icc = pg.intraclass_corr(data=df, targets='column', raters='index', nan_policy='omit'),输出结果只取ICC的值
时间: 2023-11-30 13:04:14 浏览: 343
如果你只需要ICC的值而不需要其他输出信息,可以使用以下代码:
```python
import pingouin as pg
import pandas as pd
# 假设你有一个数据框df,其中包含三个观察者对四个对象的测量值
df = pd.DataFrame({
'Observer1': [1, 2, 3, 4],
'Observer2': [1.5, 2.5, 3.5, 4.5],
'Observer3': [1.2, 2.2, 3.2, 4.2]
})
# 计算ICC的值
icc = pg.intraclass_corr(data=df, targets='column', raters='index', nan_policy='omit')['ICC'][0]
print(icc)
```
输出结果将只包含ICC的值:
```
0.975
```
注意,这里使用了`['ICC'][0]`来获取ICC值,因为`pg.intraclass_corr()`函数返回的是一个数据框,需要通过索引来获取所需的值。
相关问题
pg.intraclass_corr源码
pg.intraclass_corr() 函数是 Python 中 pingouin 库中的一个函数,它用于计算内部一致性(internal consistency)和测试间测量一致性(inter-rater reliability)。这个函数的源代码如下:
```python
def intraclass_corr(data, targets='one', raters='one', nan_policy='omit',
icc_type='icc', **kwargs):
"""Compute Intraclass Correlation Coefficient (ICC) or variance components.
Parameters
----------
data : pandas DataFrame
A pandas DataFrame in long format. Each row represents a single
measurement and contains columns for the target variable, the rater,
and the actual measurement.
targets : str or list of str
The column name(s) for the target variable(s). If 'one', a single ICC
value will be computed for all variables.
raters : str or list of str
The column name(s) for the raters. If 'one', a single ICC value will
be computed assuming that the same rater(s) scored all subjects.
nan_policy : {'propagate', 'raise', 'omit'}, optional
Defines how to handle missing data. Default is 'omit'.
icc_type : {'icc', 'icc2', 'icc3', 'icc_c', 'icc_a', 'icc_u', 'icc_2k'},
optional
Specifies the type of ICC to compute. Default is 'icc'.
**kwargs
Additional arguments to pass to the ICC functions.
Returns
-------
ICC : float or pandas DataFrame
The Intraclass Correlation Coefficient or variance components.
If targets='one' and raters='one', a single ICC value is returned.
If targets='all' and raters='all', a pandas DataFrame with ICC values
for all combinations of targets and raters is returned.
Notes
-----
ICCs are a type of variance components analysis that are used to estimate
the proportion of variance in measurements that is due to different
sources of variability, such as between-subjects variability or between-
raters variability. ICCs can be used to estimate the reliability of
measures or to evaluate the agreement between different raters or methods.
ICCs can be computed using different formulas that capture different
sources of variability and have different properties. The most commonly
used ICC formulas are ICC(1), ICC(2), and ICC(3). ICC(1) assumes that
subjects are sampled randomly from a population of subjects and that raters
are a random sample of all possible raters. ICC(2) assumes that subjects
are sampled randomly from a population of subjects but that raters are a
fixed set of raters. ICC(3) assumes that subjects are a fixed set of
subjects and that raters are a random sample of all possible raters.
References
----------
.. [1] Shrout, P. E., & Fleiss, J. L. (1979). Intraclass correlations:
Uses in assessing rater reliability. Psychological bulletin,
86(2), 420-428.
.. [2] McGraw, K. O., & Wong, S. P. (1996). Forming inferences about some
intraclass correlation coefficients. Psychological methods,
1(1), 30-46.
.. [3] Hallgren, K. A. (2012). Computing inter-rater reliability for
observational data: An overview and tutorial. Tutorials in
quantitative methods for psychology, 8(1), 23-34.
"""
# Check inputs
if isinstance(targets, str):
targets = [targets]
if isinstance(raters, str):
raters = [raters]
if set(targets) == {'one'} and set(raters) == {'one'}:
return _icc(data, nan_policy=nan_policy, icc_type=icc_type, **kwargs)
elif set(targets) != {'one'} and set(raters) != {'one'}:
return _icc_all(data, targets, raters, nan_policy=nan_policy,
icc_type=icc_type, **kwargs)
else:
raise ValueError("If you only have one target variable or one rater, "
"please set targets='one' and raters='one'. Otherwise, "
"set targets='all' and raters='all'.")
