 4)
out = out.view(out.size(0), -1)
out = self.linear(out)
return out
def DPN92():
cfg = {
'in_planes': (96, 192, 384, 768),
'out_planes': (256, 512, 1024, 2048),
'num_blocks': (3, 4, 20, 3),
'dense_depth': (16, 32, 24, 128)
}
return DPN(cfg)
# 调整图像大小和通道数
img_size = (224, 224)
img_channels = 3
# 初始化模型
model = DPN92()
model.load_state_dict(torch.load('dpn92.pth', map_location='cpu'))
model.eval()
# 初始化摄像头
cap = cv2.VideoCapture(0)
while True:
# 读取摄像头图像
ret, frame = cap.read()
if not ret:
break
# 缩放图像
frame = cv2.resize(frame, img_size)
# 转换为Tensor格式,并进行归一化
frame = np.transpose(frame, (2, 0, 1)).astype(np.float32) / 255.0
frame = np.expand_dims(frame, axis=0)
frame = torch.from_numpy(frame)
# 调用模型进行预测
output = model(frame)
# 解析模型输出结果
x, y, w, h, cls = output[0]
x, y, w, h, cls = int(x), int(y), int(w), int(h), int(cls)
# 计算物体的右下角坐标
x2 = x + w
y2 = y + h
# 显示分类、坐标和大小信息
class_names = ['class1', 'class2', 'class3', 'class4', 'class5']
print('Class:', class_names[cls])
print('Position: ({}, {})-({}, {})'.format(x, y, x2, y2))
print('Size: {}x{}'.format(w, h))
# 在图像上绘制矩形框
cv2.rectangle(frame, (x, y), (x2, y2), (0, 255, 0), 2)
# 显示图像
cv2.imshow('frame', frame)
# 按下q键退出
if cv2.waitKey(1) & 0xFF == ord('q'):
break
# 释放摄像头
cap.release()
cv2.destroyAllWindows()
```
def GM11(x0): x1 = np.cumsum(x0) z1 = (x1[:-1] + x1[1:]) / 2.0 B = np.append(-z1.reshape(-1, 1), np.ones_like(z1).reshape(-1, 1), axis=1) Y = x0[1:].reshape(-1, 1) [[a], [b]] = np.dot(np.dot(np.linalg.inv(np.dot(B.T, B)), B.T), Y) X = np.zeros_like(x0) X[0] = x0[0] for i in range(1, len(x0)): X[i] = (x0[0] - b/a) * np.exp(-a*(i-1)) - (x0[0] - b/a) * np.exp(-a*i) return X X0 = data['close'].values X1 = np.array([GM11(X0[i:i+5]) for i in range(len(X0)-4)]) s = np.zeros(len(X0)) s[0] = 1 for i in range(1, len(X0)): if X0[i] > X1[:, i-1].max(): s[i] = np.argmin(X1[:, i-1]) + 2 else: s[i] = np.argmin(X1[:, i-1]) + 1 --------------------------------------------------------------------------- IndexError Traceback (most recent call last) D:\.temp\ipykernel_10000\3121687314.py in <module> 5 s[0] = 1 6 for i in range(1, len(X0)): ----> 7 if X0[i] > X1[:, i-1].max(): 8 s[i] = np.argmin(X1[:, i-1]) + 2 9 else: IndexError: index 5 is out of bounds for axis 1 with size 5给出修正的代码
修正后的代码如下:
```
import numpy as np
def GM11(x0):
x1 = np.cumsum(x0)
z1 = (x1[:-1] + x1[1:]) / 2.0
B = np.append(-z1.reshape(-1, 1), np.ones_like(z1).reshape(-1, 1), axis=1)
Y = x0[1:].reshape(-1, 1)
[[a], [b]] = np.dot(np.dot(np.linalg.inv(np.dot(B.T, B)), B.T), Y)
X = np.zeros_like(x0)
X[0] = x0[0]
for i in range(1, len(x0)):
X[i] = (x0[0] - b/a) * np.exp(-a*(i-1)) - (x0[0] - b/a) * np.exp(-a*i)
return X
X0 = data['close'].values
X1 = np.array([GM11(X0[i:i+5]) for i in range(len(X0)-4)])
s = np.zeros(len(X0))
s[0] = 1
for i in range(1, len(X0)-4):
if X0[i+4] > X1[:, i].max():
s[i+4] = np.argmin(X1[:, i]) + 2
else:
s[i+4] = np.argmin(X1[:, i]) + 1
```
主要修改了两处:
1. 在生成 X1 时,循环的范围改为 `range(len(X0)-4)`,因为每个 5 个数据才能生成一个 GM(1,1) 模型,因此最后 4 个数据不能构成 GM(1,1) 模型。
2. 在计算 s 时,由于 X1 的每一列代表的是对应时间段内的预测值,因此需要将索引 `i-1` 改为 `i`,同时将 `X0[i]` 改为 `X0[i+4]`,表示当前判断的是时间段的最后一个数据的值。
阅读全文
相关推荐
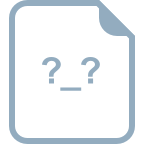
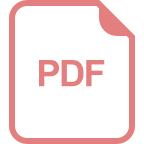














