module 'torch.nn' has no attribute 'MAELoss'
时间: 2023-09-08 22:11:21 浏览: 166
对不起,我之前提到的"torch.nn.MAELoss"是一个错误的信息。在PyTorch中,没有直接提供平均绝对误差(Mean Absolute Error,MAE)的损失函数。但是,你可以使用均方误差(Mean Squared Error,MSE)作为代替,然后将其结果开根号以获得类似于MAE的效果。
以下是如何使用MSE损失函数计算MAE的近似值:
```python
import torch
import torch.nn as nn
import torch.optim as optim
# 定义模型
model = YourModel()
criterion = nn.MSELoss() # 使用均方误差损失函数
# 定义优化器
optimizer = optim.Adam(model.parameters(), lr=0.001)
# 计算MAE的近似值
def mean_absolute_error(y_true, y_pred):
return torch.sqrt(criterion(y_true, y_pred))
# 在训练循环中使用
loss = criterion(outputs, labels) # 计算均方误差
mae = mean_absolute_error(outputs, labels) # 计算MAE的近似值
```
通过将MSE损失函数与平方根操作结合起来,我们可以获得类似于MAE的效果。请注意,这只是一个近似值,并不等同于使用真正的MAE损失函数。
相关问题
module torch.nn has no attribute BatchNormld
这个错误可能是由于拼写错误导致的。在 PyTorch 中,Batch Normalization 的正确拼写是 BatchNorm2d 而不是 BatchNormld。请检查你的代码,确保正确地引入和使用 BatchNorm2d。以下是一个示例:
```python
import torch
import torch.nn as nn
# 创建一个包含Batch Normalization的模型
model = nn.Sequential(
nn.Conv2d(3, 64, kernel_size=3, stride=1, padding=1),
nn.BatchNorm2d(64),
nn.ReLU(),
...
)
# 使用模型进行前向传播
input = torch.randn(1, 3, 224, 224)
output = model(input)
```
请注意,上述示例中的 "..." 表示模型中的其他层。确保在创建模型时按照正确的顺序添加 BatchNorm2d 层,并传递正确的参数。如果问题仍然存在,请提供更多的代码和错误信息以便我能够更好地帮助你。
AttributeError: module torch.nn has no attribute KMeans
这个错误的原因是torch.nn模块中没有名为KMeans的属性,因此无法调用。KMeans通常是用于聚类算法的库,你可能需要使用其他第三方库来执行聚类操作,例如scikit-learn。你可以尝试导入scikit-learn库并使用它的KMeans方法来解决这个问题。具体操作方法可以参考scikit-learn的官方文档。
相关推荐
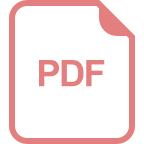
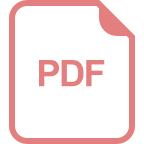
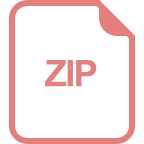