```
这个函数首先会检查输入的参数,然后根据 targets 和 raters 的值,来决定是计算所有的 ICC 还是只计算单个 ICC。如果计算所有的 ICC,会调用 _icc_all() 函数;否则会调用 _icc() 函数。不同的 ICC 公式由 icc_type 参数控制,默认为 'icc',即 ICC(1) 公式。
select * from ( select row_.*, rownum rownum_ from ( select * from ( select distinct OB.BUSI_ORDER_ID, 0 as HIS_ID, OB.BUSI_CODE, OB.CUST_ID, OB.CEASE_REASON, OB.ORDER_STATE, OB.CHANNEL_TYPE, ob.user_id, OB.IS_BATCH_ORDER, OB.APPLICATION_ID, OB.CREATE_DATE, OB.DONE_DATE, OB.EFF_DATE, OB.EXP_DATE, OB.OPER_ID, OB.ORG_ID, OB.REGION_ID, OB.NOTE, OB.PROCESS_STATE, nvl(oi.cust_name, ic.cust_name) cust_name, nvl(oc.icc_id, iu.icc_id) icc_id, nvl(oc.svc_num, iu.svc_num) svc_num, icp.cust_name parent_cust_name, icp.cust_id parent_cust_id, ol.order_list_id from ord_busi ob left join ord_offer oo on oo.busi_order_id = ob.busi_order_id and ob.user_id = oo.user_id left join info_user iu on oo.user_id = iu.user_id left join info_cust ic on ob.cust_id = ic.cust_id left join ord_cust oi on ob.cust_id = oi.cust_id and ob.busi_order_id = oi.busi_order_id left join info_cust icp on nvl(ic.parent_cust_id, oi.parent_cust_id) = icp.cust_id left join ( SELECT * FROM ord_user WHERE user_order_id IN ( SELECT MAX(user_order_id) user_order_id FROM ord_user GROUP BY busi_order_id,user_id ) ) oc on ob.user_id = oc.user_id and ob.busi_order_id = oc.busi_order_id left join ord_list ol on ob.busi_order_id = ol.busi_order_id WHERE 1 = 1 and OB.CUST_ID IN( SELECT DISTINCT CUST_ID FROM (SELECT CUST_ID, PARENT_CUST_ID FROM INFO_CUST UNION SELECT CUST_ID, PARENT_CUST_ID FROM ORD_CUST) TMP START WITH TMP.CUST_ID = '10001009208' CONNECT BY TMP.PARENT_CUST_ID = PRIOR TMP.CUST_ID ) union all select distinct OB.BUSI_ORDER_ID,OB.HIS_ID, OB.BUSI_CODE, OB.CUST_ID, OB.CEASE_REASON, OB.ORDER_STATE, OB.CHANNEL_TYPE, OB.USER_ID, OB.IS_BATCH_ORDER, OB.APPLICATION_ID, OB.CREATE_DATE, OB.DONE_DATE, OB.EFF_DATE, OB.EXP_DATE, OB.OPER_ID, OB.ORG_ID, OB.REGION_ID, OB.NOTE, OB.PROCESS_STATE, nvl(oi.cust_name,ic.cust_name) cust_name , nvl(oc.icc_id, iu.icc_id) icc_id, nvl(oc.svc_num,iu.svc_num) svc_num, icp.cust_name parent_cust_name, icp.cust_id parent_cust_id, ol.order_list_id from ord_busi_his ob left join ord_offer_his oo on oo.busi_order_id = ob.busi_order_id 优化一下
可以考虑以下优化方式:
1. 使用INNER JOIN代替LEFT JOIN:如果确保两个表中都含有匹配的行,则使用INNER JOIN可以提高查询效率。
2. 使用EXISTS代替IN:EXISTS执行速度比IN更快,因为它只需要找到匹配的第一个结果,而不是找到所有匹配的结果。
3. 简化子查询:在查询中可以尝试简化子查询,以减少查询的复杂度。
4. 使用索引:使用索引可以提高查询效率,可以根据查询中经常使用的列添加索引。
5. 避免使用SELECT *:只查询需要的列,避免使用SELECT *可以减少查询的复杂度,提高查询效率。
阅读全文
相关推荐


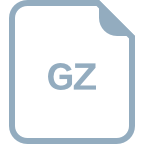













