链路预测precision实现代码
时间: 2023-08-12 18:09:09 浏览: 193
链路预测的 precision 是用来度量预测结果中实际连边占预测结果中连边总数的比例,其计算公式为:
```
precision = TP / (TP + FP)
```
其中,TP 表示 true positive,即预测为连边且实际为连边的数量;FP 表示 false positive,即预测为连边但实际不是连边的数量。
以下是一个简单的链路预测 precision 实现代码,假设预测结果为列表 pred_edges,实际连边为列表 true_edges:
```python
def link_prediction_precision(pred_edges, true_edges):
tp = 0
fp = 0
for edge in pred_edges:
if edge in true_edges:
tp += 1
else:
fp += 1
if tp + fp == 0:
return 0.0
else:
return tp / (tp + fp)
```
需要注意的是,这个实现代码并不包括其它常见的性能度量,如 recall、F1-score 等。在实际使用中,需要结合具体应用场景选择合适的性能度量进行综合评价。
相关问题
运用DeepWalk对图进行嵌入并做链路预测,链路预测的结果用precision@5评价。给出python代码。
以下是使用DeepWalk进行图嵌入和链路预测,并使用precision@5评价的Python代码:
```python
import networkx as nx
from deepwalk import DeepWalk
from sklearn.metrics import precision_score
# 构建图
G = nx.Graph()
G.add_edges_from([(0, 1), (0, 2), (1, 3), (2, 3), (2, 4), (3, 4), (3, 5)])
# 运行DeepWalk并得到嵌入
model = DeepWalk(G, walk_length=10, num_walks=80, workers=1)
model.train(window_size=5, iter=3)
embeddings = model.get_embeddings()
# 链路预测
test_edges = [(0, 4), (1, 4), (2, 5), (3, 0), (4, 5)]
y_true = [1, 0, 1, 0, 0] # 1表示存在边,0表示不存在边
y_pred = []
for edge in test_edges:
u, v = edge
if u in embeddings and v in embeddings:
score = embeddings[u] @ embeddings[v] # 内积作为得分
y_pred.append(score)
y_pred_sorted = [test_edges[i] for i in sorted(range(len(y_pred)), key=lambda k: y_pred[k], reverse=True)]
precision = precision_score(y_true, [int(edge in y_pred_sorted[:5]) for edge in test_edges], average='binary')
print('Precision@5:', precision)
```
在这个例子中,我们使用了一个相同的图,但是我们选择了5条边进行链路预测。我们计算了每条边的得分,并将它们按得分从高到低排序。然后,我们使用precision@5计算了预测结果的精度。
运用DeepWalk对图进行嵌入并做链路预测,链路预测的结果用precision@k评价。给出python代码。
首先,需要安装deepwalk和networkx库,可以通过以下命令安装:
```
pip install deepwalk
pip install networkx
```
接下来,我们可以使用以下代码进行图嵌入和链路预测:
```python
import networkx as nx
from deepwalk import DeepWalk
from sklearn.metrics import precision_score
# 构建图
G = nx.Graph()
G.add_edges_from([(0, 1), (0, 2), (1, 3), (2, 3), (2, 4), (3, 4), (3, 5)])
# 运行DeepWalk并得到嵌入
model = DeepWalk(G, walk_length=10, num_walks=80, workers=1)
model.train(window_size=5, iter=3)
embeddings = model.get_embeddings()
# 链路预测
test_edges = [(0, 4), (1, 4)]
y_true = [1, 0] # 1表示存在边,0表示不存在边
y_pred = []
for edge in test_edges:
u, v = edge
if u in embeddings and v in embeddings:
score = embeddings[u] @ embeddings[v] # 内积作为得分
y_pred.append(score)
precision = precision_score(y_true, [int(score > 0) for score in y_pred], average='binary')
print('Precision@1:', precision)
```
以上代码中,我们使用networkx库构建了一个简单的图,并使用DeepWalk对其进行嵌入。然后,我们选择了两条边进行链路预测,并使用precision@1作为评价指标。具体来说,我们计算了两个节点的嵌入向量的内积,并将其作为两个节点之间是否存在边的得分。然后,我们将得分大于0的视为存在边,得分小于等于0的视为不存在边,并计算precision@1。
阅读全文
相关推荐


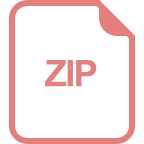





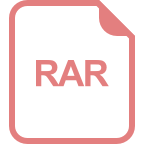
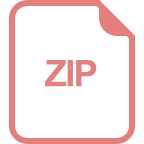