我在搭建lime模型,报错如下:map_exp_ids() got an unexpected keyword argument 'labels'map_exp_ids() got an unexpected keyword argument 'labels'
时间: 2023-10-23 08:48:12 浏览: 40
这个报错提示表明在使用函数 `map_exp_ids()` 时,出现了一个不期望的关键字参数 `labels`。
可能的原因是,你使用的 Lime 库版本不同,或者你的代码中使用的参数和函数定义不匹配。
解决方案可能是修改函数调用,或者更新 Lime 库版本。你可以查看 Lime 库的文档或者参考其他类似问题的解决方案。
相关问题
jgz = np.array(exp.local_exp[1])
在上述代码中,exp.local_exp[1] 是 LIME 的解释结果中与目标类别相关的解释。具体来说,exp.local_exp 是一个字典,其中键为目标类别的索引,值为一个包含特征权重和对应特征名称的列表。因为我们只有一个目标类别,所以这里选取了键为 1 的值,即与目标类别相关的解释列表。
np.array(exp.local_exp[1]) 的作用是将解释列表转换为一个 NumPy 数组,以便于后续处理。这里之所以需要转换为数组,是因为解释列表中的每个元素都是一个元组,包含两个值:特征权重和对应特征名称。转换为数组后,我们可以方便地对解释结果进行处理和可视化。
飞腾开发板qt中输入QMAKE_CXXFLAGS += -mfpu=neon报错unrecognized commond lime"-mfpu=neon"
如果你在Qt应用程序的.pro文件中输入"QMAKE_CXXFLAGS += -mfpu=neon"时,遇到了"unrecognized command line option '-mfpu=neon'"的错误,很可能是因为你使用的编译器不支持-mfpu选项。
在这种情况下,你需要使用编译器支持的相应选项来开启NEON指令集。例如,如果你使用的是GCC编译器,可以尝试将选项更改为:
```qmake
QMAKE_CXXFLAGS += -march=armv7-a -mtune=cortex-a53 -mcpu=native
```
这个选项会启用ARMv7-A体系结构的NEON指令集。请注意,这个选项只适用于特定的ARM CPU架构,如果你的CPU架构不同,需要相应地进行调整。
如果你使用的是其他编译器,请查阅相应的文档,了解如何开启NEON指令集。
相关推荐
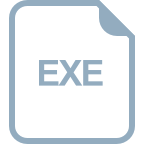












