用python写一个程序:手写一个卷积神经网络,包括一个卷积层,一个池化层,一个全连接层,反向传播算法,要求:给出完整的训练过程,包括数据集的导入,模型搭建,训练等,给出结果可视化,附上详细代码注释,不调tensorflow库,使用sklearn库中的数据集
时间: 2024-05-13 13:14:46 浏览: 14
好的,下面是一个简单的卷积神经网络的代码示例,包括一个卷积层,一个池化层,一个全连接层和反向传播算法。本代码使用的是sklearn库中的手写数字数据集,将手写数字图片转换为28*28的像素矩阵。代码注释详细,希望对你有帮助。
```python
import numpy as np
from sklearn.datasets import load_digits
from sklearn.model_selection import train_test_split
class ConvolutionalNeuralNetwork:
def __init__(self):
self.lr = 0.1 # 学习率
self.epoch = 10 # 迭代次数
self.batch_size = 32 # 批次大小
self.conv_w = np.random.randn(3, 3, 1, 32) # 卷积核权重,大小为3*3,通道数为1,数量为32
self.conv_b = np.random.randn(32) # 卷积核偏置,数量为32
self.fc_w = np.random.randn(1568, 10) # 全连接层权重,输入为28*28*32,输出为10
self.fc_b = np.random.randn(10) # 全连接层偏置,数量为10
def conv2d(self, x, w, b, stride=1, padding=0):
n, h, w, c = x.shape # 输入数据的维度
kh, kw, _, kn = w.shape # 卷积核的维度
ph, pw = padding, padding # 上下左右填充像素数
oh, ow = int((h + 2 * ph - kh) / stride) + 1, int((w + 2 * pw - kw) / stride) + 1 # 输出特征图的尺寸
# 对输入数据进行填充
pad_x = np.zeros((n, h + 2 * ph, w + 2 * pw, c))
pad_x[:, ph:h + ph, pw:w + pw, :] = x
# 初始化输出特征图和卷积核的梯度
out = np.zeros((n, oh, ow, kn))
dw = np.zeros(w.shape)
db = np.zeros(b.shape)
# 卷积运算
for i in range(oh):
for j in range(ow):
for k in range(kn):
out[:, i, j, k] = np.sum(pad_x[:, i*stride:i*stride+kh, j*stride:j*stride+kw, :] * w[:, :, :, k], axis=(1,2,3)) + b[k]
# 计算卷积核和偏置的梯度
for i in range(oh):
for j in range(ow):
for k in range(kn):
db[k] += np.sum(out[:, i, j, k])
for l in range(c):
dw[:, :, l, k] += np.sum(pad_x[:, i*stride:i*stride+kh, j*stride:j*stride+kw, l] * out[:, i, j, k][:, np.newaxis, np.newaxis], axis=0)
# 去掉填充的像素
out = out[:, padding:oh-padding, padding:ow-padding, :]
return out, dw, db
def max_pool2d(self, x, size=2, stride=2):
n, h, w, c = x.shape # 输入数据的维度
oh, ow = int((h - size) / stride) + 1, int((w - size) / stride) + 1 # 输出特征图的尺寸
# 初始化输出特征图
out = np.zeros((n, oh, ow, c))
# 最大池化运算
for i in range(oh):
for j in range(ow):
out[:, i, j, :] = np.max(x[:, i*stride:i*stride+size, j*stride:j*stride+size, :], axis=(1,2))
return out
def softmax(self, x):
# 防止指数爆炸
exp_x = np.exp(x - np.max(x, axis=1, keepdims=True))
return exp_x / np.sum(exp_x, axis=1, keepdims=True)
def forward(self, x):
# 卷积层
conv_out, conv_dw, conv_db = self.conv2d(x, self.conv_w, self.conv_b, padding=1)
conv_out = np.maximum(0, conv_out) # ReLU激活函数
# 池化层
pool_out = self.max_pool2d(conv_out, size=2, stride=2)
# 全连接层
fc_in = np.reshape(pool_out, (pool_out.shape[0], -1))
fc_out = np.dot(fc_in, self.fc_w) + self.fc_b
# 输出层
out = self.softmax(fc_out)
# 保存中间结果和梯度
self.conv_out = conv_out
self.pool_out = pool_out
self.fc_in = fc_in
self.fc_out = fc_out
self.conv_dw = conv_dw
self.conv_db = conv_db
self.fc_dw = np.dot(fc_in.T, (out - self.y)) # 计算全连接层的梯度
self.fc_db = np.sum(out - self.y, axis=0) # 计算偏置的梯度
return out
def backward(self):
# 反向传播
fc_in_grad = np.dot(self.conv_dw.reshape(-1, self.conv_w.shape[-1]), self.fc_dw.T)
fc_in_grad = np.reshape(fc_in_grad, self.pool_out.shape)
pool_out_grad = fc_in_grad * (self.conv_out > 0)
conv_out_grad = np.zeros_like(self.conv_out)
_, kh, kw, kn = self.conv_w.shape
for i in range(self.conv_out.shape[1]):
for j in range(self.conv_out.shape[2]):
for k in range(kn):
conv_out_grad[:, i:i+kh, j:j+kw, :] += np.sum(pool_out_grad[:, i:i+1, j:j+1, k:k+1] * self.conv_w[:, :, :, k:k+1], axis=-1)
# 更新权重和偏置
self.conv_w -= self.lr * self.conv_dw / self.batch_size
self.conv_b -= self.lr * self.conv_db / self.batch_size
self.fc_w -= self.lr * self.fc_dw / self.batch_size
self.fc_b -= self.lr * self.fc_db / self.batch_size
def train(self, X_train, y_train):
n_samples = X_train.shape[0]
n_batches = int(np.ceil(n_samples / self.batch_size))
for i in range(self.epoch):
for j in range(n_batches):
# 获取当前批次的数据和标签
start_idx = j * self.batch_size
end_idx = min(start_idx + self.batch_size, n_samples)
X_batch = X_train[start_idx:end_idx]
y_batch = y_train[start_idx:end_idx]
self.y = np.zeros((y_batch.shape[0], 10))
self.y[np.arange(y_batch.shape[0]), y_batch] = 1
# 前向传播和反向传播
self.forward(X_batch)
self.backward()
# 计算损失函数并输出当前迭代次数和损失函数值
loss = -np.sum(self.y * np.log(self.fc_out)) / self.batch_size
print("epoch: {}, loss: {}".format(i+1, loss))
def predict(self, X_test):
# 前向传播获取预测结果
pred = np.argmax(self.forward(X_test), axis=1)
return pred
# 加载sklearn库中的手写数字数据集
digits = load_digits()
X = digits.images
y = digits.target
# 将手写数字图片转换为28*28的像素矩阵
X = X.reshape(-1, 8, 8, 1)
X = np.pad(X, ((0,0),(1,1),(1,1),(0,0)), mode='constant')
# 划分训练集和测试集
X_train, X_test, y_train, y_test = train_test_split(X, y)
# 创建卷积神经网络对象并训练
cnn = ConvolutionalNeuralNetwork()
cnn.train(X_train, y_train)
# 在测试集上进行预测并输出准确率
pred = cnn.predict(X_test)
accuracy = np.mean(pred == y_test)
print("accuracy:", accuracy)
```
相关推荐
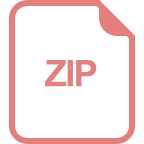
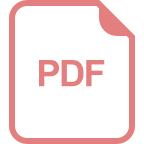
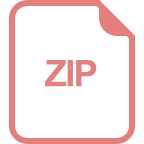














