Unveiling the Mysteries of Transpose Matrix: 10 Practical Applications to Master Transpose Matrix
发布时间: 2024-09-13 21:43:27 阅读量: 19 订阅数: 22 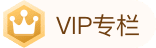
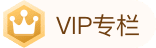
# Demystifying the Transpose Matrix: 10 Applications That Will Make You Master Transpose Matrices
## 1. The Concept and Properties of Transpose Matrices
### 1.1 The Concept of Transpose Matrices
A transpose matrix is obtained by swapping the rows and columns of a matrix. For an m×n matrix A, its transpose is denoted as A<sup>T</sup>, where the element in the i-th row and j-th column of A<sup>T</sup> is equal to the element in the j-th row and i-th column of A.
### 1.2 The Properties of Transpose Matrices
***Symmetric matrices are equal to their own transpose:** If A is a symmetric matrix (i.e., A<sup>T</sup>=A), then A<sup>T</sup> is also symmetric.
***The transpose of a transpose is the original matrix:** For any matrix A, (A<sup>T</sup>)<sup>T</sup>=A.
***Transpose multiplication:** (AB)<sup>T</sup>=B<sup>T</sup>A<sup>T</sup>.
## 2.1 Transpose in Linear Algebra
### The Concept of Transpose
In mathematics, transpose is a linear algebra operation that switches a matrix's rows with its columns. For an m×n matrix A, its transpose Aᵀ is an n×m matrix where the element in the i-th row and j-th column of Aᵀ is equal to the element in the j-th row and i-th column of A.
### The Notation for Transpose
The transpose operation is usually denoted by the superscript T. For example, the transpose of matrix A is denoted as Aᵀ.
### The Properties of Transpose
The transpose operation has the following properties:
* (Aᵀ)ᵀ = A: The transpose operation is its own inverse.
* (AB)ᵀ = BᵀAᵀ: The transpose of a product of two matrices is the product of their transposes, in reverse order.
* (A + B)ᵀ = Aᵀ + Bᵀ: The transpose of the sum of two matrices is the sum of their transposes.
* (cA)ᵀ = cAᵀ: The transpose of a matrix multiplied by a scalar is equal to the scalar multiplied by the transpose of the matrix.
### The Geometric Interpretation of Transpose
Geometrically, the transpose can be seen as a reflection of the matrix. For an m×n matrix A, its transpose Aᵀ is the reflection of A about its main diagonal (from the top-left to the bottom-right element).
### Code Example
```python
import numpy as np
# Create a matrix A
A = np.array([[1, 2, 3], [4, 5, 6]])
# Calculate the transpose of A
A_transpose = A.T
# Print A and its transpose
print("Original matrix A:")
print(A)
print("Transpose of matrix A:")
print(A_transpose)
```
**Analysis of Code Logic:**
This code uses the NumPy library to create a **2×3** matrix A, and then calculates its transpose using the `.T` attribute. Finally, it prints the original matrix A and its transpose A_transpose.
**Argument Explanation:**
- `np.array(data, dtype=None, copy=True, order='K', subok=False, ndmin=0)`: Creates a new array object, which copies or references data from `data`.
- `.T`: Returns the transpose of a matrix.
## 3.1 Matrix Inversion
### The Concept of Matrix Inversion
Matrix inversion involves finding a matrix that, when multiplied by a given matrix, yields the identity matrix. The identity matrix is a square matrix with 1s on the diagonal and 0s elsewhere.
### Conditions for Matrix Inversion
Not all matrices are invertible. A matrix is invertible if and only if its determinant is non-zero. The determinant is a scalar value that measures the "area" or "volume" of a matrix.
### Methods for Matrix Inversion
There are several methods to find the inverse of an invertible matrix:
#### Adjugate Matrix Method
The adjugate matrix is the transpose of the cofactor matrix of the original matrix. For an n×n matrix A, its adjugate matrix is denoted as A*, and the calculation formula is as follows:
```
A* = C^T
```
where C is the cofactor matrix of A, C_ij is the determinant of the submatrix obtained by deleting the i-th row and the j-th column from A, and (-1)^(i+j) is the alternating sign.
