model = PhysicsInformedNN(X_u_train, u_train, X_f_train, layers, lb, ub, nu)
时间: 2023-10-07 20:06:35 浏览: 123
这行代码看起来是在定义一个物理知识指导下的神经网络模型,其中:
- `X_u_train` 是输入数据的训练集,用于指导模型学习物理规律;
- `u_train` 是输入数据对应的输出数据的训练集,也用于指导模型学习物理规律;
- `X_f_train` 是用于训练神经网络的额外的无标签数据集,用于帮助模型理解物理规律;
- `layers` 是一个列表,表示神经网络的层数和每层的神经元数;
- `lb` 和 `ub` 分别是输入数据的下界和上界,用于对输入数据进行归一化;
- `nu` 是流体的粘度。
在这个模型中,我们采用了物理知识来指导神经网络的学习,通过加入额外的无标签数据集,使得神经网络能够更好地理解物理规律,从而提高模型的精度和鲁棒性。
相关问题
net = trainNetwork(X_train', Y_train', layers, options)
这段代码是用MATLAB中的Deep Learning Toolbox进行神经网络的训练。其中,X_train和Y_train分别是训练集的输入特征和标签,layers是神经网络的层结构,options是训练选项。trainNetwork函数会根据训练集和网络结构,以及训练选项对神经网络进行训练,并返回训练好的网络模型net。
model.add(LSTM(units=128, input_shape=(X_train.shape[-2:])))给这段代码加一个注意力机制
可以通过添加一个Attention层来实现注意力机制,代码如下:
```
from keras.layers import Layer, Input, Dense, LSTM, Attention, Concatenate
from keras.models import Model
# 定义Attention层
class AttentionLayer(Layer):
def __init__(self, **kwargs):
super(AttentionLayer, self).__init__(**kwargs)
def build(self, input_shape):
self.W = self.add_weight(name="att_weight", shape=(input_shape[-1], 1), initializer="normal")
self.b = self.add_weight(name="att_bias", shape=(input_shape[1], 1), initializer="zeros")
super(AttentionLayer, self).build(input_shape)
def call(self, x):
e = K.tanh(K.dot(x, self.W) + self.b)
a = K.softmax(e, axis=1)
output = x * a
return K.sum(output, axis=1)
def compute_output_shape(self, input_shape):
return (input_shape[0], input_shape[-1])
# 定义模型
inputs = Input(shape=(X_train.shape[-2], X_train.shape[-1]))
lstm = LSTM(units=128, return_sequences=True)(inputs)
attention = AttentionLayer()(lstm)
outputs = Dense(1, activation='sigmoid')(attention)
model = Model(inputs=inputs, outputs=outputs)
```
这里定义了一个Attention层,将LSTM的输出作为输入,然后计算每个时间步的权重,最后将加权后的输出进行求和得到Attention结果,最终通过Dense层输出。需要注意的是,Attention层的输入为三维张量,因此需要将LSTM的return_sequences参数设置为True。
在模型训练时,需要将模型的损失函数设置为binary_crossentropy,代码如下:
```
model.compile(loss='binary_crossentropy', optimizer='adam', metrics=['accuracy'])
model.fit(X_train, y_train, epochs=10, batch_size=64, validation_data=(X_test, y_test))
```
相关推荐
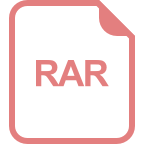
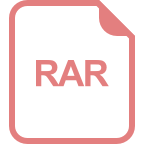
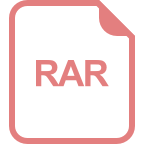













