这行代码实现什么功能:contour_filter.distribution = ColorMapDistribution.Continuous
时间: 2023-06-08 13:07:46 浏览: 54
这行代码的功能是将 contour_filter 对象的 distribution 属性设置为 ColorMapDistribution 枚举类型下的 Continuous 成员,用于在连续的颜色映射中计算轮廓线的颜色值分布。
相关问题
def compute_shape_features(images): contour_areas = [] contour_perimeters = [] aspect_ratios = [] for img in images: contours, _ = cv2.findContours(img, cv2.RETR_EXTERNAL, cv2.CHAIN_APPROX_SIMPLE) contour = max(contours, key=cv2.contourArea) area = cv2.contourArea(contour) perimeter = cv2.arcLength(contour, True) x, y, w, h = cv2.boundingRect(contour) aspect_ratio = w / h if h != 0 else 0 contour_areas.append(area) contour_perimeters.append(perimeter) aspect_ratios.append(aspect_ratio) return np.array(contour_areas), np.array(contour_perimeters), np.array(aspect_ratios)的公牛
这段代码是用来计算一组图像的形状特征的。它通过使用OpenCV库的函数来找出每个图像的轮廓(contours),并计算轮廓的面积(contour_area)、周长(contour_perimeter)和宽高比(aspect_ratio)等特征。最后将这些特征存储在三个NumPy数组(contour_areas、contour_perimeters和aspect_ratios)中,并返回这些数组。这段代码的应用场景可能是对图像进行分类或者识别等任务,通过这些形状特征来区分不同的物体或者场景。
def chose_licence_plate(contours, Min_Area=2000): temp_contours = [] for contour in contours: if cv2.contourArea(contour) > Min_Area: temp_contours.append(contour) car_plate = [] for temp_contour in temp_contours: rect_tupple = cv2.minAreaRect(temp_contour) rect_width, rect_height = rect_tupple[1] if rect_width < rect_height: rect_width, rect_height = rect_height, rect_width aspect_ratio = rect_width / rect_height # 车牌正常情况下宽高比在2 - 5.5之间 if aspect_ratio > 2 and aspect_ratio < 5.5: car_plate.append(temp_contour) rect_vertices = cv2.boxPoints(rect_tupple) rect_vertices = np.int0(rect_vertices) return car_plate。 能详细解释这段代码吗
这段代码是一个函数,名为chose_licence_plate,它的作用是从一组轮廓中选择出符合条件的车牌轮廓。其中,参数contours是输入的轮廓列表,Min_Area是最小面积阈值,默认为2000。函数首先对输入的轮廓进行筛选,只保留面积大于Min_Area的轮廓,然后对这些轮廓进行进一步处理,计算其最小外接矩形的宽高比,如果宽比高小,则交换宽高比,最后将符合条件的车牌轮廓保存在car_plate列表中。
相关推荐
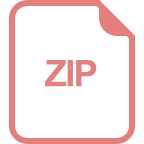