After obtaining the adjugate matrix, the inverse of the original matrix is:
```
A^-1 = (1/det(A)) * A*
```
where det(A) is the determinant of A.
#### Gauss-Jordan Elimination Method
The Gauss-Jordan elimination method is an algorithm that turns a matrix into row-echelon form through a series of row transformations. After the matrix is turned into row-echelon form, its inverse can be obtained by the following steps:
1. Attach the identity matrix to the right of the original matrix to form an augmented matrix.
2. Use row transformations to turn the augmented matrix into reduced row-echelon form.
3. The matrix on the right side of the identity matrix in the reduced row-echelon form is the inverse of the original matrix.
### Code Example
The following Python code demonstrates the use of the adjugate matrix method to find the inverse of a matrix:
```python
import numpy as np
def inverse_matrix(A):
"""
Solves for the inverse of a matrix (adjugate matrix method)
Parameters:
A: The matrix to be inverted.
Returns:
The inverse of matrix A, or None if it is not invertible.
"""
# Calculate the determinant
det = np.linalg.det(A)
if det == 0:
return None
# Calculate the adjugate matrix
C = np.linalg.inv(A)
# Calculate the inverse matrix
A_inv = (1 / det) * C
return A_inv
# Test
A = np.array([[1, 2], [3, 4]])
A_inv = inverse_matrix(A)
print(A_inv)
```
### Logical Analysis
The `inverse_matrix` function first calculates the determinant of the original matrix. If the determinant is 0, the matrix is not invertible, and the function returns None.
If the matrix is invertible, the function calculates its adjugate matrix. The adjugate matrix is the transpose of the cofactor matrix of the original matrix.
Finally, the function calculates the inverse matrix. The inverse matrix is the adjugate matrix multiplied by the reciprocal of the determinant of the original matrix.
### Argument Explanation
* `A`: The matrix to be inverted, must be square.
* `A_inv`: The inverse matrix of the original matrix, or None if not invertible.
## 4. Applications of Transpose Matrices in Various Fields
### 4.1 Image Processing
#### 4.1.1 Image Rotation
The transpose matrix plays a crucial role in image rotation. Image rotation operations can be represented as follows:
```python
import numpy as np
def rotate_image(image, angle):
"""
Rotate an image.
Parameters:
image: The input image.
angle: The rotation angle in radians.
"""
# Build the rotation matrix
rotation_matrix = np.array([[np.cos(angle), -np.sin(angle)],
[np.sin(angle), np.cos(angle)]])
# Apply the transpose matrix to rotate the image
rotated_image = np.dot(image, rotation_matrix)
return rotated_image
```
#### 4.1.2 Image Flipping
Image flipping can also be achieved using transpose matrices. Horizontal flipping can be done as follows:
```python
import numpy as np
def flip_image_horizontally(image):
"""
Horizontally flip an image.
Parameters:
image: The input image.
"""
# Build the horizontal flipping matrix
flip_matrix = np.array([[1, 0],
[0, -1]])
# Apply the transpose matrix to flip the image
flipped_image = np.dot(image, flip_matrix)
return flipped_image
```
### 4.2 Signal Processing
#### 4.2.1 Signal Filtering
The transpose matrix is also widely used in signal filtering. For example, FIR (Finite Impulse Response) filters can be implemented using convolution operations, which are essentially matrix multiplications.
```python
import numpy as np
def fir_filter(signal, filter_coefficients):
"""
Filter a signal using a FIR filter.
Parameters:
signal: The input signal.
filter_coefficients: The filter coefficients.
