fast rcnn和yolo的优缺点是什么
时间: 2023-06-02 10:08:21 浏览: 182
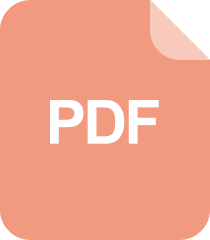
ssd yolo faster rcnn 对比
Fast R-CNN相比于YOLO的优点是,其定位准确度更高,同时能够生成更好的真实边界框(RoI);但其缺点是速度较慢,需要进行两个模型之间的交互,耗费时间和计算资源。而YOLO则是一种单级的目标检测框架,速度较快;但其缺点是准确度较低,特别是对于小目标的检测能力较弱。
阅读全文
相关推荐
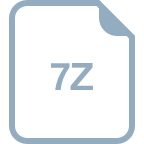
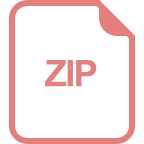

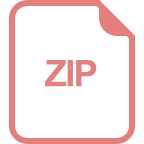
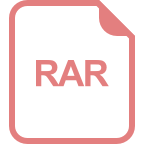
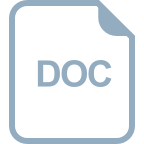
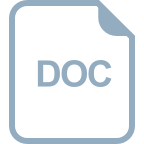
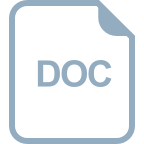
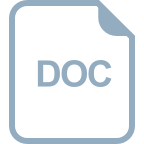
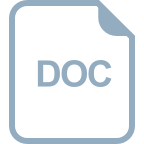
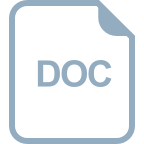
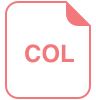
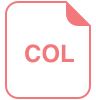
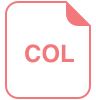
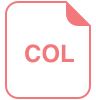
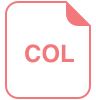
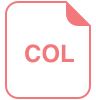
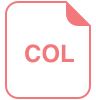
ssd yolo faster rcnn 对比