对彩色图像应用log算子、sobel算子、prewitt算子和roberts算子进行边缘检测
时间: 2023-12-29 08:06:44 浏览: 77
边缘检测是图像处理中常见的一种操作,可以通过不同的算子来实现。以下是使用log算子、sobel算子、prewitt算子和roberts算子对彩色图像进行边缘检测的步骤:
1. 将彩色图像转换为灰度图像。
2. 对灰度图像应用log算子,得到边缘强度图像。
3. 对灰度图像应用sobel算子,得到水平和垂直方向的边缘强度图像,然后将两个图像合并得到综合边缘强度图像。
4. 对灰度图像应用prewitt算子,得到水平和垂直方向的边缘强度图像,然后将两个图像合并得到综合边缘强度图像。
5. 对灰度图像应用roberts算子,得到水平和垂直方向的边缘强度图像,然后将两个图像合并得到综合边缘强度图像。
6. 可以通过设置阈值来对边缘强度图像进行二值化处理,从而得到二值化的边缘图像。
需要注意的是,以上步骤中的算子都是针对灰度图像的,因此在处理彩色图像时要先将其转换为灰度图像。此外,不同的算子会得到不同的边缘强度图像,因此根据具体应用场景选择合适的算子是十分重要的。
相关问题
读取一张彩色图像,分别用log算子、sobel算子、prewitt算子和roberts算子对图像进行边缘检测。
好的,我可以帮您实现这个任务。这里假设您使用的是Python语言和OpenCV库。
首先,读取一张彩色图像可以使用OpenCV库中的`cv2.imread()`函数。该函数返回一个3维的numpy数组,其中第三个维度表示图像的颜色通道(RGB)。代码如下:
```python
import cv2
img = cv2.imread('image.jpg')
```
接下来,我们可以将彩色图像转换为灰度图像,因为边缘检测通常只需要一个通道的图像。可以使用OpenCV库中的`cv2.cvtColor()`函数将图像从BGR颜色空间转换为灰度颜色空间。代码如下:
```python
gray_img = cv2.cvtColor(img, cv2.COLOR_BGR2GRAY)
```
然后,我们可以使用log算子、sobel算子、prewitt算子和roberts算子对图像进行边缘检测。可以使用OpenCV库中的`cv2.filter2D()`函数进行卷积操作。代码如下:
```python
import numpy as np
# Log算子
kernel_log = np.array([[0, 0, -1, 0, 0],
[0, -1, -2, -1, 0],
[-1, -2, 16, -2, -1],
[0, -1, -2, -1, 0],
[0, 0, -1, 0, 0]])
log_img = cv2.filter2D(gray_img, -1, kernel_log)
# Sobel算子
kernel_sobel_x = np.array([[-1, 0, 1],
[-2, 0, 2],
[-1, 0, 1]])
sobel_x_img = cv2.filter2D(gray_img, -1, kernel_sobel_x)
kernel_sobel_y = np.array([[-1, -2, -1],
[0, 0, 0],
[1, 2, 1]])
sobel_y_img = cv2.filter2D(gray_img, -1, kernel_sobel_y)
sobel_img = np.sqrt(sobel_x_img**2 + sobel_y_img**2)
# Prewitt算子
kernel_prewitt_x = np.array([[-1, 0, 1],
[-1, 0, 1],
[-1, 0, 1]])
prewitt_x_img = cv2.filter2D(gray_img, -1, kernel_prewitt_x)
kernel_prewitt_y = np.array([[-1, -1, -1],
[0, 0, 0],
[1, 1, 1]])
prewitt_y_img = cv2.filter2D(gray_img, -1, kernel_prewitt_y)
prewitt_img = np.sqrt(prewitt_x_img**2 + prewitt_y_img**2)
# Roberts算子
kernel_roberts_x = np.array([[1, 0],
[0, -1]])
roberts_x_img = cv2.filter2D(gray_img, -1, kernel_roberts_x)
kernel_roberts_y = np.array([[0, 1],
[-1, 0]])
roberts_y_img = cv2.filter2D(gray_img, -1, kernel_roberts_y)
roberts_img = np.sqrt(roberts_x_img**2 + roberts_y_img**2)
```
最后,将每种边缘检测算子得到的图像显示出来,可以使用OpenCV库中的`cv2.imshow()`函数。完整代码如下:
```python
import cv2
import numpy as np
img = cv2.imread('image.jpg')
gray_img = cv2.cvtColor(img, cv2.COLOR_BGR2GRAY)
# Log算子
kernel_log = np.array([[0, 0, -1, 0, 0],
[0, -1, -2, -1, 0],
[-1, -2, 16, -2, -1],
[0, -1, -2, -1, 0],
[0, 0, -1, 0, 0]])
log_img = cv2.filter2D(gray_img, -1, kernel_log)
# Sobel算子
kernel_sobel_x = np.array([[-1, 0, 1],
[-2, 0, 2],
[-1, 0, 1]])
sobel_x_img = cv2.filter2D(gray_img, -1, kernel_sobel_x)
kernel_sobel_y = np.array([[-1, -2, -1],
[0, 0, 0],
[1, 2, 1]])
sobel_y_img = cv2.filter2D(gray_img, -1, kernel_sobel_y)
sobel_img = np.sqrt(sobel_x_img**2 + sobel_y_img**2)
# Prewitt算子
kernel_prewitt_x = np.array([[-1, 0, 1],
