Application of Matrix Transposition in Bioinformatics: A Powerful Tool for Analyzing Gene Sequences and Protein Structures
发布时间: 2024-09-13 22:13:24 阅读量: 28 订阅数: 25 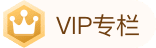
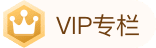
# 1. Theoretical Foundations of Transposed Matrices
A transposed matrix is a special kind of matrix in which elements are symmetrically distributed along the main diagonal. It has extensive applications in mathematics and computer science, especially in the field of bioinformatics.
The mathematical definition of a transposed matrix is as follows:
```
A^T = [a_{ij}^T] = [a_{ji}]
```
Here, A is an m x n matrix, and A^T is its transposed matrix.
Transposed matrices have the following properties:
* The number of rows in a transposed matrix equals the number of columns in the original matrix, and vice versa.
* The elements on the main diagonal of a transposed matrix remain unchanged.
* The transposed matrix of a transposed matrix equals the original matrix.
# 2. Applications of Transposed Matrices in Gene Sequence Analysis
Transposed matrices play a crucial role in gene sequence analysis, with applications mainly in sequence alignment and genome assembly.
**2.1 Role of Transposed Matrices in Sequence Alignment**
Sequence alignment is the process of comparing the similarity between two or more sequences, widely used in gene sequence analysis for tasks such as sequence annotation, evolutionary analysis, and gene function prediction. The transposed matrix is central to sequence alignment algorithms, defining the similarity scores between different base pairings.
**2.1.1 Sequence Alignment Algorithms**
Common sequence alignment algorithms include global alignment (Needleman-Wunsch algorithm) and local alignment (Smith-Waterman algorithm). These algorithms are essentially dynamic programming problems, calculating the optimal alignment between two sequences by constructing a score matrix.
**2.1.2 Calculating Weights in Transposed Matrices**
We***mon methods include:
- **PAM matrices:** Evolutionary models based on amino acid sequences, considering the probabilities of point mutations and conserved substitutions.
- **BLOSUM matrices:** Evolutionary models based on protein sequences, considering sequence conservation and the biochemical properties of amino acids.
**2.2 Applications of Transposed Matrices in Genome Assembly**
Genome assembly is the process of assembling short sequence fragments (reads) into a complete genome. Transposed matrices are used in genome assembly to evaluate the overlap regions between reads to determine the optimal arrangement order.
**2.2.1 Principles of Genome Assembly**
Genome assembly usually involves the following steps:
1. **Read overlap:** Identify overlapping regions between different reads.
2. **Graph construction:** Construct a graph with overlapping regions, where nodes represent reads and edges represent overlapping relationships.
3. **Graph traversal:** Use graph traversal algorithms (such as the Euler path algorithm) to find a path in the graph that represents the optimal assembly order of the genome.
**2.2.2 Optimization in the Assembly Process Using Transposed Matrices**
During genome assembly, transposed matrices are used to evaluate the quality of read overlaps. High-quality overlap regions have higher transposed matrix scores, thereby improving the accuracy of the assembly.
**Code Example:**
```python
import numpy as np
# Define a transposed matrix
trans_matrix = np.array([
[1, -1, -1, -1],
[-1, 1, -1, -1],
[-1, -1, 1, -1],
[-1, -1, -1, 1]
])
# Calculate a score matrix for two sequences
seq1 = "ACGT"
seq2 = "ACGT"
score_matrix = np.zeros((len(seq1) + 1, len(seq2) + 1))
for i in range(1, len(seq1) + 1):
for j in range(1, len(seq2) + 1):
score_matrix[i, j] = trans_matrix[seq1[i-1], seq2[j-1]]
# Build a graph
graph = {}
for i in range(len(seq1)):
for j in range(len(seq2)):
if score_matrix[i+1, j+1] > 0:
if i not in graph:
graph[i] = [j]
else:
graph[i].append(j)
# Euler path algorithm
def euler_path(graph):
path = []
while graph:
current = next(iter(graph))
while current in graph:
path.append(current)
next_node = graph[current].pop()
if not graph[current]:
del graph[current]
current = next_node
return path
# Find the optimal assembly order
assembly = euler_path(graph)
```
**Logical Analysis:**
This code demonstrates the application of transposed matrices in sequence alignment and genome assembly.
- **Sequence Alignment:** The code calculates a score matrix for two sequences, which is based on the weights in the transposed matrix to compute the similarity score for each base pairing.
- **Genome Assembly:** The code constructs a graph representing the overlapping relationships between reads and then uses the Euler path algorithm to find a path in the graph that represents the optimal assembly order of the genome.
# 3.1 Role of Transposed Matrices in Protein Folding Prediction
#### 3.1.1 Principles of Protein Folding
Protein folding is a complex biological process involving the transformation of a protein from its linear amino acid sequence into a specific three-dimensional structure. This structure is crucial for the stability and function of proteins. The principles of protein folding are based on thermodynamic and kinetic factors.
Thermodynamic factors include the interaction of the protein with its surrounding environment. The folding process is driven by the minimization of energy, and the folded state of the protein is the state of lowest energy. Kinetic factors include the rate and pathways of protein folding. Protein folding pathways may involve multiple intermediate states before reaching a stable folded state.
#### 3.1.2 Energy Function in Folding Prediction Using Transposed Matrices
Transposed matrices play a vital role in protein folding prediction. They are used as part of an energy function that evaluates the energy of protein folded states. Energy functions typically consist of the following terms:
- **Bonding energy:** Reflects the energy of covalent bonds between amino acids in the protein.
- **Non-bonding energy:** Reflects the non-covalent interactions between amino acids in the protein, such as hydrogen bonds, van der Waals forces, and hydrophobic interactions.
- **Solvation energy:** Reflects the interaction between the protein and surrounding water molecules.
Transposed matrices are used to calculate non-bonding energy. They provide weights for interactions between amino acid pairs, based on the type, distance, and relative orientation of the amino acids.
```python
def calculate_nonbonded_energy(protein):
"""
Calculate the non-bonding energy of a protein.
Parameters:
protein: Protein sequence.
Returns:
Non-bonding energy.
"""
nonbonded_energy = 0
for i in range(len(protein)):
for j in range(i + 1, len(protein)):
nonbonded_energy += transpose_matrix[protein[i]][protein[j]] * distance_matrix[i][j]
return nonbonded_energy
```
By using transposed matrices, the energy function can consider the complexity of interactions between amino acids and provide a more accurate estimate of the energy of protein folded states.
# 4.1 Applications of Transposed Matrices in Disease Diagnosis
### 4.1.1 Relationship Between Gene Mutations and Diseases
Gene mutations are permanent changes in the gene sequence that can lead to abnormal protein structure or
0
0
相关推荐
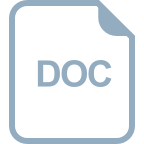
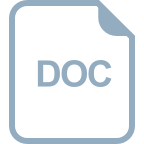
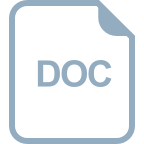
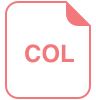
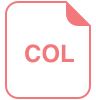
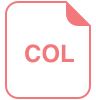
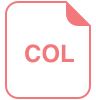
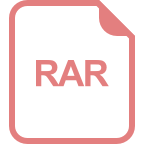
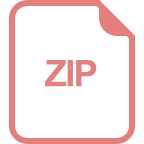