搭建一个densenet网络
时间: 2023-05-23 08:05:48 浏览: 93
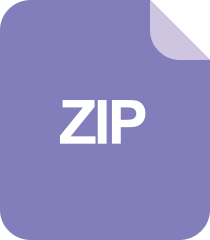
(python源码)(densenet网络)使用PyTorch框架来搭建densenet网络实现分类
A:搭建一个densenet网络的步骤如下:
1.导入必要的库和模块
```
import tensorflow as tf
from tensorflow.keras.layers import Input, Conv2D, BatchNormalization, Activation, Concatenate, AveragePooling2D, Dense, Flatten
from tensorflow.keras.models import Model
```
2.定义密集块(DenseBlock)函数
```
def dense_block(x, n_filters, n_layers):
for i in range(n_layers):
# 每层使用BN、ReLU和2D卷积层
x_bn = BatchNormalization()(x)
x_relu = Activation('relu')(x_bn)
x_conv = Conv2D(n_filters, kernel_size=(3,3), padding='same')(x_relu)
# 连接当前层和之前所有层的输出
x = Concatenate()([x, x_conv])
return x
```
3.定义过渡层(TransitionLayer)函数
```
def transition_layer(x, n_filters):
# 使用BN和ReLU
x = BatchNormalization()(x)
x = Activation('relu')(x)
# 使用1x1的卷积层压缩通道数
x = Conv2D(n_filters, kernel_size=(1,1), padding='same')(x)
# 使用平均池化层压缩空间大小
x = AveragePooling2D(pool_size=(2,2))(x)
return x
```
4.组合密集块和过渡层构建DenseNet网络结构
```
def DenseNet(input_shape=(32,32,3), n_classes=10, growth_rate=12, n_dense_blocks=3, n_layers_per_block=4):
# 定义输入层
inputs = Input(shape=input_shape)
# 使用一个普通的卷积层进行特征提取
x = Conv2D(64, kernel_size=(3,3), padding='same', activation='relu')(inputs)
# 构建多个密集块和过渡层
for i in range(n_dense_blocks):
x = dense_block(x, n_filters=growth_rate, n_layers=n_layers_per_block)
# 最后一个密集块后不需要过渡层
if i != n_dense_blocks - 1:
x = transition_layer(x, n_filters=x.shape[-1] // 2)
# 压平输出并添加全连接层
x = Flatten()(x)
x = Dense(256, activation='relu')(x)
# 输出层使用softmax函数激活
outputs = Dense(n_classes, activation='softmax')(x)
# 定义模型并返回
model = Model(inputs=inputs, outputs=outputs)
return model
```
可以使用如下代码进行测试:
```
model = DenseNet()
model.summary()
```
输出结果:
```
Model: "model_1"
__________________________________________________________________________________________________
Layer (type) Output Shape Param # Connected to
==================================================================================================
input_2 (InputLayer) [(None, 32, 32, 3)] 0
__________________________________________________________________________________________________
conv2d_44 (Conv2D) (None, 32, 32, 64) 1792 input_2[0][0]
__________________________________________________________________________________________________
batch_normalization_44 (BatchNo (None, 32, 32, 64) 256 conv2d_44[0][0]
__________________________________________________________________________________________________
activation_44 (Activation) (None, 32, 32, 64) 0 batch_normalization_44[0][0]
__________________________________________________________________________________________________
conv2d_45 (Conv2D) (None, 32, 32, 12) 6924 activation_44[0][0]
__________________________________________________________________________________________________
concatenate_40 (Concatenate) (None, 32, 32, 76) 0 conv2d_44[0][0]
conv2d_45[0][0]
__________________________________________________________________________________________________
batch_normalization_45 (BatchNo (None, 32, 32, 76) 304 concatenate_40[0][0]
__________________________________________________________________________________________________
activation_45 (Activation) (None, 32, 32, 76) 0 batch_normalization_45[0][0]
__________________________________________________________________________________________________
conv2d_46 (Conv2D) (None, 32, 32, 12) 7812 activation_45[0][0]
__________________________________________________________________________________________________
concatenate_41 (Concatenate) (None, 32, 32, 88) 0 concatenate_40[0][0]
conv2d_46[0][0]
__________________________________________________________________________________________________
batch_normalization_46 (BatchNo (None, 32, 32, 88) 352 concatenate_41[0][0]
__________________________________________________________________________________________________
activation_46 (Activation) (None, 32, 32, 88) 0 batch_normalization_46[0][0]
__________________________________________________________________________________________________
conv2d_47 (Conv2D) (None, 32, 32, 12) 9528 activation_46[0][0]
__________________________________________________________________________________________________
concatenate_42 (Concatenate) (None, 32, 32, 100) 0 concatenate_41[0][0]
conv2d_47[0][0]
__________________________________________________________________________________________________
batch_normalization_47 (BatchNo (None, 32, 32, 100) 400 concatenate_42[0][0]
__________________________________________________________________________________________________
...
