np.array(df['close'])
时间: 2023-06-10 08:08:23 浏览: 143
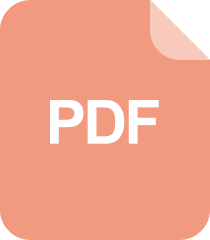
浅谈numpy中np.array()与np.asarray的区别以及.tolist
这段代码将DataFrame中'close'列的值转换为NumPy数组。假设你已经导入了NumPy和Pandas库,可以这样使用:
```python
import numpy as np
import pandas as pd
# 假设你的数据存储在DataFrame df中
close_arr = np.array(df['close'])
```
这将创建一个名为`close_arr`的NumPy数组,其中包含DataFrame中'close'列的所有值。你可以使用这个数组进行各种数值计算和分析。
阅读全文
相关推荐
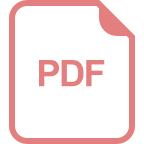
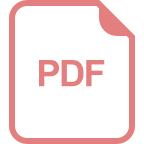








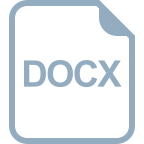
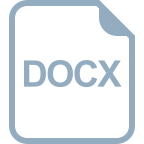
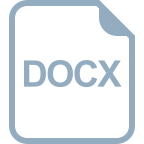
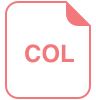



