num_samples = len(train_source_dataset.data) rgb_data = np.zeros((num_samples, 3, 28, 28), dtype=np.uint8) for i in range(num_samples): gray_image = train_source_dataset.data[i][0] rgb_image = np.stack([gray_image] * 3, axis=0) rgb_data[i] = rgb_image # 将数据集中的图像替换为 RGB 图像 train_source_dataset.data = rgb_data执行这段代码后报错ValueError: could not broadcast input array from shape (3,28) into shape (3,28,28)
时间: 2023-06-17 13:02:41 浏览: 46
这个错误是因为在执行代码时,可能出现了以下情况之一:
1. 数据集中的一些图像大小不是 28x28 像素,因此在将灰度图像转换为 RGB 图像时,图像的形状不正确。
2. 在将灰度图像转换为 RGB 图像时,可能没有正确地重复灰度通道以生成 RGB 图像。
为了解决这个问题,你可以尝试以下方法:
1. 检查数据集中的图像大小是否都是 28x28 像素。如果不是,你可以将它们缩放或裁剪到正确的大小。
2. 在将灰度图像转换为 RGB 图像时,确保正确地重复灰度通道以生成 RGB 图像。可以尝试使用以下代码:
```
rgb_image = np.repeat(gray_image[..., np.newaxis], 3, axis=-1)
```
这将在最后一个维度上添加一个新的维度,并将它重复 3 次以生成 RGB 图像。
如果你仍然无法解决问题,请提供更多代码和错误信息,以便更好地理解问题。
相关问题
怎么加快这段代码的运行速度num_samples = x_data.shape[0] features = tf.Variable(initial_value=tf.zeros((1, 0)), dtype=tf.float32) batch_size = 32 for i in range(0, num_samples, batch_size): batch = x_data[i:i + batch_size] batch = tf.expand_dims(batch, axis=0) if i + batch_size > num_samples: batch_num = num_samples - i if batch_num == 1: feature_batch = model.predict(batch.reshape(1, *input_shape), batch_size=batch_num) else: feature_batch = model.predict(batch, batch_size=batch_num) features = tf.concat([features, feature_batch], axis=1) else: feature_batch = model.predict(batch, batch_size=batch_size) features = tf.concat([features, feature_batch], axis=1) print(features.shape)
这段代码的运行速度可以通过以下几种方式进行优化:
1. 使用更快的模型:如果模型的计算速度较慢,可以尝试使用更快的模型,例如MobileNet、EfficientNet等。
2. 使用GPU加速:如果你的电脑有GPU,可以使用GPU加速模型的计算。可以使用`tf.config.experimental.set_memory_growth()`方法来设置GPU内存分配策略,以避免内存溢出问题。
3. 使用TensorFlow Dataset:TensorFlow Dataset是一种高效的数据输入管道,可以帮助提高训练速度。可以使用`tf.data.Dataset.from_tensor_slices()`方法来创建一个Dataset对象,然后使用`batch()`方法和`prefetch()`方法来设置批量大小和预取数据。
4. 使用更大的批量大小:如果你的电脑有足够的内存,可以尝试使用更大的批量大小,以提高训练速度。但是需要注意,批量大小过大可能会导致内存溢出问题。
5. 使用多线程预处理数据:如果你的电脑有多个CPU核心,可以使用多线程预处理数据,以加快数据处理速度。可以使用`tf.data.Dataset.map()`方法来定义一个数据预处理函数,并使用`num_parallel_calls`参数来设置线程数。
根据你的代码,可以使用方法1、2和4来进行优化,具体代码如下:
```python
# 方法1:使用更快的模型
from tensorflow.keras.applications import MobileNetV2
model = MobileNetV2(input_shape=input_shape, include_top=False, weights='imagenet')
# 方法2:使用GPU加速
gpus = tf.config.list_physical_devices('GPU')
if gpus:
try:
tf.config.experimental.set_memory_growth(gpus[0], True)
except RuntimeError as e:
print(e)
# 方法4:使用更大的批量大小
batch_size = 64
# 修改后的代码
features = tf.Variable(initial_value=tf.zeros((1, 0)), dtype=tf.float32)
for i in range(0, num_samples, batch_size):
batch = x_data[i:i + batch_size]
batch = tf.expand_dims(batch, axis=0)
if i + batch_size > num_samples:
batch_num = num_samples - i
if batch_num == 1:
feature_batch = model.predict(batch.reshape(1, *input_shape), batch_size=batch_num)
else:
feature_batch = model.predict(batch, batch_size=batch_num)
features = tf.concat([features, feature_batch], axis=1)
else:
feature_batch = model.predict(batch, batch_size=batch_size)
features = tf.concat([features, feature_batch], axis=1)
print(features.shape)
```
希望这些方法能够帮助你加快代码的运行速度!