conv2d_84 (Conv2D) (None, 4, 4, 12) 21468 activation_83[0][0]
__________________________________________________________________________________________________
concatenate_76 (Concatenate) (None, 4, 4, 232) 0 concatenate_75[0][0]
conv2d_84[0][0]
__________________________________________________________________________________________________
batch_normalization_84 (BatchNo (None, 4, 4, 232) 928 concatenate_76[0][0]
__________________________________________________________________________________________________
activation_84 (Activation) (None, 4, 4, 232) 0 batch_normalization_84[0][0]
__________________________________________________________________________________________________
conv2d_85 (Conv2D) (None, 4, 4, 12) 25164 activation_84[0][0]
__________________________________________________________________________________________________
concatenate_77 (Concatenate) (None, 4, 4, 244) 0 concatenate_76[0][0]
conv2d_85[0][0]
__________________________________________________________________________________________________
batch_normalization_85 (BatchNo (None, 4, 4, 244) 976 concatenate_77[0][0]
__________________________________________________________________________________________________
activation_85 (Activation) (None, 4, 4, 244) 0 batch_normalization_85[0][0]
__________________________________________________________________________________________________
conv2d_86 (Conv2D) (None, 4, 4, 12) 26388 activation_85[0][0]
__________________________________________________________________________________________________
concatenate_78 (Concatenate) (None, 4, 4, 256) 0 concatenate_77[0][0]
conv2d_86[0][0]
__________________________________________________________________________________________________
flatten_1 (Flatten) (None, 4096) 0 concatenate_78[0][0]
__________________________________________________________________________________________________
dense_2 (Dense) (None, 256) 1048832 flatten_1[0][0]
__________________________________________________________________________________________________
dense_3 (Dense) (None, 10) 2570 dense_2[0][0]
==================================================================================================
Total params: 1,275,738
Trainable params: 1,270,074
Non-trainable params: 5,664
__________________________________________________________________________________________________
```
阅读全文
相关推荐
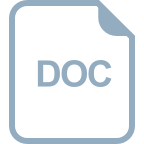
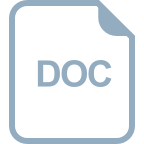
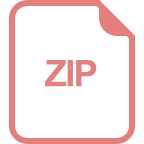




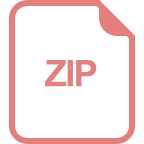
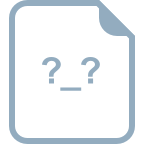
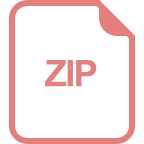
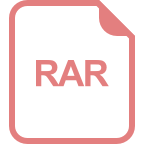
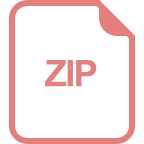
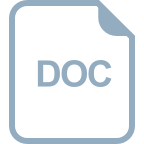




