out of the box模型
时间: 2023-12-30 11:05:15 浏览: 29
Out of the box模型是指能够直接使用或者只需要进行少量调整就能够在各种场景下进行有效工作的模型。这些模型通常是由经验丰富的机器学习和深度学习专家设计和训练的,具有广泛的适用性和高度的可重用性。这些模型通常包括预训练模型和已经训练好的模型,例如ImageNet,BERT和GPT等。使用这些模型可以大大减少模型训练和调整的工作量,同时也可以提高模型的准确性和鲁棒性。
相关问题
def forward(self, samples: NestedTensor): """ The forward expects a NestedTensor, which consists of: - samples.tensor: batched images, of shape [batch_size x 3 x H x W] - samples.mask: a binary mask of shape [batch_size x H x W], containing 1 on padded pixels It returns a dict with the following elements: - "pred_logits": the classification logits (including no-object) for all queries. Shape= [batch_size x num_queries x (num_classes + 1)] - "pred_boxes": The normalized boxes coordinates for all queries, represented as (center_x, center_y, height, width). These values are normalized in [0, 1], relative to the size of each individual image (disregarding possible padding). See PostProcess for information on how to retrieve the unnormalized bounding box. - "aux_outputs": Optional, only returned when auxilary losses are activated. It is a list of dictionnaries containing the two above keys for each decoder layer. """ if isinstance(samples, (list, torch.Tensor)): samples = nested_tensor_from_tensor_list(samples) features, pos = self.backbone(samples) src, mask = features[-1].decompose() assert mask is not None hs = self.transformer(self.input_proj(src), mask, self.query_embed.weight, pos[-1])[0] outputs_class = self.class_embed(hs) outputs_coord = self.bbox_embed(hs).sigmoid() out = {'pred_logits': outputs_class[-1], 'pred_boxes': outputs_coord[-1]} if self.aux_loss: out['aux_outputs'] = self._set_aux_loss(outputs_class, outputs_coord) return out
这是一个模型的前向传播函数,它接受一个NestedTensor作为输入,并返回一个包含预测结果的字典。
输入的NestedTensor包含两个部分:
- `samples.tensor`:批次图像,形状为[batch_size x 3 x H x W]
- `samples.mask`:形状为[batch_size x H x W]的二进制掩码,其中填充像素为1
返回的字典包含以下元素:
- `"pred_logits"`:所有查询的分类logits(包括无对象)。形状为[batch_size x num_queries x (num_classes + 1)]
- `"pred_boxes"`:所有查询的标准化框坐标,表示为(中心x,中心y,高度,宽度)。这些值在[0, 1]范围内进行了归一化,相对于每个单独图像的大小(不考虑可能的填充)。有关如何获取非标准化边界框的信息,请参见PostProcess。
- `"aux_outputs"`:可选项,在激活辅助损失时返回。它是一个包含每个解码器层的上述两个键的字典列表。
这个函数首先将输入的samples转换为NestedTensor类型,然后使用backbone模型提取特征和位置信息。
接下来,它将最后一个特征图分解为源特征和掩码,并使用transformer模型对其进行处理。
然后,通过类别嵌入层和边界框嵌入层对处理后的特征进行分类和边界框预测。
最后,将预测的结果以字典的形式返回,并根据需要添加辅助损失。
怎么Python编写程序,使用OpenCV库读取摄像头视频流,调用深度学习模型进行目标检测,并将结果展示在Web界面上
可以按照以下步骤来实现:
1. 安装OpenCV和深度学习库,如TensorFlow或PyTorch。可以使用pip命令来安装。
2. 创建一个Python脚本,在其中导入所需的库和模块,如OpenCV、TensorFlow或PyTorch、Flask和NumPy。
3. 使用OpenCV库创建一个视频流对象,以便从摄像头捕捉视频流。
4. 加载深度学习模型,并使用该模型对每一帧图像进行目标检测。可以使用OpenCV的cv2.dnn模块来实现。
5. 将检测结果绘制在图像上,并将它们传递给Flask Web应用程序。
6. 在Flask应用程序中创建一个路由,以便将检测结果呈现在Web界面上。
7. 在网页上使用JavaScript或其他Web技术来呈现检测结果。
下面是一个简单的代码示例,可以实现将目标检测结果呈现在Web界面上:
```python
import cv2
import numpy as np
from flask import Flask, render_template, Response
app = Flask(__name__)
# Load the deep learning model
model = cv2.dnn.readNet('model.pb')