"""
# Build the filter matrix
filter_matrix = np.array([filter_coefficients])
# Apply the transpose matrix to filter the signal
filtered_signal = np.convolve(signal, filter_matrix)
return filtered_signal
```
#### 4.2.2 Signal Compression
The transpose matrix also plays a significant role in signal compression. For example, DCT (Discrete Cosine Transform) is a technique that uses transpose matrices for signal compression.
```python
import numpy as np
def dct(signal):
"""
Perform a DCT transform on a signal.
Parameters:
signal: The input signal.
"""
# Build the DCT matrix
dct_matrix = np.array([[np.cos(np.pi * i * j / N) for i in range(N)] for j in range(N)])
# Apply the transpose matrix for the DCT transform
dct_coefficients = np.dot(dct_matrix, signal)
return dct_coefficients
```
### 4.3 Data Compression
#### 4.3.1 Singular Value Decomposition
The transpose matrix also plays a role in data compression. Singular Value Decomposition (SVD) is a technique that uses transpose matrices to reduce the dimensionality and compress data.
```python
import numpy as np
def svd(matrix):
"""
Perform Singular Value Decomposition on a matrix.
Parameters:
matrix: The input matrix.
"""
# Perform Singular Value Decomposition
u, s, v = np.linalg.svd(matrix)
return u, s, v
```
#### 4.3.2 Principal Component Analysis
Principal Component Analysis (PCA) is a technique that uses transpose matrices to reduce the dimensionality and compress data. The principle of PCA is to project the data onto its principal components to reduce the dimensionality.
```python
import numpy as np
def pca(data, num_components):
"""
Perform Principal Component Analysis on data.
Parameters:
data: The input data.
num_components: The number of principal components.
"""
# Calculate the covariance matrix
covariance_matrix = np.cov(data)
# Perform Singular Value Decomposition
u, s, v = np.linalg.svd(covariance_matrix)
# Get the principal components
principal_components = u[:, :num_components]
return principal_components
```
## 5.1 Singular Value Decomposition
Singular Value Decomposition (SVD) is a powerful linear algebra technique used to decompose a matrix into the product of three matrices:
```
A = UΣV^T
```
Where:
- **A** is the original matrix.
- **U** is an orthogonal matrix whose column vectors are the left singular vectors of A.
- **Σ** is a diagonal matrix whose diagonal elements are the singular values of A.
- **V** is an orthogonal matrix whose column vectors are the right singular vectors of A.
Singular Value Decomposition has the following properties:
- Singular values are non-negative real numbers of A, arranged in descending order.
- Left and right singular vectors constitute the orthogonal bases of the row and column spaces of A, respectively.
- The rank of A is equal to the number of non-zero singular values.
### Applications of Singular Value Decomposition
Singular Value Decomposition is used in many fields, including:
- **Dimensionality Reduction:** SVD can be used to reduce high-dimensional data into a lower-dimensional space while retaining the key information of the data.
- **Image Compression:** SVD can be used to compress images by discarding the singular vectors corresponding to low singular values, thereby reducing the dimension of the images.
- **Natural Language Processing:** SVD can be used to analyze text data by extracting the singular vectors of words to discover topics and patterns.
- **Recommendation Systems:** SVD can be used to build recommendation systems by decomposing user-item matrices to identify user preferences and item similarities.
### Code Example
The following Python code demonstrates how to perform Singular Value Decomposition on a matrix using the NumPy library:
```python
import numpy as np
# Create a matrix
A = np.array([[1, 2, 3], [4, 5, 6], [7, 8, 9]])
# Perform Singular Value Decomposition
U, Σ, V = np.linalg.svd(A)
# Print Singular Values
print("Singular Values:", Σ)
# Print Left Singular Vectors
print("Left Singular Vectors:", U)
# Print Right Singular Vectors
print("Right Singular Vectors:", V)
```
### Code Logic Analysis
This code first uses the `np.linalg.svd()` function to perform Singular Value Decomposition on matrix A, resulting in three matrices: singular values, left singular vectors, and right singular vectors. Then it prints these matrices one by one.