在MNIST数据集中,每个图像都是28x28的灰度图像,如何修改train_source_dataset.data[i][0]使之成为RGB图像
### 回答1:
首先,MNIST数据集中的图像是单通道灰度图像,需要将其转换为三通道RGB图像。可以通过在每个像素位置复制该像素的值来实现这一点。具体来说,可以将每个灰度值复制三次,以便在每个通道中都具有相同的值。
下面是一种可以实现此转换的方法:
``` python
import numpy as np
# 加载 MNIST 数据集
train_source_dataset = ...
# 复制灰度值到三个通道
num_samples = len(train_source_dataset.data)
rgb_data = np.zeros((num_samples, 3, 28, 28), dtype=np.uint8)
for i in range(num_samples):
gray_image = train_source_dataset.data[i][0]
rgb_image = np.stack([gray_image] * 3, axis=0)
rgb_data[i] = rgb_image
# 将数据集中的图像替换为 RGB 图像
train_source_dataset.data = rgb_data
```
在这里,我们首先创建一个新的 numpy 数组 `rgb_data`,其形状为 `(num_samples, 3, 28, 28)`,其中 `num_samples` 是数据集中的样本数。然后,我们循环遍历每个样本,将其灰度图像复制到三个通道中,最终得到一个 RGB 图像。最后,我们将 `train_source_dataset.data` 替换为 RGB 图像数据。
### 回答2:
在MNIST数据集中,每个图像都是28x28的灰度图像,要将其修改为RGB图像,需要进行以下步骤:
1. 首先,需要将原始的灰度图像复制成一个新的RGB图像。新图像的尺寸仍然是28x28,但是每个像素点需要用3个数值来表示红、绿、蓝三个通道的颜色数值。
2. 针对每个像素点的灰度数值,可以将其赋值给新图像的红、绿、蓝三个通道的数值,由于都是灰度图像,三个通道的数值是相等的。
具体代码如下:
```python
import numpy as np
# 假设train_source_dataset是原始数据集对象
# 其中包含data属性用于存储图像数据,每一行是一个28x28的灰度图像
train_source_dataset = ...
# 创建新的RGB图像数据集对象
train_target_dataset = np.zeros((train_source_dataset.shape[0], 28, 28, 3))
# 针对每个图像,将灰度数值赋值给RGB通道
for i in range(train_source_dataset.shape[0]):
gray_image = train_source_dataset.data[i][0] # 获取图像的灰度数据
# 将每个像素点灰度数值赋值给RGB通道
for row in range(28):
for col in range(28):
train_target_dataset[i][row][col][0] = gray_image[row][col] # 红色通道
train_target_dataset[i][row][col][1] = gray_image[row][col] # 绿色通道
train_target_dataset[i][row][col][2] = gray_image[row][col] # 蓝色通道
```
经过以上操作,train_target_dataset就是一个新的RGB图像数据集,其中的每个图像都被修改为了28x28的RGB图像。
### 回答3:
在MNIST数据集中,每个图像都是原始的28x28的灰度图像。要将其修改为RGB图像,可以通过以下步骤进行处理:
1. 创建一个新的RGB图像数组,其大小为(28x28x3),其中28x28表示图像的宽度和高度,3表示每个像素点的三个通道(红色、绿色、蓝色)。
2. 将原始灰度图像的数据复制到新的RGB图像数组中的每个通道。此时,需要将原始灰度值复制到新数组的每个通道中,以使得每个通道的值相同。
3. 将修改后的RGB图像数组赋值给train_source_dataset.data[i][0],以替换原始的灰度图像数据。
下面是示例代码,实现将MNIST数据集的图像转换为RGB图像的功能:
```python
import numpy as np
# 假设原始灰度图像数据为train_source_dataset.data[i][0]
gray_image = train_source_dataset.data[i][0]
# 创建新的RGB图像数组
rgb_image = np.zeros((28, 28, 3), dtype=np.uint8)
# 将灰度值复制到RGB图像数组的每个通道
rgb_image[:, :, 0] = gray_image # 红色通道
rgb_image[:, :, 1] = gray_image # 绿色通道
rgb_image[:, :, 2] = gray_image # 蓝色通道
# 将修改后的RGB图像数组赋值给train_source_dataset.data[i][0]
train_source_dataset.data[i][0] = rgb_image
```
通过上述步骤,我们将原始的灰度图像修改为了RGB图像,其中每个像素点具有红色、绿色和蓝色三个通道的值。现在,train_source_dataset.data[i][0]中的图像已经是RGB图像了。
相关推荐
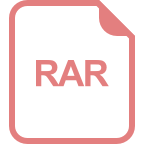
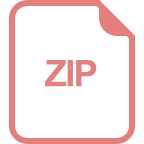