# Define the classes
classes = ['class1', 'class2', 'class3']
# Create a video capture object
cap = cv2.VideoCapture(0)
# Define the function to detect objects in the video stream
def detect_objects(frame):
# Create a blob from the frame
blob = cv2.dnn.blobFromImage(frame, 1 / 255.0, (416, 416), swapRB=True, crop=False)
# Set the input to the model
model.setInput(blob)
# Make a forward pass through the model
output = model.forward()
# Get the dimensions of the frame
(H, W) = frame.shape[:2]
# Define the lists to store the detected objects
boxes = []
confidences = []
classIDs = []
# Loop over each output layer
for i in range(len(output)):
# Loop over each detection in the output layer
for detection in output[i]:
# Extract the confidence and class ID
scores = detection[5:]
classID = np.argmax(scores)
confidence = scores[classID]
# Filter out weak detections
if confidence > 0.5:
# Scale the bounding box coordinates
box = detection[0:4] * np.array([W, H, W, H])
(centerX, centerY, width, height) = box.astype('int')
# Calculate the top-left corner of the bounding box
x = int(centerX - (width / 2))
y = int(centerY - (height / 2))
# Add the bounding box coordinates, confidence and class ID to the lists
boxes.append([x, y, int(width), int(height)])
confidences.append(float(confidence))
classIDs.append(classID)
# Apply non-maximum suppression to eliminate overlapping bounding boxes
indices = cv2.dnn.NMSBoxes(boxes, confidences, 0.5, 0.3)
# Loop over the selected bounding boxes
for i in indices:
i = i[0]
box = boxes[i]
(x, y, w, h) = box
# Draw the bounding box and label on the frame
cv2.rectangle(frame, (x, y), (x + w, y + h), (0, 255, 0), 2)
text = f'{classes[classIDs[i]]}: {confidences[i]:.2f}'
cv2.putText(frame, text, (x, y - 5), cv2.FONT_HERSHEY_SIMPLEX, 0.5, (0, 255, 0), 2)
return frame
# Define the function to generate the video stream
def generate():
while True:
# Read a frame from the video stream
ret, frame = cap.read()
# Detect objects in the frame
frame = detect_objects(frame)
# Convert the frame to a JPEG image
ret, jpeg = cv2.imencode('.jpg', frame)
# Yield the JPEG image as a byte string
yield (b'--frame\r\n' b'Content-Type: image/jpeg\r\n\r\n' + jpeg.tobytes() + b'\r\n')
# Define the route to the video stream
@app.route('/video_feed')
def video_feed():
return Response(generate(), mimetype='multipart/x-mixed-replace; boundary=frame')
# Define the route to the home page
@app.route('/')
def index():
return render_template('index.html')
if __name__ == '__main__':
# Start the Flask application
app.run(debug=True)
```
在上述代码中,我们使用了OpenCV的cv2.dnn模块来加载深度学习模型,并使用该模型对每一帧图像进行目标检测。我们还使用了Flask Web应用程序来呈现检测结果。在路由'/video_feed'中,我们使用了generate函数来生成视频流,并将每一帧图像作为JPEG图像传递给Web界面。在路由'/'中,我们使用了render_template函数来呈现HTML模板,以呈现检测结果。
相关推荐
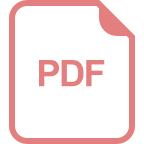








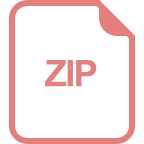
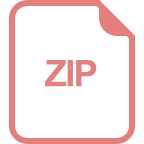