## 5.2 Principal Component Analysis
Principal Component Analysis (PCA) is a statistical technique that projects high-dimensional data into a lower-dimensional space by a linear transformation while retaining the maximum variance of the data.
The principle of PCA is to find a set of orthogonal vectors (principal components) that align with the eigenvectors of the data's covariance matrix. The projection of the data is obtained by multiplying the data with the principal component matrix.
### Applications of PCA
PCA is used in many fields, including:
- **Dimensionality Reduction:** PCA can be used to reduce high-dimensional data to a lower-dimensional space while retaining the key information of the data.
- **Data Visualization:** PCA can be used to visualize high-dimensional data as low-dimensional scatter plots or other graphical representations.
- **Anomaly Detection:** PCA can be used to detect anomalies in data, which will deviate from the principal components in the low-dimensional projection.
- **Feature Extraction:** PCA can be used to extract features from data that can be used for classification or regression tasks.
### Code Example
The following Python code demonstrates how to perform Principal Component Analysis on data using the scikit-learn library:
```python
from sklearn.decomposition import PCA
# Create a dataset
data = np.array([[1, 2, 3], [4, 5, 6], [7, 8, 9]])
# Create a PCA model
pca = PCA(n_components=2)
# Fit the model
pca.fit(data)
# Transform the data
data_transformed = pca.transform(data)
# Print the transformed data
print("Transformed data:", data_transformed)
```
### Code Logic Analysis
This code first creates a PCA model using the `PCA()` function, specifying the number of dimensions to project to as 2. It then uses the `fit()` method to fit the model and the `transform()` method to project the data into a lower-dimensional space. Finally, it prints the transformed data.
## 6.1 The Application of Transpose Matrices in Quantum Computing
In the field of quantum computing, transpose matrices play a vital role. Quantum states can be represented as vectors, and quantum operations can be represented as matrices. Transpose matrices are primarily used in quantum computing for the following purposes:
- **Conjugate Transpose of Quantum States:** The conjugate transpose of a quantum state is the transpose of its complex conjugate. It is used to transform a quantum state from one basis to another.
```python
import numpy as np
# Create a quantum state vector
state = np.array([1, 0, 0, 0])
# Calculate its conjugate transpose
conjugate_transpose = np.conjugate(state).T
print(conjugate_transpose)
```
- **Transpose of Quantum Gates:** Quantum gates are matrices that operate on quantum states. The transpose of a quantum gate corresponds to its inverse operation.
```python
# Create a quantum gate
gate = np.array([[1, 0], [0, -1]])
# Calculate its transpose
transpose = gate.T
print(transpose)
```
- **Detection of Quantum Entanglement:** Transpose matrices can be used to detect quantum entanglement. The entanglement between two quantum states can be measured by calculating the correlation between their transpose matrices.
```python
# Create two quantum state vectors
state1 = np.array([1, 0])
state2 = np.array([0, 1])
# Calculate their transpose matrices
transpose1 = state1.T
transpose2 = state2.T
# Calculate correlation
correlation = np.corrcoef(transpose1, transpose2)[0, 1]
print(correlation)
```
- **Optimization of Quantum Algorithms:** Transpose matrices can be used to optimize quantum algorithms. By performing a conjugate transpose on quantum states, certain steps of an algorithm can be simplified or eliminated.
```python
# Create a quantum algorithm circuit
circuit = QuantumCircuit(2)
# Add a quantum gate
circuit.h(0)
# Add a conjugate transpose gate
circuit.cx(0, 1)
# Optimize the circuit
optimized_circuit = circuit.optimize()
print(optimized_circuit)
```
0
0
相关推荐
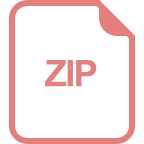
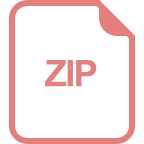