projected gradient descent
时间: 2023-04-25 22:03:24 浏览: 232
投影梯度下降法(projected gradient descent)是一种优化算法,用于解决约束优化问题。它通过在每次迭代中将梯度投影到可行域内来保证解的可行性。该算法在机器学习和优化领域广泛应用。
相关问题
这是什么意思We optimize the loss (3) or (6) by projected gradient descent with line search (subject to the observation above). The projection consists of imposing Pk Yk = 0, which we enforce by centering ∇Y before taking a step. This eliminates the degeneracy of the Loss in (3) and (6) w.r.t constant shift in Y. To further improve the good trade-off between time per iteration and number of iterations, we found that a heavy-ball method with parameter α is effective. At each iteration computing the gradient is O((S + s3)n) where S is the number of nonzero entries of L.
这段话是在描述一种优化算法,使用投影梯度下降法来优化损失函数(3)或(6),并且在投影时强制执行Pk Yk = 0的约束条件。在采取步骤之前,通过将∇Y居中来消除(3)和(6)中的常数移位的退化性。为了进一步改善每次迭代的时间和迭代次数之间的良好平衡,发现带参数α的重球方法是有效的。在每次迭代中,计算梯度的复杂度为O((S + s3)n),其中S是L的非零条目数。
代码 arr=projected_gradient_descent(get_classifier(),x,eps=0.3,eps_iter=0.01,nb_iter=50,norm=2, loss_fn=None, clip_min=0, clip_max=1, y=None, targeted=False, rand_init=None, rand_minmax=None, sanity_checks=False)
该代码是一个使用投影梯度下降(projected gradient descent)算法进行对抗样本攻击的函数。具体参数解释如下:
- get_classifier(): 获取分类器函数,即对抗样本攻击的目标模型。
- x: 原始图像(或批量图像)。
- eps: 对抗扰动的最大范数,即攻击强度。
- eps_iter: 每次迭代中允许的最大扰动,可控制对抗样本的精度和速度。
- nb_iter: 迭代次数。
- norm: 扰动范数的类型,取值为0、1或2。
- loss_fn: 损失函数,用于评估对抗样本的质量。默认为None,表示使用原始模型的损失函数。
- clip_min: 图像像素值的下界。
- clip_max: 图像像素值的上界。
- y: 原始图像的标签。如果是有监督的攻击,则需要指定。
- targeted: 是否进行有目标攻击。
- rand_init: 是否在原始图像上添加随机扰动作为初始扰动。
- rand_minmax: 随机扰动的范围,以像素值为单位。
- sanity_checks: 是否进行一些额外的检查以确保输入参数的合法性。
函数返回值为攻击后的图像(或批量图像)。
相关推荐
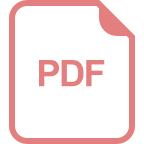
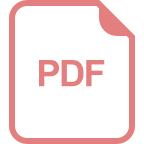
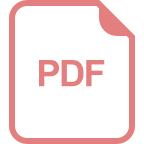