[-1, 0, 1],
[-1, 0, 1]])
prewitt_x_img = cv2.filter2D(gray_img, -1, kernel_prewitt_x)
kernel_prewitt_y = np.array([[-1, -1, -1],
[0, 0, 0],
[1, 1, 1]])
prewitt_y_img = cv2.filter2D(gray_img, -1, kernel_prewitt_y)
prewitt_img = np.sqrt(prewitt_x_img**2 + prewitt_y_img**2)
# Roberts算子
kernel_roberts_x = np.array([[1, 0],
[0, -1]])
roberts_x_img = cv2.filter2D(gray_img, -1, kernel_roberts_x)
kernel_roberts_y = np.array([[0, 1],
[-1, 0]])
roberts_y_img = cv2.filter2D(gray_img, -1, kernel_roberts_y)
roberts_img = np.sqrt(roberts_x_img**2 + roberts_y_img**2)
# 显示每种边缘检测算子得到的图像
cv2.imshow('Log', log_img)
cv2.imshow('Sobel', sobel_img)
cv2.imshow('Prewitt', prewitt_img)
cv2.imshow('Roberts', roberts_img)
cv2.waitKey(0)
cv2.destroyAllWindows()
```
这样,就可以看到每种边缘检测算子得到的图像了。
读取一副灰度图像,分别用 log 算子、 sobel 算子、 prewitt算子和 roberts 算子对图像进行边缘检测。
首先,需要导入必要的库和读取灰度图像。这里使用Python的OpenCV库进行处理。
```python
import cv2
import numpy as np
# 读取灰度图像
img = cv2.imread('image.jpg', 0)
```
接下来,分别应用log算子、sobel算子、prewitt算子和roberts算子进行边缘检测。
1. Log算子
Log算子是一种基于拉普拉斯变换的边缘检测算法,它可以增强图像的高频部分,使得边缘更加明显。
```python
# 定义log算子
log_kernel = np.array([[0, 0, -1, 0, 0],
[0, -1, -2, -1, 0],
[-1, -2, 16, -2, -1],
[0, -1, -2, -1, 0],
[0, 0, -1, 0, 0]])
# 应用log算子
log_img = cv2.filter2D(img, -1, log_kernel)
# 显示边缘检测结果
cv2.imshow('Log Edge Detection', log_img)
cv2.waitKey(0)
```
2. Sobel算子
Sobel算子是一种基于图像梯度的边缘检测算法,它可以检测出图像中的水平和竖直边缘。
```python
# 定义sobel算子
sobel_x = np.array([[-1, 0, 1],
[-2, 0, 2],
[-1, 0, 1]])
sobel_y = np.array([[-1, -2, -1],
[0, 0, 0],
[1, 2, 1]])
# 应用sobel算子
sobel_x_img = cv2.filter2D(img, -1, sobel_x)
sobel_y_img = cv2.filter2D(img, -1, sobel_y)
sobel_img = cv2.bitwise_or(sobel_x_img, sobel_y_img)
# 显示边缘检测结果
cv2.imshow('Sobel Edge Detection', sobel_img)
cv2.waitKey(0)
```
3. Prewitt算子
Prewitt算子也是一种基于图像梯度的边缘检测算法,它与Sobel算子类似,但是使用了不同的卷积核。
```python
# 定义prewitt算子
prewitt_x = np.array([[-1, 0, 1],
[-1, 0, 1],
[-1, 0, 1]])
prewitt_y = np.array([[-1, -1, -1],
[0, 0, 0],
[1, 1, 1]])
# 应用prewitt算子
prewitt_x_img = cv2.filter2D(img, -1, prewitt_x)
prewitt_y_img = cv2.filter2D(img, -1, prewitt_y)
prewitt_img = cv2.bitwise_or(prewitt_x_img, prewitt_y_img)
# 显示边缘检测结果
cv2.imshow('Prewitt Edge Detection', prewitt_img)
cv2.waitKey(0)
```
4. Roberts算子
Roberts算子是一种基于图像梯度的边缘检测算法,它使用了不同的卷积核计算图像中的边缘。
```python
# 定义roberts算子
roberts_x = np.array([[0, 0, 0],
[0, 1, 0],
[0, 0, -1]])
roberts_y = np.array([[0, 0, 0],
[0, 0, 1],
[0, -1, 0]])
# 应用roberts算子
roberts_x_img = cv2.filter2D(img, -1, roberts_x)
roberts_y_img = cv2.filter2D(img, -1, roberts_y)
roberts_img = cv2.bitwise_or(roberts_x_img, roberts_y_img)
# 显示边缘检测结果
cv2.imshow('Roberts Edge Detection', roberts_img)
cv2.waitKey(0)
```
最后,需要释放窗口和内存。
```python
# 释放窗口和内存
cv2.destroyAllWindows()
```
完整代码如下:
阅读全文
相关推荐
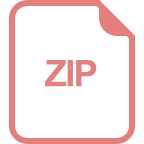



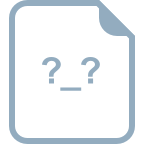









