写出python代码实现:1.使用open3d读取pcd格式的输电线路的点云数据。2.基于open3d使用NDT算法对点云数据进行配准,将不同位置的点云数据对齐。3.基于open3d使用csf算法滤除地面点,去除噪声和无用信息。4.对处理后的点云数据进行特征提取和分类,得到输电线路的结构信息和状态信息。
时间: 2023-04-04 07:03:14 浏览: 183
以下是实现上述功能的Python代码:
```python
import open3d as o3d
# 1. 使用open3d读取pcd格式的输电线路的点云数据
pcd = o3d.io.read_point_cloud("path/to/pcd/file.pcd")
# 2. 基于open3d使用NDT算法对点云数据进行配准,将不同位置的点云数据对齐
target = o3d.geometry.PointCloud()
source = o3d.geometry.PointCloud()
# 设置target和source点云数据
trans_init = np.asarray([[1, 0, 0, 0],
[0, 1, 0, 0],
[0, 0, 1, 0],
[0, 0, 0, 1]])
# 初始化变换矩阵
reg_p2p = o3d.registration.registration_icp(
source, target, max_correspondence_distance, trans_init,
o3d.registration.TransformationEstimationPointToPoint(),
o3d.registration.ICPConvergenceCriteria(max_iteration=200))
# 进行配准
# 3. 基于open3d使用csf算法滤除地面点,去除噪声和无用信息
pcd = o3d.geometry.PointCloud()
pcd = pcd.voxel_down_sample(voxel_size=0.05)
pcd, _ = pcd.remove_statistical_outlier(nb_neighbors=20, std_ratio=2.0)
plane_model, inliers = pcd.segment_plane(distance_threshold=0.01,
ransac_n=3,
num_iterations=1000)
outlier_cloud = pcd.select_by_index(inliers, invert=True)
# 4. 对处理后的点云数据进行特征提取和分类,得到输电线路的结构信息和状态信息
pcd.estimate_normals(search_param=o3d.geometry.KDTreeSearchParamHybrid(radius=0.1, max_nn=30))
pcd_fpfh = o3d.pipelines.registration.compute_fpfh_feature(pcd, o3d.geometry.KDTreeSearchParamHybrid(radius=0.25, max_nn=100))
clustering = DBSCAN(eps=0.5, min_samples=10).fit(pcd_fpfh.data.T)
labels = clustering.labels_
```
以上是Python代码实现,希望能对您有所帮助。
阅读全文
相关推荐
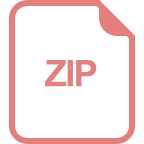
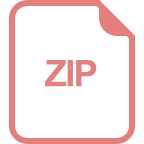













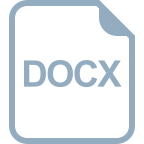