DeepFace.verify(img1_path=img1_path, img2_path=img2_path, enforce_detection=False, model=net, histogram=histogram, grayscale=False, pose_estimator=pose_estimator, landmarks=landmarks) 什么意思
时间: 2024-04-02 16:37:23 浏览: 16
这段代码使用DeepFace库中的verify()函数来比较两张人脸照片的相似度。该函数接受以下参数:
- img1_path: 第一张人脸照片的路径;
- img2_path: 第二张人脸照片的路径;
- enforce_detection: 是否强制检测到人脸。如果为True,则会在照片中检测到人脸后才会进行比较。如果为False,则不会强制检测到人脸,直接进行比较;
- model: 人脸识别模型。如果为None,则使用默认的人脸识别模型;
- histogram: 是否使用直方图对比。如果为True,则使用直方图对比来计算相似度。如果为False,则使用DeepFace库提供的模型计算相似度;
- grayscale: 是否将图片转化为灰度图。如果为True,则将图片转化为灰度图再进行比较。如果为False,则使用彩色图片进行比较;
- pose_estimator: 用于估计人脸姿态的模型。如果为None,则使用默认的姿态估计模型;
- landmarks: 人脸关键点检测模型。如果为None,则使用默认的人脸关键点检测模型。
该函数会返回一个浮点数,表示两张人脸照片的相似度。相似度越高,表示两张照片中的人脸越相似。
相关问题
Traceback (most recent call last): File "D:\pythonProject\face\verifyapi.py", line 17, in <module> print(verify(r'static/picture/11.jpg',r'static/temp/11.jpg')) File "D:\pythonProject\face\verifyapi.py", line 7, in verify result = DeepFace.verify(img1_path=img1_path, img2_path=img2_path, enforce_detection=False, model=net, File "D:\pythonProject\face\deepface\DeepFace.py", line 153, in verify img1_objs = functions.extract_faces( File "D:\pythonProject\face\deepface\commons\functions.py", line 201, in extract_faces face_alignment = FaceAlignment(LandmarksType._2D, flip_input=False, device='cuda') File "D:\anaconda3\lib\enum.py", line 429, in getattr raise AttributeError(name) from None AttributeError: _2D 怎么解决
这个错误提示是因为枚举类型 LandmarksType 中没有属性名为 '_2D' 的成员。在调用 FaceAlignment 函数时,第一个参数应该传入 LandmarksType 枚举类型的成员,指定使用的人脸关键点检测算法。
解决方法是检查传入的参数值是否正确,可以参考相关文档或示例代码确认正确的参数值。如果不确定可以尝试省略这个参数,让函数使用默认值。同时,也需要检查是否已经正确安装了必要的依赖库和模型文件。
row.record_status == 1 && $store.User.baseInfo.power == 2 && row.verify_status == 0 || row.verify_status == 3
这是一个条件表达式,包含了多个条件判断。根据给出的条件,我可以解释一下它的含义:
1. `row.record_status == 1`:判断 `row` 的 `record_status` 是否等于 1。
2. `$store.User.baseInfo.power == 2`:判断 `$store.User.baseInfo.power` 是否等于 2。
3. `row.verify_status == 0`:判断 `row` 的 `verify_status` 是否等于 0。
4. `row.verify_status == 3`:判断 `row` 的 `verify_status` 是否等于 3。
整个条件表达式使用了逻辑运算符来组合这些条件:
- `&&`:逻辑与运算符,表示同时满足前后两个条件。
- `||`:逻辑或运算符,表示满足前后两个条件中的任意一个即可。
因此,整个条件表达式的含义是:当 `row.record_status` 等于 1 并且 `$store.User.baseInfo.power` 等于 2,并且 `row.verify_status` 等于 0 或者 `row.verify_status` 等于 3 时,条件判断结果为真。
相关推荐
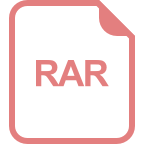
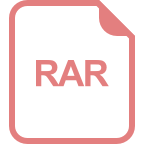












