test_normal_data = np.empty((0, param["feature"]["n_frames"] * 129), float)
时间: 2024-05-22 14:14:53 浏览: 120
As an AI language model, I am not provided with complete context of this code snippet. However, based on the provided code, it seems that this line is creating an empty numpy array with dimensions (0, n_frames * 129) to store normal data. The n_frames represents the number of frames and 129 represents the number of features per frame. This array will be populated with normal data later in the code.
相关问题
给我解释 test_normal_data = np.empty((0, param["feature"]["n_frames"] * 129), float)
这行代码创建了一个空的 numpy 数组,其形状为 (0, n_frames * 129),其中 n_frames 是一个指定的参数。这个数组的数据类型为 float。
在这个代码中,使用了 np.empty() 方法来创建一个没有被初始化的、具有特定形状和数据类型的数组。这里的形状是 (0, n_frames * 129),其中的 0 表示该数组没有任何行,但是其列数为 n_frames * 129。
该数组被赋值给了 test_normal_data 变量,用于存储测试数据。具体来说,这个数组可以存储多个音频文件的特征数据,每个音频文件的特征数据通过一行来表示,每行的长度是 n_frames * 129。在实际使用中,我们可以通过不断往这个数组中添加行来存储更多的音频文件的特征数据。
阅读全文
相关推荐
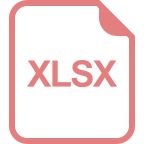
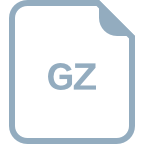
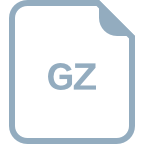
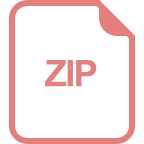
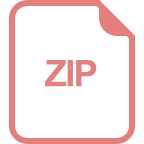
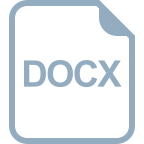
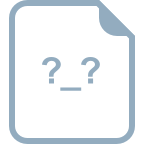
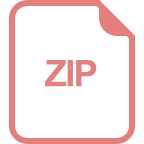
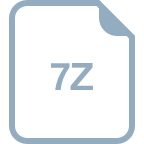
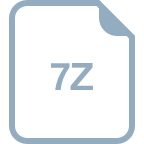
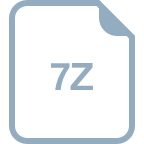
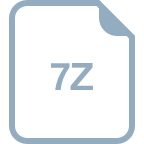
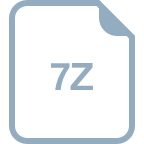
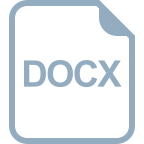
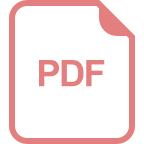
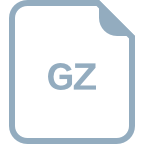