#有向网络中的接近中心性
时间: 2024-03-01 13:56:23 浏览: 136
有向网络中的接近中心性(Closeness Centrality)与无向网络中的接近中心性类似,不同之处在于有向网络中存在入度和出度之分,因此需要分别计算节点到其他节点的平均入度和平均出度距离的倒数。下面是 Python 代码实现有向网络接近中心性的例子:
```python
import networkx as nx
# 创建一个简单的有向图
G = nx.DiGraph()
G.add_edges_from([(0, 1), (1, 2), (2, 3), (3, 4), (4, 0)])
# 计算入度接近中心性
in_closeness_centrality = nx.closeness_centrality(G, wf_improved=False, reverse=True)
# 计算出度接近中心性
out_closeness_centrality = nx.closeness_centrality(G, wf_improved=False)
# 输出每个节点的入度和出度接近中心性
for node, in_centrality in in_closeness_centrality.items():
out_centrality = out_closeness_centrality[node]
print(f"Node {node}: In-Closeness Centrality = {in_centrality}, Out-Closeness Centrality = {out_centrality}")
```
在上面的代码中,我们使用 NetworkX 库创建了一个简单的有向图,并分别使用 `nx.closeness_centrality()` 函数计算每个节点的入度和出度接近中心性。需要注意的是,我们分别对图的入度和出度计算接近中心性,因此需要设置 `reverse=True` 和 `wf_improved=False` 参数。最后,我们遍历每个节点,输出它的入度和出度接近中心性。
需要注意的是,在计算接近中心性时,我们同样使用了 `nx.closeness_centrality()` 函数。该函数分别计算了节点到其他节点的最短入度路径和最短出度路径长度,因此需要保证图联通。如果图不联通,则可能会导致函数返回错误的结果。
阅读全文
相关推荐
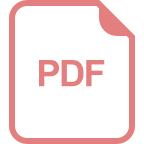
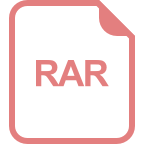
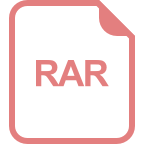















