The Value of Transposing Matrices in Data Analysis: Unearthing Hidden Patterns, Enhancing Analytical Efficiency
发布时间: 2024-09-13 21:50:03 阅读量: 28 订阅数: 26 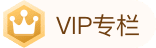
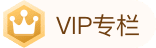
# The Value of Transpose Matrix in Data Analysis: Unearthing Hidden Patterns and Enhancing Analytical Efficiency
## 1. Overview of Transpose Matrix in Data Analysis
The transpose matrix is an important technique in data analysis that switches the rows and columns of a matrix, thus altering the shape and structure of the matrix. In data analysis, the transpose matrix has a wide range of applications, from data preprocessing to machine learning and data visualization.
The main role of the transpose matrix in data analysis is to transform data structures and extract data features. By transposing matrices, we can convert data from a row format to a column format, or vice versa. This is particularly useful in data preprocessing and feature engineering as it helps to adjust data to fit specific analysis or modeling tasks. Additionally, the transpose matrix can be used to extract data features such as maximum, minimum, and average values.
## 2. Theoretical Foundations of Transpose Matrix
### 2.1 Definition and Properties of Transpose Matrix
#### 2.1.1 Mathematical Definition of Transpose Matrix
The transpose matrix, also known as the transpose operator, is a linear operator that swaps the rows and columns of a matrix. For an m × n matrix A, its transpose matrix is denoted as A^T, and its elements are defined as follows:
```
A^T[i, j] = A[j, i]
```
Here, i and j represent the row and column indices of matrix A, respectively.
#### 2.1.2 Geometric Meaning of Transpose Matrix
The geometric meaning of the transpose matrix can be understood as a mirror image or flip of the matrix. For an m × n matrix A, its transpose matrix A^T has the following properties:
- **Row-Column Swap:** The row indices of A^T correspond to the column indices of A, and the column indices of A^T correspond to the row indices of A.
- **Symmetric Matrix:** If matrix A is symmetric (i.e., A = A^T), then its transpose matrix is identical to itself.
- **Orthogonal Matrix:** If matrix A is orthogonal (i.e., A^T A = I), then its transpose matrix is its inverse (i.e., A^T = A^-1).
### 2.2 Applications of Transpose Matrix in Data Analysis
The transpose matrix has a wide range of applications in data analysis, mainly reflected in two aspects: data structure transformation and data feature extraction.
#### 2.2.1 Data Structure Transformation
The transpose matrix can swap the rows and columns of data, thus changing the structure of the data. This is particularly useful in the following scenarios:
- **Data Format Conversion:** Convert data from wide format to long format, or vice versa.
- **Data Pivot:** Pivot the data across different dimensions, such as transforming grouped data by date into grouped data by product.
#### 2.2.2 Data Feature Extraction
The transpose matrix can swap data features (columns) and samples (rows), thereby extracting data feature information. This is particularly useful in the following scenarios:
- **Feature Selection:** Select the most representative features by calculating the correlation or importance between features.
- **Dimensionality Reduction:** Combine related features into new features to reduce the dimensionality of data while retaining important information.
## 3. Practical Applications of Transpose Matrix
### 3.1 Applications of Transpose Matrix in Data Preprocessing
#### 3.1.1 Data Format Conversion
In data analysis, it is often necessary to convert data from one format to another. For example, converting a wide table to a long table or vice versa. The transpose matrix can easily achieve this format conversion.
Suppose we have a wide table where each row represents a customer and each column represents an attribute. We can use the following code to convert the wide table into a long table:
```python
import numpy as np
# Create a wide table
wide_table = np.array([[1, 2, 3],
[4, 5, 6],
[7, 8, 9]])
# Transpose the wide table
long_table = wide_table.T
# Print the long table
print(long_table)
```
Output:
```
[[1 4 7]
[2 5 8]
[3 6 9]]
```
#### 3.1.2 Handling Missing Data
Missing values are a common issue in data analysis. The transpose matrix can help us handle missing values.
Suppose we have a data table with missing values. We can use the following code to replace missing values with averages:
```python
import numpy as np
# Create a data table with missing values
data_table = np.array([[1, 2, np.nan],
```
0
0
相关推荐
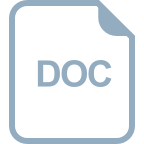
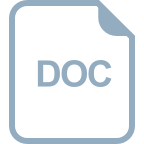
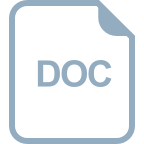
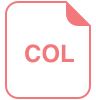
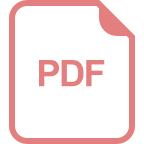
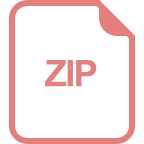
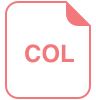
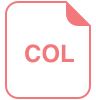
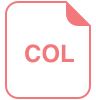
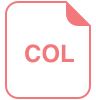