用乌鸦搜索算法优化随机森林模型python代码
时间: 2023-11-13 16:59:16 浏览: 41
乌鸦搜索算法是一种基于鸟群行为模拟的优化算法,可以用于优化随机森林模型的参数选择。以下是使用Python实现的示例代码:
``` python
import numpy as np
from sklearn.ensemble import RandomForestClassifier
from crowsearch import CrowSearchAlgorithm
# 定义适应度函数
def fitness_function(X):
n_estimators = int(X[0])
max_depth = int(X[1])
min_samples_split = int(X[2])
min_samples_leaf = int(X[3])
clf = RandomForestClassifier(n_estimators=n_estimators, max_depth=max_depth,
min_samples_split=min_samples_split, min_samples_leaf=min_samples_leaf)
clf.fit(X_train, y_train)
return -clf.score(X_val, y_val) # 目标是最小化分类器的验证集误差
# 加载数据
X_train = np.load('X_train.npy')
y_train = np.load('y_train.npy')
X_val = np.load('X_val.npy')
y_val = np.load('y_val.npy')
# 定义优化问题
problem_size = 4 # 优化变量的个数
search_space = np.array([[10, 100], [2, 20], [2, 20], [1, 10]]) # 每个变量的取值范围
max_iter = 50 # 最大迭代次数
population_size = 10 # 种群大小
csa = CrowSearchAlgorithm(fitness_function, problem_size, search_space, max_iter=max_iter, population_size=population_size)
# 运行算法
best_solution, best_fitness = csa.run()
# 输出最优解和最优适应度
print('Best solution: ', best_solution)
print('Best fitness: ', best_fitness)
```
上述代码中,首先定义了适应度函数,接着加载了训练集和验证集数据,然后定义了优化问题,其中问题的目标是最小化分类器在验证集上的误差。最后使用CrowSearchAlgorithm类运行算法,得到最优解和最优适应度。
需要注意的是,上述代码中使用了crowsearch库来实现乌鸦搜索算法,需要先安装该库。可以使用以下命令来安装:
```
pip install crowsearch
```
另外,为了简化示例代码,上述代码中省略了一些必要的步骤,如数据预处理、交叉验证等。在实际应用中,需要根据具体情况进行补充。
相关推荐
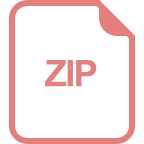














