plt.style.use(style_name)
时间: 2023-08-17 10:46:01 浏览: 30
`plt.style.use(style_name)` 是Matplotlib中的一个函数,用于设置图形的样式。Matplotlib提供了许多内置的样式,例如 `'ggplot'`、`'seaborn'`、`'bmh'` 等,可以通过将样式名称作为参数传递给 `use()` 函数来使用这些样式。此外,也可以创建自定义样式文件,将其保存在本地目录中,并使用 `use()` 函数来加载该样式文件。例如,`plt.style.use('ggplot')` 将使用内置的 `'ggplot'` 样式来设置图形的外观。可以在 `use()` 函数中指定多个样式名称,以便组合多个样式来自定义图形的外观。
相关问题
python plt.style.use
"python plt.style.use"是一条Python代码,它可以用于设置matplotlib绘图库的风格。具体来说,通过使用plt.style.use()方法,可以选择不同的样式表来设置图形的颜色、线型、字体等属性,从而让绘图更美观可读。例如,使用“plt.style.use('ggplot')”可以让图形呈现出灰色背景和细线条的风格。
plt.style.use( seaborn )报错
在使用 `plt.style.use()` 函数时,需要使用引号将样式名称包裹起来。如果您使用了引号但仍然报错,可能是因为 `seaborn` 没有被正确导入。您需要在代码开头添加以下行:
```python
import seaborn as sns
```
然后在使用 `plt.style.use()` 函数时,将 `seaborn` 样式名称用引号包裹起来,例如:
```python
import seaborn as sns
import matplotlib.pyplot as plt
sns.set(style='whitegrid')
plt.style.use('seaborn')
```
这样就可以使用 `seaborn` 样式了。如果仍然有问题,请提供完整的错误信息以供参考。
相关推荐
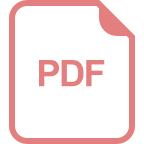












