Y=[] for ind in review_long_clean.index_content.unique(): y=[ word for word in review_long_clean.content_type[review_long_clean.index_content==ind].unique() ] Y.append(y) len(Y) X=[] for ind in review_long_clean.index_content.unique(): term=[ word for word in review_long_clean.word[review_long_clean.index_content==ind].values ] X.append(' '.join(term)) len(X) X Y
时间: 2024-04-06 18:32:38 浏览: 139
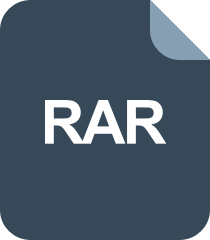
win_is_ind.rar_The Call
根据你提供的代码,我可以看出这是一个数据处理的过程,其中 X 和 Y 分别是特征和标签。其中 X 是一个列表,每个元素是一篇文本的词语拼接而成的字符串,Y 是一个列表,每个元素是一篇文本的标签列表。具体而言,代码中使用了 pandas 库进行数据处理,从 review_long_clean 数据集中根据 index_content 列进行了分组,然后按照 content_type 列和 word 列分别提取了标签和词语,并将它们组成了 X 和 Y 两个列表。最后,X 和 Y 的长度应该是相等的,分别对应了数据集中 unique 的 index_content 的数量。
阅读全文
相关推荐
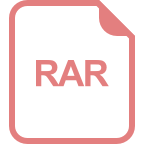
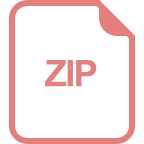














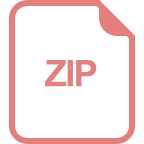
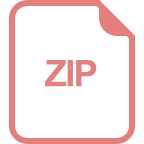