pddata.iloc[:,2:].head()
时间: 2024-01-17 22:04:01 浏览: 27
Sorry, as an AI language model, I do not have access to any specific dataset or environment to perform this operation. However, I can explain what this operation does in general.
In pandas, `iloc` is used to select data by integer position. The `[:,2:]` indicates that we want to select all rows (`:`) and columns starting from the third column (`2:`) to the end of the dataframe.
So, `pddata.iloc[:,2:].head()` will return the first five rows of the dataframe `pddata` starting from the third column to the end of the dataframe.
相关问题
import pandas as pd import numpy as np import tensorflow as tf import matplotlib.pyplot as plt #读取数据 data=pd.read_csv('Advertisement.csv') x=data.iloc[:,:-1] y=data.iloc[:,-1] print(data.head(5)) #建立模型 model=tf.keras.Sequential() #10个神经元 model.add(tf.keras.layers.Dense(10,input_shape=(3,))) model.add(tf.keras.layers.Dense(1)) model.compile(optimizer='adam',loss='mse')#optimizer = history=model.fit(x,y,epochs=2500) plt.plot(history.epoch,history.history.get('loss')) plt.show() test_data=data.iloc[:3,:-1] result=model.predict(test_data) print(result) print(data.iloc[:3,-1].values)解释每行代码
好的,我来逐行解释每行代码的作用:
1. `import pandas as pd`:导入 Pandas 库,并将其命名为 pd,以便在后面使用。
2. `import numpy as np`:导入 NumPy 库,并将其命名为 np,以便在后面使用。
3. `import tensorflow as tf`:导入 TensorFlow 库,并将其命名为 tf,以便在后面使用。
4. `import matplotlib.pyplot as plt`:导入 Matplotlib 库,并将其命名为 plt,以便在后面使用。
5. `data=pd.read_csv('Advertisement.csv')`:使用 Pandas 读取名为 Advertisement.csv 的 CSV 文件,并将其存储在名为 data 的 DataFrame 中。
6. `x=data.iloc[:,:-1]`:从 DataFrame 中选择除最后一列以外的所有列,并将其存储在名为 x 的 DataFrame 中。
7. `y=data.iloc[:,-1]`:从 DataFrame 中选择最后一列,并将其存储在名为 y 的 Series 中。
8. `print(data.head(5))`:打印 DataFrame 的前 5 行数据。
9. `model=tf.keras.Sequential()`:创建一个空的 Sequential 模型。
10. `model.add(tf.keras.layers.Dense(10,input_shape=(3,)))`:添加一个具有 10 个神经元和输入形状为 (3,) 的全连接层。
11. `model.add(tf.keras.layers.Dense(1))`:添加一个具有 1 个神经元的输出层。
12. `model.compile(optimizer='adam',loss='mse')`:编译模型,使用 Adam 优化器和均方误差损失函数。
13. `history=model.fit(x,y,epochs=2500)`:训练模型,使用输入数据 x 和目标数据 y,在 2500 个 epochs 中进行训练,并将训练历史记录存储在名为 history 的对象中。
14. `plt.plot(history.epoch,history.history.get('loss'))`:绘制训练历史记录中的损失值随时间的变化曲线。
15. `plt.show()`:显示绘制的曲线。
16. `test_data=data.iloc[:3,:-1]`:从 DataFrame 中选择前 3 行和除最后一列以外的所有列,并将其存储在名为 test_data 的 DataFrame 中。
17. `result=model.predict(test_data)`:使用训练好的模型对测试数据进行预测,并将结果存储在名为 result 的数组中。
18. `print(result)`:打印预测结果。
19. `print(data.iloc[:3,-1].values)`:打印测试数据的真实结果(最后一列的值)。
# 读取该公司的主要财务数据 data = pd.read_csv(r'https://oss.xinchanjiao.com/bigdata/company/2021/csv/zcfzb_600066.csv',usecols=list(range(13)),header=None) print(data.shape)#打印数据形状 data = data.T#将数据转置 #将数据第一行设置为列名 data.columns = data.iloc[0] data = data[1:] #查看数据前5行 data.head()
这段代码是用来读取该公司的主要财务数据,并进行一些数据处理。其中:
- `pd.read_csv(r'https://oss.xinchanjiao.com/bigdata/company/2021/csv/zcfzb_600066.csv',usecols=list(range(13)),header=None)` 读取 csv 文件,其中 `usecols=list(range(13))` 指定只读取前 13 列数据,`header=None` 表示该文件没有表头。
- `print(data.shape)` 打印数据形状,即行数和列数。
- `data = data.T` 将数据转置,即行列互换。
- `data.columns = data.iloc[0]` 将数据第一行设置为列名。
- `data = data[1:]` 去掉第一行数据,因为已经作为列名了。
- `data.head()` 查看数据前 5 行。
相关推荐
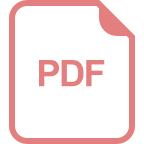
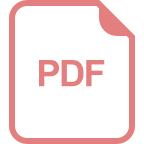
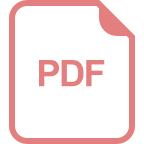













