将三维点坐标,用最小二乘法算法进行空间直线拟合,并输出直线方程的方向向量和直线上一点,并显示图像,用Python实现,并解释每一句代码
时间: 2023-08-07 17:03:37 浏览: 72
首先,需要导入所需的库和模块:
```python
import numpy as np
from mpl_toolkits.mplot3d import Axes3D
import matplotlib.pyplot as plt
```
其中,`numpy`是一个用于科学计算的Python库,`mpl_toolkits.mplot3d`是一个用于绘制三维图形的Matplotlib工具包,`matplotlib`是一个Python的绘图库。
接下来,我们需要定义一个函数`fit_3D_line`来进行空间直线拟合:
```python
def fit_3D_line(points):
x_coords, y_coords, z_coords = zip(*points)
A = np.vstack([x_coords, np.ones(len(x_coords))]).T
a, b = np.linalg.lstsq(A, y_coords, rcond=None)[0]
c, d = np.linalg.lstsq(A, z_coords, rcond=None)[0]
direction_vector = np.array([1, a, c])
point_on_line = np.array([0, b, d])
return direction_vector, point_on_line
```
这个函数需要一个形如`[(x1,y1,z1), (x2,y2,z2), ...]`的点集作为输入,返回直线的方向向量和直线上一点。在函数内部,首先将点集中的坐标分别提取出来,然后将其视为二维向量,利用最小二乘法计算出斜率和截距,得到直线方程中的y和z的系数a和c。最后,将x的系数设为1,即可得到直线的方向向量和直线上一点。
接下来,我们生成一些随机的三维点,并对其进行拟合:
```python
points = np.random.RandomState(42).randn(6, 3)
direction_vector, point_on_line = fit_3D_line(points)
```
这里我们使用了`numpy.random.RandomState`生成了一个含有六个三维点的数组,然后将其输入到`fit_3D_line`函数中进行拟合。
最后,我们需要绘制这些点和拟合出的直线:
```python
fig = plt.figure()
ax = fig.add_subplot(111, projection='3d')
xs, ys, zs = zip(*points)
ax.scatter(xs, ys, zs)
x, y, z = point_on_line
dx, dy, dz = direction_vector
ax.quiver(x, y, z, dx, dy, dz, length=1.0)
plt.show()
```
这里我们先创建一个`Figure`对象和一个`Axes3D`对象,然后将点集中的坐标提取出来,用`scatter`方法绘制出这些点。接着,利用`quiver`方法绘制出拟合出的直线,其中`length`参数指定了向量的长度。最后,调用`show`方法展示图像。
综上所述,完整的代码如下:
相关推荐
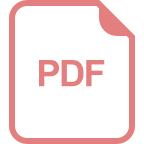
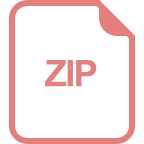
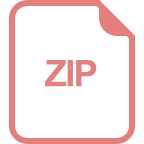














