keras实现用Googlenet对四种天气分类,并绘制图像
时间: 2024-02-01 21:12:37 浏览: 73
首先,我们需要准备数据集。假设我们有一个包含四种天气(晴天、多云、雨天、雪天)的数据集,其中每个类别包含100张图片。我们将这些图片分为训练集和测试集,其中训练集包含80张图片,测试集包含20张图片。
接下来,我们需要使用Keras实现GoogLeNet模型。以下是代码:
```python
from keras.layers import Input, Conv2D, MaxPooling2D, Dropout, Dense, AveragePooling2D, Flatten, concatenate
from keras.models import Model
def inception_module(x, filters):
filter1, filter3, filter5, filterpool = filters
conv1 = Conv2D(filter1, (1,1), padding='same', activation='relu')(x)
conv3 = Conv2D(filter3, (1,1), padding='same', activation='relu')(x)
conv3 = Conv2D(filter3, (3,3), padding='same', activation='relu')(conv3)
conv5 = Conv2D(filter5, (1,1), padding='same', activation='relu')(x)
conv5 = Conv2D(filter5, (5,5), padding='same', activation='relu')(conv5)
maxpool = MaxPooling2D((3,3), strides=(1,1), padding='same')(x)
maxpool = Conv2D(filterpool, (1,1), padding='same', activation='relu')(maxpool)
output = concatenate([conv1, conv3, conv5, maxpool], axis=3)
return output
input_layer = Input(shape=(224,224,3))
conv1 = Conv2D(64, (7,7), strides=(2,2), padding='same', activation='relu')(input_layer)
maxpool1 = MaxPooling2D((3,3), strides=(2,2), padding='same')(conv1)
conv2 = Conv2D(64, (1,1), padding='same', activation='relu')(maxpool1)
conv2 = Conv2D(192, (3,3), padding='same', activation='relu')(conv2)
maxpool2 = MaxPooling2D((3,3), strides=(2,2), padding='same')(conv2)
inception3a = inception_module(maxpool2, [64, 96, 128, 16])
inception3b = inception_module(inception3a, [128, 128, 192, 32])
maxpool3 = MaxPooling2D((3,3), strides=(2,2), padding='same')(inception3b)
inception4a = inception_module(maxpool3, [192, 96, 208, 16])
inception4b = inception_module(inception4a, [160, 112, 224, 24])
inception4c = inception_module(inception4b, [128, 128, 256, 24])
inception4d = inception_module(inception4c, [112, 144, 288, 32])
inception4e = inception_module(inception4d, [256, 160, 320, 32])
maxpool4 = MaxPooling2D((3,3), strides=(2,2), padding='same')(inception4e)
inception5a = inception_module(maxpool4, [256, 160, 320, 32])
inception5b = inception_module(inception5a, [384, 192, 384, 48])
averagepool = AveragePooling2D((7,7), strides=(7,7))(inception5b)
flatten = Flatten()(averagepool)
dropout = Dropout(0.4)(flatten)
output_layer = Dense(4, activation='softmax')(dropout)
model = Model(inputs=input_layer, outputs=output_layer)
model.summary()
```
然后,我们需要编译并训练模型。以下是代码:
```python
from keras.optimizers import SGD
from keras.preprocessing.image import ImageDataGenerator
train_datagen = ImageDataGenerator(rescale=1./255)
test_datagen = ImageDataGenerator(rescale=1./255)
train_generator = train_datagen.flow_from_directory(
'train/',
target_size=(224,224),
batch_size=16,
class_mode='categorical'
)
test_generator = test_datagen.flow_from_directory(
'test/',
target_size=(224,224),
batch_size=16,
class_mode='categorical'
)
sgd = SGD(lr=0.01, decay=1e-6, momentum=0.9, nesterov=True)
model.compile(optimizer=sgd, loss='categorical_crossentropy', metrics=['accuracy'])
model.fit_generator(train_generator, steps_per_epoch=80, epochs=50, validation_data=test_generator, validation_steps=20)
```
最后,我们可以使用以下代码进行预测并绘制图像:
```python
import numpy as np
from keras.preprocessing import image
import matplotlib.pyplot as plt
test_image = image.load_img('test_image.jpg', target_size=(224,224))
test_image = image.img_to_array(test_image)
test_image = np.expand_dims(test_image, axis=0)
result = model.predict(test_image)
labels = ['Sunny', 'Cloudy', 'Rainy', 'Snowy']
plt.imshow(image.load_img('test_image.jpg'))
plt.title(labels[np.argmax(result)])
plt.show()
```
注意,上述代码中的`'train/'`和`'test/'`分别表示训练集和测试集的文件夹路径,你需要根据实际情况修改这些路径。另外,`'test_image.jpg'`表示要进行预测的图像文件名,你也需要根据实际情况修改这个文件名。
阅读全文
相关推荐
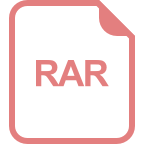




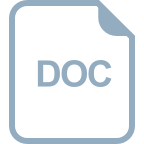
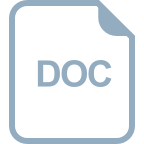
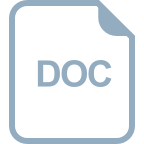
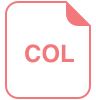
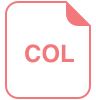
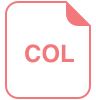
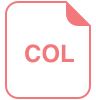
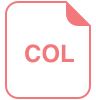
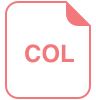
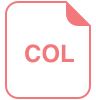
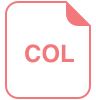
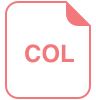