再写一个行人属性识别训练没有验证集路径的图片代码
时间: 2024-02-21 14:02:20 浏览: 70
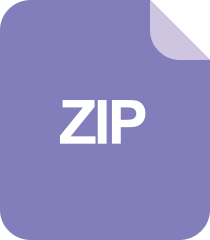
行人属性识别Rethinking-of-PAR源码+数据集PA100K+pt模型.zip

好的,以下是一个简单的行人属性识别训练代码,可以处理没有验证集路径的图片数据集:
```python
import os
import random
import numpy as np
from PIL import Image
import tensorflow as tf
# 定义数据集路径和标签
data_dir = "path/to/dataset"
labels = ["male", "female", "young", "old", "adult", "teenager"]
# 读取数据集并预处理
def preprocess_data():
data = []
for label in labels:
label_folder = os.path.join(data_dir, label)
for filename in os.listdir(label_folder):
img_path = os.path.join(label_folder, filename)
img = Image.open(img_path).resize((224, 224))
img = np.array(img) / 255.0
data.append((img, label))
random.shuffle(data)
return data
# 划分训练集和验证集
def split_data(data, split_ratio):
split_index = int(split_ratio * len(data))
train_data = data[:split_index]
val_data = data[split_index:]
return train_data, val_data
# 定义模型
def create_model():
base_model = tf.keras.applications.ResNet50(include_top=False, input_shape=(224, 224, 3))
for layer in base_model.layers:
layer.trainable = False
global_average_layer = tf.keras.layers.GlobalAveragePooling2D()
prediction_layer = tf.keras.layers.Dense(len(labels), activation="softmax")
model = tf.keras.Sequential([
base_model,
global_average_layer,
prediction_layer
])
model.compile(optimizer="adam", loss="sparse_categorical_crossentropy", metrics=["accuracy"])
return model
# 训练模型
def train_model():
data = preprocess_data()
train_data, val_data = split_data(data, 0.8)
train_images, train_labels = zip(*train_data)
val_images, val_labels = zip(*val_data)
train_images = np.array(train_images)
train_labels = np.array([labels.index(label) for label in train_labels])
val_images = np.array(val_images)
val_labels = np.array([labels.index(label) for label in val_labels])
model = create_model()
model.fit(train_images, train_labels, epochs=10, validation_data=(val_images, val_labels))
return model
# 测试模型
def test_model(model):
test_images = []
for filename in os.listdir("path/to/test/images"):
img_path = os.path.join("path/to/test/images", filename)
img = Image.open(img_path).resize((224, 224))
img = np.array(img) / 255.0
test_images.append(img)
test_images = np.array(test_images)
predictions = model.predict(test_images)
predicted_labels = [labels[np.argmax(prediction)] for prediction in predictions]
return predicted_labels
# 训练并测试模型
model = train_model()
predicted_labels = test_model(model)
print(predicted_labels)
```
这个代码中,我们首先定义了数据集路径和标签,然后使用 `preprocess_data()` 函数读取数据集并预处理,接着使用 `split_data()` 函数划分训练集和验证集,然后使用 `create_model()` 函数定义模型,使用 `train_model()` 函数训练模型,使用 `test_model()` 函数测试模型,最后输出预测标签。需要注意的是,由于没有验证集路径,我们在训练模型时直接使用了划分后的训练集和验证集,而在测试模型时使用了预定义的测试图片路径。
阅读全文
相关推荐
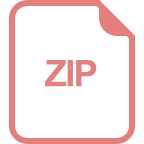
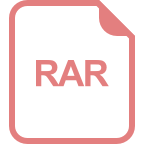















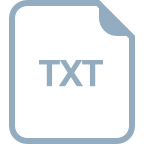