d2l.plt.scatter()是什么函数
时间: 2023-07-09 12:31:17 浏览: 123
d2l.plt.scatter() 是 Deep Learning - The Straight Dope (D2L) 书中定义的一个函数,用于绘制散点图。它是基于 Matplotlib 库实现的,具有以下特点:
- 支持自定义点的颜色、大小、形状等属性。
- 支持添加标题、坐标轴标签等注释。
- 支持同时绘制多个散点图。
该函数的定义如下:
```python
def set_figsize(figsize=(3.5, 2.5)):
"""Set matplotlib figure size."""
use_svg_display()
d2l.plt.rcParams['figure.figsize'] = figsize
def use_svg_display():
"""Use svg format to display plot in jupyter."""
d2l.plt.rcParams['svg.fonttype'] = 'none'
def plot(X, Y=None, xlabel=None, ylabel=None, legend=None, xlim=None,
ylim=None, xscale='linear', yscale='linear', fmts=None,
figsize=(3.5, 2.5), axes=None):
"""Plot multiple lines."""
if legend is None:
legend = []
set_figsize(figsize)
axes = axes if axes else d2l.plt.gca()
# Return True if X (tensor or list) has more than 1 dimension
def has_one_dim(X):
if isinstance(X, np.ndarray) or isinstance(X, list):
return len(X.shape) == 1
else:
return len(X.size()) == 1
# Convert Y to list so that len(Y) is always defined
if Y is None:
Y = []
if not isinstance(Y, list):
Y = [Y]
if not isinstance(X, list):
X = [X] * len(Y)
if len(X) != len(Y):
X = X * len(Y)
# Format lines and points
if fmts is None:
fmts = ['-'] * len(X)
elif isinstance(fmts, str):
fmts = [fmts] * len(X)
# Plot
for x, y, fmt in zip(X, Y, fmts):
if has_one_dim(x):
x = np.arange(len(y)) + 1
axes.plot(x, y, fmt)
axes.set_xlabel(xlabel)
axes.set_ylabel(ylabel)
axes.set_xscale(xscale)
axes.set_yscale(yscale)
if xlim:
axes.set_xlim(xlim)
if ylim:
axes.set_ylim(ylim)
if legend:
axes.legend(legend)
axes.grid()
d2l.plt.show()
def show_images(imgs, num_rows, num_cols, scale=2):
"""Plot a list of images."""
figsize = (num_cols * scale, num_rows * scale)
_, axes = d2l.plt.subplots(num_rows, num_cols, figsize=figsize)
for i in range(num_rows):
for j in range(num_cols):
axes[i][j].imshow(imgs[i * num_cols + j])
axes[i][j].axes.get_xaxis().set_visible(False)
axes[i][j].axes.get_yaxis().set_visible(False)
return axes
def plot_learning_curves(train_acc, test_acc, train_loss, test_loss):
"""Plot the learning curves."""
d2l.plt.plot(train_acc, linestyle='-', color='blue', label='train accuracy')
d2l.plt.plot(test_acc, linestyle='--', color='red', label='test accuracy')
d2l.plt.xlabel('epochs')
d2l.plt.ylabel('accuracy')
d2l.plt.legend(loc='upper right')
d2l.plt.twinx()
d2l.plt.plot(train_loss, linestyle='-', color='green', label='train loss')
d2l.plt.plot(test_loss, linestyle='--', color='orange', label='test loss')
d2l.plt.ylabel('loss')
d2l.plt.legend(loc='upper left')
d2l.plt.show()
def use_svg_display():
"""Use svg format to display plot in jupyter."""
d2l.plt.rcParams['svg.fonttype'] = 'none'
def set_figsize(figsize=(3.5, 2.5)):
"""Set matplotlib figure size."""
use_svg_display()
d2l.plt.rcParams['figure.figsize'] = figsize
def plot(X, Y=None, xlabel=None, ylabel=None, legend=None, xlim=None,
ylim=None, xscale='linear', yscale='linear', fmts=None,
figsize=(3.5, 2.5), axes=None):
"""Plot multiple lines."""
if legend is None:
legend = []
set_figsize(figsize)
axes = axes if axes else d2l.plt.gca()
# Return True if X (tensor or list) has more than 1 dimension
def has_one_dim(X):
if isinstance(X, np.ndarray) or isinstance(X, list):
return len(X.shape) == 1
else:
return len(X.size()) == 1
# Convert Y to list so that len(Y) is always defined
if Y is None:
Y = []
if not isinstance(Y, list):
Y = [Y]
if not isinstance(X, list):
X = [X] * len(Y)
if len(X) != len(Y):
X = X * len(Y)
# Format lines and points
if fmts is None:
fmts = ['-'] * len(X)
elif isinstance(fmts, str):
fmts = [fmts] * len(X)
# Plot
for x, y, fmt in zip(X, Y, fmts):
if has_one_dim(x):
x = np.arange(len(y)) + 1
axes.plot(x, y, fmt)
axes.set_xlabel(xlabel)
axes.set_ylabel(ylabel)
axes.set_xscale(xscale)
axes.set_yscale(yscale)
if xlim:
axes.set_xlim(xlim)
if ylim:
axes.set_ylim(ylim)
if legend:
axes.legend(legend)
axes.grid()
d2l.plt.show()
def show_images(imgs, num_rows, num_cols, scale=2):
"""Plot a list of images."""
figsize = (num_cols * scale, num_rows * scale)
_, axes = d2l.plt.subplots(num_rows, num_cols, figsize=figsize)
for i in range(num_rows):
for j in range(num_cols):
axes[i][j].imshow(imgs[i * num_cols + j])
axes[i][j].axes.get_xaxis().set_visible(False)
axes[i][j].axes.get_yaxis().set_visible(False)
return axes
def plot_learning_curves(train_acc, test_acc, train_loss, test_loss):
"""Plot the learning curves."""
d2l.plt.plot(train_acc, linestyle='-', color='blue', label='train accuracy')
d2l.plt.plot(test_acc, linestyle='--', color='red', label='test accuracy')
d2l.plt.xlabel('epochs')
d2l.plt.ylabel('accuracy')
d2l.plt.legend(loc='upper right')
d2l.plt.twinx()
d2l.plt.plot(train_loss, linestyle='-', color='green', label='train loss')
d2l.plt.plot(test_loss, linestyle='--', color='orange', label='test loss')
d2l.plt.ylabel('loss')
d2l.plt.legend(loc='upper left')
d2l.plt.show()
def set_axes(axes, xlabel, ylabel, xlim, ylim, xscale, yscale, legend):
"""Set the axes for matplotlib."""
axes.set_xlabel(xlabel)
axes.set_ylabel(ylabel)
axes.set_xscale(xscale)
axes.set_yscale(yscale)
if xlim:
axes.set_xlim(xlim)
if ylim:
axes.set_ylim(ylim)
if legend:
axes.legend(legend)
axes.grid()
相关推荐
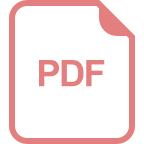
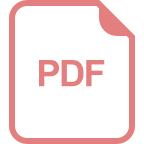














