n_locations = len(locations) n_goals = 0 n_successes = 0 i = n_locations distance_traveled = 0 start_time = rospy.Time.now() running_time = 0 location = "" last_location = ""
时间: 2024-02-14 14:19:54 浏览: 19
这段代码中定义了一些变量,具体含义如下:
- `n_locations`: `locations`中地点的个数。
- `n_goals`: 发送给导航节点的目标点个数,初始化为0。
- `n_successes`: 到达目标点的成功次数,初始化为0。
- `i`: 待前往的目标点在`locations`列表中的索引,初始化为`n_locations`。
- `distance_traveled`: 机器人已经行驶的距离,初始化为0。
- `start_time`: 导航任务开始时间,初始化为当前时间。
- `running_time`: 导航任务运行时间,初始化为0。
- `location`: 当前待前往的目标点名称,初始化为空字符串。
- `last_location`: 上一个到达的目标点名称,初始化为空字符串。
这些变量将在后续的导航任务中被使用,用于记录导航任务的状态和统计导航结果。
相关问题
self.labels = [] self.person = [] self.face_locations = [] self.face_encodings = [] self.face_names = [] self.process_this_frame = True
这是一段代码片段,看起来是在使用某个人脸识别库或者机器学习模型进行人脸识别。其中的变量含义如下:
- `self.labels`:存储人脸标签的列表。
- `self.person`:存储人脸名字的列表。
- `self.face_locations`:存储人脸位置的列表。
- `self.face_encodings`:存储人脸编码的列表。
- `self.face_names`:存储人脸名字的列表。
- `self.process_this_frame`:一个布尔值,表示当前帧是否需要进行处理。如果为True,则进行人脸识别处理,否则跳过。
self.video_capture = cv2.VideoCapture(0) self.no_video = False self.labels = [] self.person = [] self.face_locations = [] self.face_encodings = [] self.face_names = [] self.process_this_frame = True
这段代码使用了OpenCV库中的VideoCapture类初始化了一个摄像头对象。属性no_video为False表示正在使用视频流。labels、person、face_locations、face_encodings和face_names是用来存储人脸识别所需的信息。process_this_frame为True表示处理当前帧。
相关推荐
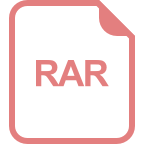
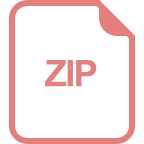












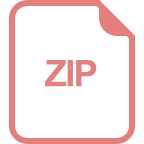