markov(a,init_mat,trans_mat,emit_mat)
时间: 2024-04-26 20:22:27 浏览: 12
这是一个基于马尔可夫链的文本生成函数,它接收四个参数:
- a:一个包含文本的字符串或列表。
- init_mat:一个表示初始状态的向量或矩阵。
- trans_mat:一个表示转移概率的矩阵。
- emit_mat:一个表示发射概率的矩阵。
函数的作用是根据传入的参数生成一个新的文本,其生成过程基于马尔可夫链的思想。具体地,在开始时,函数根据 init_mat 确定初始状态,然后根据 trans_mat 和当前状态计算下一个状态,再根据 emit_mat 和当前状态生成一个字符,然后将该字符添加到生成的文本中,并将状态更新为新的状态。这个过程重复进行,直到生成的文本达到指定长度或无法继续生成为止。
相关问题
markov(a,init_mat,trans_mat,emit_mat) init_mat_compute = copy.deepcopy(init_mat) trans_mat_compute = copy.deepcopy(trans_mat) emit_mat_compute = copy.deepcopy(emit_mat) print(init_mat) print(trans_mat) print(emit_mat) compute(init_mat_compute,trans_mat_compute,emit_mat_compute) print("当前初始状态向量", init_mat_compute) print("当前转移矩阵", trans_mat_compute) print("当前发射矩阵:",emit_mat_compute)
这段代码展示了一个函数markov的调用过程,以及在函数内部对三个字典变量进行深拷贝并打印出来的结果。
具体来说,代码首先调用了名为markov的函数,该函数接受四个参数:a、init_mat、trans_mat和emit_mat。接下来,代码使用copy.deepcopy函数分别对init_mat、trans_mat和emit_mat进行深拷贝,得到三个新的字典变量init_mat_compute、trans_mat_compute和emit_mat_compute,这是因为markov函数内部会对这三个字典进行修改,为了避免在函数外部修改原始字典,需要进行深拷贝。
接着,代码打印出了init_mat、trans_mat和emit_mat三个字典的内容,以便查看它们的初始状态。然后,代码调用了compute函数,该函数会对init_mat_compute、trans_mat_compute和emit_mat_compute三个字典进行修改,因此这三个字典的内容会发生改变。
最后,代码又一次打印出了init_mat_compute、trans_mat_compute和emit_mat_compute三个字典的内容,以便查看它们在compute函数中被修改后的状态。
这段代码什么作用def get_tag(word): tag=[] if len(word)==1: tag = ['S'] elif len(word)==2: tag = ['B','E'] else: num = len(word)-2 tag.append('B') tag.extend(['M']*num) tag.append('E') return tag def compute(init_mat,trans_mat,emit_mat): init_sum = sum(init_mat.values()) for key,value in init_mat.items(): init_mat[key] = round(value/init_sum,3) for key,value in trans_mat.items(): cur_sum = sum(value.values()) if(cur_sum==0): continue for i,j in value.items(): trans_mat[key][i] = round(j/cur_sum,3) emit_list = emit_mat.values.tolist() for i in range(len(emit_list)): cur_sum = sum(emit_list[i]) if (cur_sum == 0): continue for j in range(len(emit_list[i])): emit_mat.iloc[i,j] = round(emit_list[i][j]/cur_sum,3) def markov(txt,init_mat,trans_mat,emit_mat):
这段代码定义了三个函数,分别是 `get_tag(word)`、`compute(init_mat,trans_mat,emit_mat)` 和 `markov(txt,init_mat,trans_mat,emit_mat)`。
`get_tag(word)` 函数的作用是根据输入的中文词汇 `word` 返回其对应的词性标记。如果词汇长度为 1,则其标记为 'S';如果长度为 2,则其标记为 'B' 和 'E';否则,其标记为 'B'、若干个 'M' 和 'E'。
`compute(init_mat,trans_mat,emit_mat)` 函数用于计算并更新初始概率矩阵 `init_mat`、转移概率矩阵 `trans_mat` 和发射概率矩阵 `emit_mat`,其中 `init_mat` 和 `trans_mat` 是字典类型,`emit_mat` 是 pandas 的 DataFrame 类型。
`markov(txt,init_mat,trans_mat,emit_mat)` 函数的作用是根据输入的中文文本 `txt` 和三个概率矩阵 `init_mat`、`trans_mat`、`emit_mat` 进行隐马尔可夫模型的分词,返回分好词的结果。
相关推荐
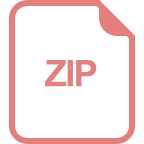
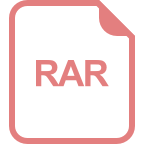












